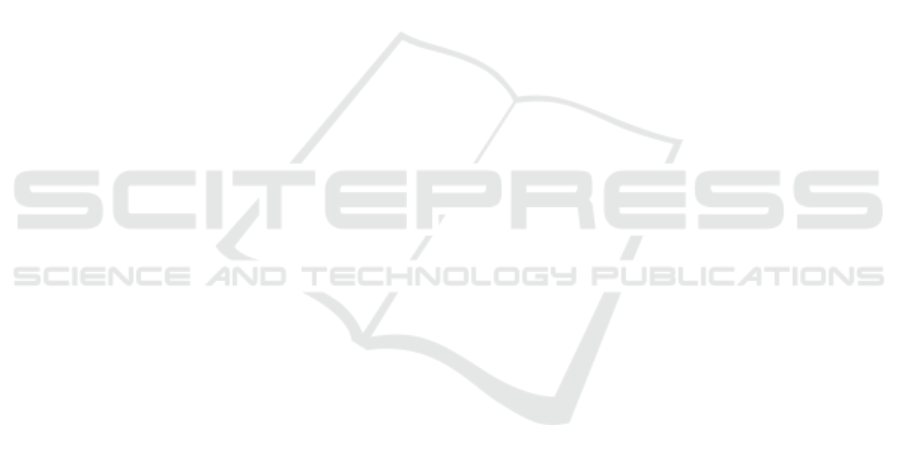
this property of ConvNets we followed the procedure
in (4) to generate adversarial examples in specific
radii. Then, we computed the Mantel score between
x
perturbed
input
and x
perturbed
out put
for each ConvNet separately.
Figure 4 shows the results.
We observe that topology of point does not lin-
early change even when they are very close to x. This
is due to the fact that the Mantel score for all of Con-
vNets is −0.1 < ρ < 0.1. As we mentioned earlier,
a simple linear transformation such as affine transfor-
mation changes the topology of points. If we think
of ConvNets as fully-connected networks with shared
weights, we realize that every neuron in this network
applies the affine transformation f (XW + b) on its in-
puts where f (.) is an activation function. This affine
transformation changes the topology of points. Con-
sidering a deep network with several convolution lay-
ers, the input passes through multiple affine transfor-
mations which greatly changes the topology of inputs.
As the result, points located at distance ε from the
original sample will not have the same topology at
the output of a ConvNet.
2.4 Lipschitz
The method discussed in Section 2.3 takes into ac-
count all pair-wise distances between samples in or-
der to compare topology of points before and after
applying Φ
L
(X). Lipschitz analysis is an alternative
method to study non-linearity of a function. Specif-
ically, given X
1
, X
2
∈ R
D
input
and function Φ
L
(X) :
R
D
input
→ R
D
out put
, Lipschitz analysis finds a constant
L called Lipschitz constant such that:
kΦ
L
(X
1
) − Φ
L
(X
2
)k ≤ LkX
1
− X
2
k
f or all X
1
, X
2
∈ R
D
input
.
(8)
This definition studies the global non-linearity of a
function. Szegedy et.al. (Szegedy et al., 2014b)
showed how to compute L for a ConvNet with convo-
lution, pooling and activation layers. Notwithstand-
ing, Lipschitz constant L found by applying (8) on
whole domain of a ConvNet does not accurately tell
us how output of the ConvNet changes locally. This
problem is shown in Figure 5. We see that the pur-
ple function is more non-linear than the yellow func-
tion. This is due to the fact that its non-linearity is
less when |x| > 5. Notwithstanding, degree of non-
linearity of both function are similar when −5 < x <
5. The Lipschitz analysis in (8) does not take into
account local non-linearity of a function. Instead, it
find L which equals to greatest gradient magnitude in
whole domain of the function.
Our aim is to study behaviour of function on ad-
versarial examples. Therefore, we must compute Lip-
schitz constant L locally. To be more specific, denot-
ing an adversarial sample with x
a
= x + ν and a clean
sample with x we find L
x
such that:
kg(Φ
L
(x
a
)) − g(Φ
L
(x))k ≤ L
x
kh(x
a
) − h(x)k
f or all ν ∈ [−ε, ε]
D
input
.
(9)
where g(.) and h(.) are two function to normalize
their input. From topology point of view, the above
equation studies how adversarial examples are trans-
formed by a ConvNet with respect to the original sam-
ple. If L
x
< 1 for all adversarial samples, this means
that Φ
L
(X) attracts the adversarial examples toward
the clean sample (They become closer to the clean
sample after being transformed to D
out put
dimensional
space by the ConvNet). However, the distance be-
tween adversarial examples and the clean example re-
mains unchanged when L
x
= 1 for all adversarial sam-
ples. Finally, Φ
L
(X) repels the adversarial examples
from the clean sample when L
x
> 1.
A ConvNet will be more tolerant against adver-
sarial samples when L
x
< 1. This is due to the fact
that when adversarial samples get closer to the clean
sample, it is more likely that they have classification
scores close to the clean sample. To empirically study
the Lipschitz constant, we generated the samples us-
ing (4) and computed kΦ
L
(x + ν) − Φ
L
(x)k as well
as kνk. It is worth mentioning that the clean samples
as well as ν
r
i
in (4) are the same for all the ConvNets
trained on the same dataset. In addition, g() and h()
are two separate min-max normalizers in which their
parameters are obtained by feeding thousands of sam-
ples to each ConvNet and collecting the minimum and
maximum value in the input and output of the Con-
vNet. Finally, each sample has a unique seed for the
uniform noise function. This means that if we run the
algorithm many times on different ConvNets for the
sample i, the same adversarial examples will be gener-
ated in all the cases. By this way, we can compare the
results from the ConvNets trained on the same dataset.
Figure 6 shows the relation between these two fac-
tors. In addition, the black and blue lines are obtained
by fitting a first order (linear regression) and second
order polynomial on data. Color of each point corre-
sponds to the radius to which the adversarial sample
is located. The colder color shows a smaller radius.
Even though (Szegedy et al., 2014b) mentioned
that the global Lipschitz constant on AlexNet is
greater than 1, our empirical analysis revealed that all
of the ConvNets in our study are in general locally
contraction. In other words, the Lipschitz constant
on is less than 1 in most of the cases meaning that
adversarial examples become closer to the original
sample despite the fact that their topology changes by
Φ
L
(x). This suggests that that although ConvNet are
Explaining Adversarial Examples by Local Properties of Convolutional Neural Networks
231