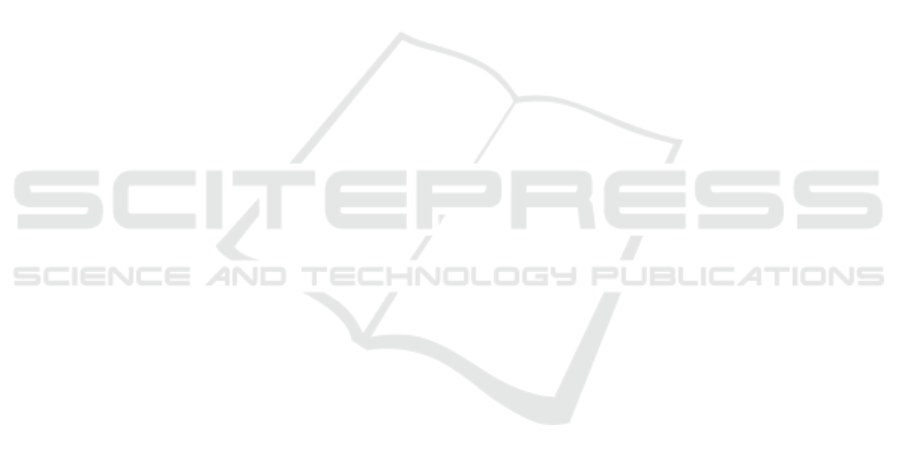
computation bottlenecks.
We demonstrated the performance of our method
by an evaluation on three multi-camera video se-
quences, confirming the accuracy improvement over
the classical triangulation method when there are fre-
quent and severe occlusions. Performance compar-
ison with state-of-the-art trackers on the widely used
PETS2009 video sequence shows that our tracker out-
performs other methods. Furthermore, the analysis of
the local estimations as well as the fused result reveals
that huge positional errors in local estimation often
correspond to occlusion and that our fusion method is
able to minimize these errors.
As future work, we will explore the possibility of
integrating other view specific attributes, which can
potentially correlate to the accuracy of the local po-
sition estimations, into the proposed fusion method.
These attributes include calibration accuracy at the
target’s position, distance between the target and the
camera, and so on. We will also conduct experiments
to show the genericity of our fusion method by de-
ploying different single view tracking algorithms on
different camera views and observing the accuracy
improvement in the fused results.
ACKNOWLEDGEMENTS
The work was financially supported by FWO through
the BOF–GOA project the project 01GA2111W “Dis-
tributed Smart Camera Systems”.
REFERENCES
Andriyenko, A. and Schindler, K. (2010). Globally optimal
multi-target tracking on a hexagonal lattice. In Pro-
ceedings of the 11th European Conference on Com-
puter Vision: Part I, ECCV’10, pages 466–479.
Andriyenko, A. and Schindler, K. (2011). Multi-target
tracking by continuous energy minimization. In Com-
puter Vision and Pattern Recognition (CVPR), 2011
IEEE Conference on, pages 1265–1272.
Berclaz, J., Fleuret, F., Turetken, E., and Fua, P. (2011).
Multiple object tracking using k-shortest paths opti-
mization. IEEE Trans. on Pattern Analysis and Ma-
chine Intelligence, 33(9):1806–1819.
Bernardin, K. and Stiefelhagen, R. (2008). Evaluating mul-
tiple object tracking performance: The clear mot met-
rics. J. Image Video Process., 2008:1–10.
Bo Bo, N., Deboeverie, F., Eldib, M., Guan, J., Xie, X.,
Nio, J., Van Haerenborgh, D., Slembrouck, M., Van de
Velde, S., Steendam, H., Veelaert, P., Kleihorst, R.,
Aghajan, H., and Philips, W. (2014a). Human mo-
bility monitoring in very low resolution visual sensor
network. Sensors, 14(11):20800–20824.
Bo Bo, N., Deboeverie, F., Veelaert, P., and Philips, W.
(2015). Real-time multi-people tracking by greedy
likelihood maximization. In Proceedings of the 9th
International Conference on Distributed Smart Cam-
eras, ICDSC ’15, pages 32–37, New York, NY, USA.
ACM. [doi:10.1145/2789116.2789125].
Bo Bo, N., Deboeverie, F., Veelaert, P., and Philips, W.
(2016). Multiple people tracking in smart camera net-
works by greedy joint-likelihood maximization. In
Proceedings of the 11th Joint Conference on Com-
puter Vision, Imaging and Computer Graphics Theory
and Applications, pages 602–609.
Bo Bo, N., Gruenwedel, S., Van Hese, P., Ni
˜
no Casta
˜
neda,
J., Van Haerenborgh, D., Van Cauwelaert, D., Vee-
laert, P., and Philips, W. (2012). Phd forum:
Illumination-robust foreground detection for multi-
camera occupancy mapping. In Proceedings of the
Sixth International Conference on Distributed Smart
Cameras (ICDSC).
Bo Bo, N., Gr
¨
unwedel, S., Van Hese, P., Guan, J., Nio-
Castaeda, J., Van Haerenborgh, D., Van Cauwelaert,
D., Veelaert, P., and Philips, W. (2014b). Illumination-
robust people tracking using a smart camera network.
In PROCEEDINGS OF SPIE, Intelligent Robots and
Computer Vision XXXI: Algorithms and Techniques,
volume 9025, pages 90250G–90250G–10.
Bredereck, M., Jiang, X., Korner, M., and Denzler, J.
(2012). Data association for multi-object tracking-by-
detection in multi-camera networks. In Distributed
Smart Cameras (ICDSC), 2012 Sixth International
Conference on, pages 1–6.
Du, W. and Piater, J. (2006). Data fusion by belief propaga-
tion for multi-camera tracking. In 2006 9th Interna-
tional Conference on Information Fusion, pages 1–8.
Du, W. and Piater, J. (2007). Multi-camera people
tracking by collaborative particle filters and princi-
pal axis-based integration. In Proceedings of the
8th Asian Conference on Computer Vision - Volume
Part I, ACCV’07, pages 365–374, Berlin, Heidelberg.
Springer-Verlag.
Fleuret, F., Berclaz, J., Lengagne, R., and Fua, P. (2008).
Multicamera people tracking with a probabilistic oc-
cupancy map. Pattern Analysis and Machine In-
telligence, IEEE Transactions on, 30(2):267–282.
[doi:10.1109/TPAMI.2007.1174].
Gruenwedel, S., Jela
˘
ca, V., Ni
˜
no Casta
˜
neda, J., Van Hese,
P., Van Cauwelaert, D., Van Haerenborgh, D., Vee-
laert, P., and Philips, W. (2014). Low-complexity
scalable distributed multi-camera tracking of humans.
ACM Transactions on Sensor Networks, 10(2).
Gr
¨
unwedel, S., Jelaa, V., Ni
˜
no Casta
˜
neda, J., Van Hese,
P., Van Cauwelaert, D., Veelaert, P., and Philips, W.
(2012). Decentralized tracking of humans using a
camera network. In Roning, J. and Casasent, D., ed-
itors, PROCEEDINGS OF SPIE, Intelligent Robots
and Computer Vision XXIX: Algorithms and Tech-
niques, volume 8301. SPIE.
Henriques, J. F., Caseiro, R., and Batista, J. (2011). Glob-
ally optimal solution to multi-object tracking with
merged measurements. In IEEE International Con-
Occlusion Robust Symbol Level Fusion for Multiple People Tracking
225