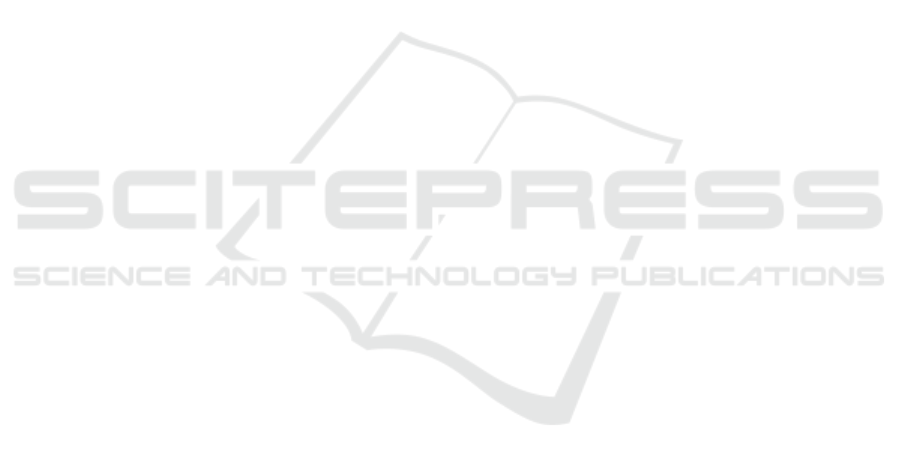
ings of the 23rd international conference on Machine
learning, pages 233–240.
De Cabrol, A., Garcia, T., Bonnin, P., and Chetto, M.
(2008). A concept of dynamically reconfigurable real-
time vision system for autonomous mobile robotics.
International Journal of Automation and Computing,
5(2):174–184.
Doll
´
ar, P., Belongie, S., and Perona, P. (2010). The fastest
pedestrian detector in the west. In Proc. BMVC, pages
68.1–11.
Gupta, P., Loparo, K., Mackall, D., Schumann, J., and
Soares, F. (2004). Verification and validation method-
ology of real-time adaptive neural networks for
aerospace applications. In International Conference
on Computational Intelligence for Modeling, Control,
and Automation.
Ingibergsson, J. T. M., Schultz, U. P., and Kuhrmann, M.
(2015). On the use of safety certification practices in
autonomous field robot software development: A sys-
tematic mapping study. In Product-Focused Software
Process Improvement, pages 335–352. Springer.
Kurd, Z. and Kelly, T. (2003). Establishing safety criteria
for artificial neural networks. In Knowledge-Based In-
telligent Information and Engineering Systems, pages
163–169. Springer.
Kurd, Z., Kelly, T., and Austin, J. (2003). Safety criteria
and safety lifecycle for artificial neural networks. In
Proc. of Eunite, volume 2003.
Lucas, B. D., Kanade, T., et al. (1981). An iterative image
registration technique with an application to stereo vi-
sion. In IJCAI, volume 81, pages 674–679.
Mekki-Mokhtar, A., Blanquart, J.-P., Guiochet, J., Powell,
D., and Roy, M. (2012). Safety trigger conditions
for critical autonomous systems. In 18th Pacific Rim
International Symposium on Dependable Computing,
pages 61–69. IEEE.
Mitka, E., Gasteratos, A., Kyriakoulis, N., and Mouroutsos,
S. G. (2012). Safety certification requirements for do-
mestic robots. Safety science, 50(9):1888–1897.
Murphy, R. R. and Hershberger, D. (1999). Handling sens-
ing failures in autonomous mobile robots. The In-
ternational Journal of Robotics Research, 18(4):382–
400.
Nguyen, A., Yosinski, J., and Clune, J. (2015). Deep neu-
ral networks are easily fooled: High confidence pre-
dictions for unrecognizable images. In Conference
on Computer Vision and Pattern Recognition (CVPR),
pages 427–436. IEEE.
Saito, T. and Rehmsmeier, M. (2015). The precision-recall
plot is more informative than the roc plot when eval-
uating binary classifiers on imbalanced datasets. In
PLoS ONE, pages 1–21.
Santosuosso, A., Boscarato, C., Caroleo, F., Labruto, R.,
and Leroux, C. (2012). Robots, market and civil li-
ability: A european perspective. In RO-MAN, pages
1051–1058. IEEE.
Schumann, J., Gupta, P., and Liu, Y. (2010). Application of
neural networks in high assurance systems: A survey.
In Applications of Neural Networks in High Assurance
Systems, pages 1–19. Springer.
Szegedy, C., Zaremba, W., Sutskever, I., Bruna, J., Er-
han, D., Goodfellow, I., and Fergus, R. (2013). In-
triguing properties of neural networks. arXiv preprint
arXiv:1312.6199.
TC 127 (2015). Earth-moving machinery – autonomous
machine system safety. International Standard
ISO 17757-2015, International Organization for Stan-
dardization.
TC 184 (2014). Robots and robotic devices - Safety require-
ments for personal care robots. International Standard
ISO 13482:2014, International Organization for Stan-
dardization.
TC 23 (2010). Tractors and machinery for agriculture and
forestry – safety-related parts of control systems. In-
ternational Standard ISO 25119-2010, International
Organization for Standardization.
TC 23 (2014). Agricultural machinery and tractors – Safety
of highly automated machinery. International Stan-
dard ISO/DIS 18497, International Organization for
Standardization.
TC 23 (2015). Standards. International standard, Interna-
tional Organization for Standardization.
TC 44 (2012). Safety of machinery – electro-sensitive pro-
tective equipment. International Standard IEC 61496-
2012, International Electronical Commission.
Torralba, A. and Efros, A. A. (2011). Unbiased look
at dataset bias. In Conference on Computer Vision
and Pattern Recognition (CVPR), pages 1521–1528.
IEEE.
Wang, R. and Bhanu, B. (2005). Learning models for pre-
dicting recognition performance. In Tenth IEEE In-
ternational Conference on Computer Vision (ICCV),
volume 2, pages 1613–1618. IEEE.
Yang, L. and Noguchi, N. (2012). Human detection for
a robot tractor using omni-directional stereo vision.
Computers and Electronics in Agriculture, 89:116–
125.
Zendel, O., Murschitz, M., Humenberger, M., and Herzner,
W. (2015). Cv-hazop: Introducing test data valida-
tion for computer vision. In Proceedings of the IEEE
International Conference on Computer Vision, pages
2066–2074.
Zenke, D., Listner, D. J., and Author, A. (2016). Meeting
on safety in sensor systems with employees from T
¨
UV
NORD.
Zhang, P., Wang, J., Farhadi, A., Hebert, M., and Parikh, D.
(2014). Predicting failures of vision systems. In Pro-
ceedings of the IEEE Conference on Computer Vision
and Pattern Recognition, pages 3566–3573.
VISAPP 2017 - International Conference on Computer Vision Theory and Applications
444