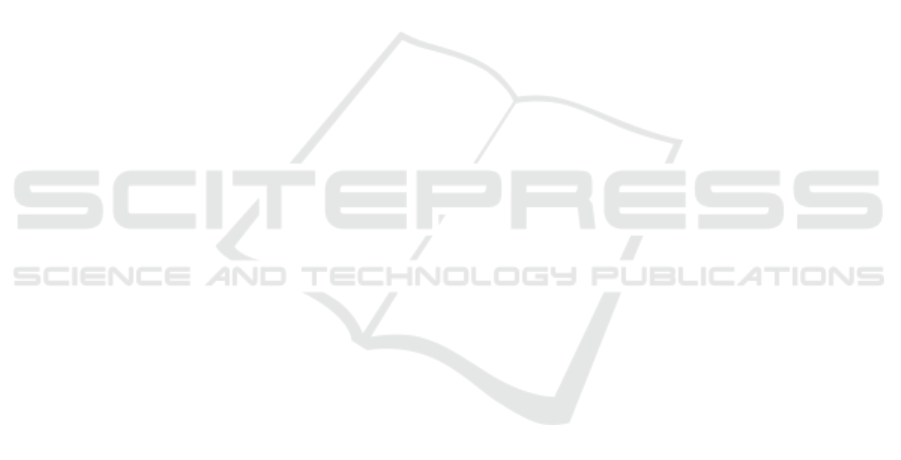
6 CONCLUSIONS
In the paper, we have shown that the coherent move-
ment extracted from dense Optical Flow method by
considering the facial movement hypothesis achieves
state-of-the-art performance on both facial full-
expression and micro-expression databases. The
magnitude and direction constraints are estimated in
order to reduce the noise induced by lighting changes
and small head motions over time. The proposed ap-
proach adapts well on both full-expressions (CK+)
and micro-expressions (CASME2). The only adjust-
ment concerning the magnitude intervals is actually
related to the nature of expression. The other param-
eters common to both experiences have been selected
empirically and deserve specific attention in future
experiments.
Our current approach is used only in near-frontal-
view recordings where the presence of occlusions,
fast head motion and lightning variation is practi-
cally zero. The next step consist in adapting our
method to the domain of spontaneous facial expres-
sion recognition. To address this situation, a normal-
ization method will be necessarily used. However, it
must be kept in mind that any change made in the
facial picture has important side-effects on the Op-
tical Flow. Despite the wealth of research already
conducted, no method is capable of dealing with all
issues at a time. We believe that the normalization
approaches based on facial components or shape are
not adapted to Optical Flow as facial deformation will
impact Optical Flow computation by inducing motion
distortion. So rather than considering the normaliza-
tion in the field of facial components, efforts should
instead be focused on the Optical Flow domain.
ACKNOWLEDGEMENTS
This research has been partially supported by the FUI
project MAGNUM 2.
REFERENCES
Bailer, C., Taetz, B., and Stricker, D. (2015). Flow fields:
Dense correspondence fields for highly accurate large
displacement optical flow estimation. In ICCV.
Chang, C.-C. and Lin, C.-J. (2011). Libsvm: a library for
support vector machines. ACM TIST.
Chen, Q. and Koltun, V. (2016). Full flow: Optical flow
estimation by global optimization over regular grids.
CVPR.
Farneb
¨
ack, G. (2003). Two-frame motion estimation based
on polynomial expansion. In SCIA. Springer.
Fortun, D., Bouthemy, P., and Kervrann, C. (2015). Optical
flow modeling and computation: a survey. Computer
Vision and Image Understanding.
Han, S., Meng, Z., Liu, P., and Tong, Y. (2014). Facial grid
transformation: A novel face registration approach for
improving facial action unit recognition. In ICIP.
Huang, X., Wang, S., Liu, X., Zhao, G., Feng, X.,
and Pietikainen, M. (2016a). Spontaneous fa-
cial micro-expression recognition using discrimina-
tive spatiotemporal local binary pattern with an im-
proved integral projection. CVPR.
Huang, X., Zhao, G., Hong, X., Zheng, W., and Pietik
¨
ainen,
M. (2016b). Spontaneous facial micro-expression
analysis using spatiotemporal completed local quan-
tized patterns. Neurocomputing.
Jiang, B., Martinez, B., Valstar, M. F., and Pantic, M.
(2014). Decision level fusion of domain specific re-
gions for facial action recognition. In ICPR.
Kazemi, V. and Sullivan, J. (2014). One millisecond face
alignment with an ensemble of regression trees. In
CVPR.
Lee, C.-S. and Chellappa, R. (2014). Sparse localized fa-
cial motion dictionary learning for facial expression
recognition. In ICASSP.
Li, X., Pfister, T., Huang, X., Zhao, G., and Pietik
¨
ainen,
M. (2013). A spontaneous micro-expression database:
Inducement, collection and baseline. In FG.
Liao, C.-T., Chuang, H.-J., Duan, C.-H., and Lai, S.-H.
(2013). Learning spatial weighting for facial expres-
sion analysis via constrained quadratic programming.
Pattern Recognition.
Liu, Y.-J., Zhang, J.-K., Yan, W.-J., Wang, S.-J., Zhao, G.,
and Fu, X. (2015). A main directional mean optical
flow feature for spontaneous micro-expression recog-
nition. Affective Computing.
Lucey, P., Cohn, J. F., Kanade, T., Saragih, J., Ambadar, Z.,
and Matthews, I. (2010). The extended cohn-kanade
dataset (ck+): A complete dataset for action unit and
emotion-specified expression. In CVPR Workshops.
P
´
eteri, R. and Chetverikov, D. (2005). Dynamic texture
recognition using normal flow and texture regularity.
In IbPRIA.
Revaud, J., Weinzaepfel, P., Harchaoui, Z., and Schmid, C.
(2015). Epicflow: Edge-preserving interpolation of
correspondences for optical flow. In CVPR.
Su, M.-C., Hsieh, Y., and Huang, D.-Y. (2007). A simple
approach to facial expression recognition. In WSEAS.
Wang, S.-J., Yan, W.-J., Zhao, G., Fu, X., and Zhou, C.-G.
(2014a). Micro-expression recognition using robust
principal component analysis and local spatiotempo-
ral directional features. In ECCV Workshop.
Wang, Y., See, J., Phan, R. C.-W., and Oh, Y.-H. (2014b).
Lbp with six intersection points: Reducing redundant
information in lbp-top for micro-expression recogni-
tion. In ACCV.
Yan, W.-J., Li, X., Wang, S.-J., Zhao, G., Liu, Y.-J., Chen,
Y.-H., and Fu, X. (2014). Casme ii: An improved
spontaneous micro-expression database and the base-
line evaluation. PloS one.
VISAPP 2017 - International Conference on Computer Vision Theory and Applications
242