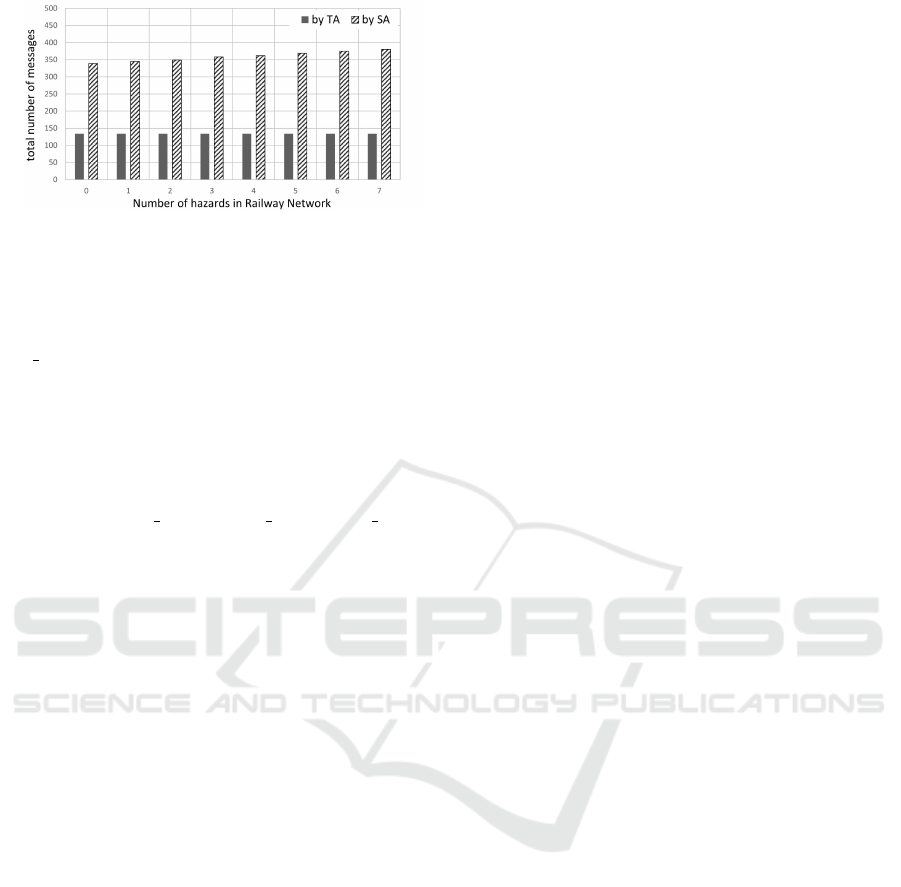
Figure 4: Summary of message passing.
7.2 Communication Overhead
Each TA sends two types of messages - ARR and
T H U T IL. During any type of hazard TA need not to
send any extra message. So according to figure 4 the
number of messages sent by all Train Agents remains
same irrespective of number of hazards occured. Each
Segment Agent sends 7 types of messages. Number
of messages REQ, DEPT , WAIT and GRANT re-
mains unchanged irespective of number of hazards.
But number of OT GRANT , OT REQ, LINK PROB
messages changes depending on the number and type
of hazards. As shown in Figure 4 number of messages
sent by all Segment Agents is not increased a lot with
the increase of number of hazards.
8 CONCLUSION
With the increase in number of trains and tracks in
Indian Railways, the on demand scheduling task be-
comes time consuming. As per the case study it is evi-
dent that Multi-agent based approach described in this
paper has promising aspects for taking dynamic deci-
sion more accurately. As Railway Network graph is
not too much complex as complete graph, no prepro-
cessing is required like other DCOP algorithms like
(Petcu and Faltings, 2005) and (Modi et al., 2005). It
is a major advantage to have additional time for multi-
agent communication. In this paper robustness is im-
plemented in Railway Network. To support this re-
search the microscopic view of ‘how to change track
and optimize the delay at junction points’ should be
taken under consideration in the near future.
ACKNOWLEDGEMENT
This research work is funded by Visvesvaraya PhD
scheme of DeitY (Department of Electronics & Infor-
mation Technology), Govt. of India.
REFERENCES
Cacchiani, V. and Toth, P. (2012). Nominal and robust train
timetabling problems. European Journal of Opera-
tional Research, 219(3):727–737.
Chung, J.-W., Oh, S.-M., and Choi, I.-C. (2009). A hybrid
genetic algorithm for train sequencing in the korean
railway. Omega, 37(3):555–565.
Cicerone, S., DAngelo, G., Di Stefano, G., Frigioni, D., and
Navarra, A. (2009). Recoverable robust timetabling
for single delay: Complexity and polynomial algo-
rithms for special cases. Journal of Combinatorial
Optimization, 18(3):229–257.
Dalapati, P., Singh, A., Dutta, A., and Bhattacharya, S.
(2014). Multi agent based railway scheduling and op-
timization. pages 1–6.
Farinelli, A., Rogers, A., Petcu, A., and Jennings, N. R.
(2008). Decentralised coordination of low-power em-
bedded devices using the max-sum algorithm. pages
639–646.
Fischetti, M. and Monaci, M. (2009). Lecture notes in
computer science. Robust and Online Large-Scale
Optimization,R.K. Ahuja, R. Moehring, C. Zaroliagis
(Eds.), 5868:61–84.
Kiekintveld, C., Yin, Z., Kumar, A., and Tambe, M. (2010).
Asynchronous algorithms for approximate distributed
constraint optimization with quality bounds. pages
133–140.
Kuo, A., Miller-Hooks, E., and Mahmassani, H. S. (2010).
Freight train scheduling with elastic demand. Trans-
portation Research Part E: Logistics and Transporta-
tion Review, 46(6):1057–1070.
Liebchen, C., Schachtebeck, M., Sch
¨
obel, A., Stiller, S.,
and Prigge, A. (2010). Computing delay resistant rail-
way timetables. Computers & Operations Research,
37(5):857–868.
Modi, P. J., Shen, W.-M., Tambe, M., and Yokoo, M.
(2005). Adopt: Asynchronous distributed constraint
optimization with quality guarantees. Artificial Intel-
ligence, 161(1):149–180.
Petcu, A. and Faltings, B. (2005). A scalable method for
multiagent constraint optimization. IJCAI, pages 266–
271.
Sahana, S. K., Jain, A., and Mahanti, P. K. (2014). Ant
colony optimization for train scheduling: an analysis.
International Journal of Intelligent Systems and Ap-
plications, 6(2):29.
Salido, M. A., Abril, M., Barber, F., Ingolotti, L., Tor-
mos, P., and Lova, A. (2007). Domain-dependent dis-
tributed models for railway scheduling. Knowledge-
Based Systems, 20(2):186–194.
Takeuchi, Y., Tomii, N., and Hirai, C. (2007). Evaluation
method of robustness for train schedules. Quarterly
Report of RTRI, 48(4):197–201.
Tormos, P., Lova, A., Barber, F., Ingolotti, L., Abril, M.,
and Salido, M. (2008). A genetic algorithm for rail-
way scheduling problems. Studies in Computa- tional
Intelligence (SCI), page 255276.
ICAART 2017 - 9th International Conference on Agents and Artificial Intelligence
144