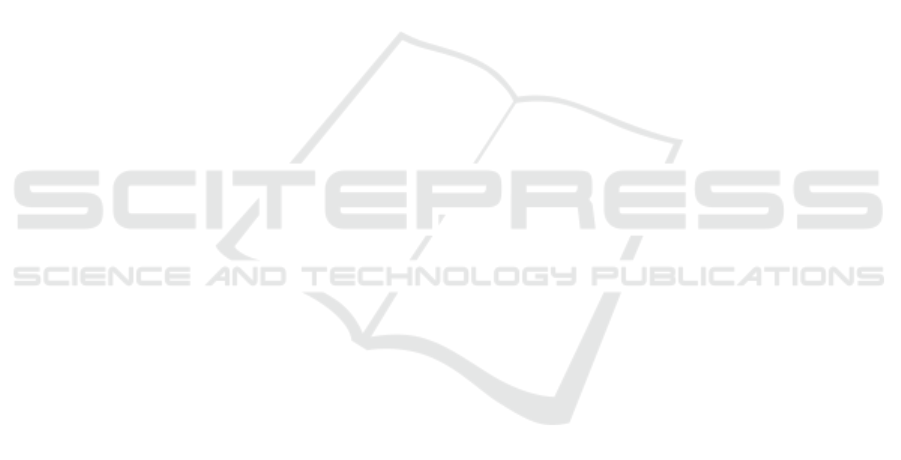
of Deep Learning might be to exploit unsupervised
approaches.
ACKNOWLEDGEMENTS
This work has been supported by Spanish MINECO
project TIN2014-61068-R.
REFERENCES
Babenko, A., Slesarev, A., Chigorin, A., and Lempitsky,
V. (2014). Neural codes for image retrieval. In Euro-
pean Conference on Computer Vision, pages 584–599.
Springer.
Bay, H., Tuytelaars, T., and Van Gool, L. (2006). Surf:
Speeded up robust features. In European conference
on computer vision, pages 404–417. Springer.
Bhushan, N., Rao, A. R., and Lohse, G. L. (1997). The
texture lexicon: Understanding the categorization of
visual texture terms and their relationship to texture
images. Cognitive Science, 21(2):219–246.
Caputo, B., Hayman, E., and Mallikarjuna, P. (2005). Class-
specific material categorisation. In Tenth IEEE Inter-
national Conference on Computer Vision (ICCV’05)
Volume 1, volume 2, pages 1597–1604. IEEE.
Cimpoi, M., Maji, S., Kokkinos, I., Mohamed, S., and
Vedaldi, A. (2014). Describing textures in the wild.
In Proceedings of the IEEE Conference on Computer
Vision and Pattern Recognition, pages 3606–3613.
Cimpoi, M., Maji, S., Kokkinos, I., and Vedaldi, A. (2016).
Deep filter banks for texture recognition, description,
and segmentation. International Journal of Computer
Vision, 118(1):65–94.
Dana, K. J., Van Ginneken, B., Nayar, S. K., and Koen-
derink, J. J. (1999). Reflectance and texture of
real-world surfaces. ACM Transactions on Graphics
(TOG), 18(1):1–34.
Deng, J., Dong, W., Socher, R., Li, L.-J., Li, K., and Fei-Fei,
L. (2009). Imagenet: A large-scale hierarchical image
database. In Computer Vision and Pattern Recogni-
tion, 2009. CVPR 2009. IEEE Conference on, pages
248–255. IEEE.
Gan, Y., Cai, X., Liu, J., and Wang, S. (2015). A texture re-
trieval scheme based on perceptual features. In 2015
Asia-Pacific Signal and Information Processing As-
sociation Annual Summit and Conference (APSIPA),
pages 897–900. IEEE.
He, K., Zhang, X., Ren, S., and Sun, J. (2015). Deep resid-
ual learning for image recognition. arXiv preprint
arXiv:1512.03385.
Jordan, A. (2002). On discriminative vs. generative clas-
sifiers: A comparison of logistic regression and naive
bayes. Advances in neural information processing sys-
tems, 14:841.
Krizhevsky, A., Sutskever, I., and Hinton, G. E. (2012). Im-
agenet classification with deep convolutional neural
networks. In Advances in neural information process-
ing systems, pages 1097–1105.
Lowe, D. G. (2004). Distinctive image features from scale-
invariant keypoints. International journal of computer
vision, 60(2):91–110.
Mikolajczyk, K. and Schmid, C. (2005). A perfor-
mance evaluation of local descriptors. IEEE trans-
actions on pattern analysis and machine intelligence,
27(10):1615–1630.
Ojala, T., Pietikainen, M., and Maenpaa, T. (2002). Mul-
tiresolution gray-scale and rotation invariant texture
classification with local binary patterns. IEEE Trans-
actions on pattern analysis and machine intelligence,
24(7):971–987.
Perronnin, F. and Dance, C. (2007). Fisher kernels on visual
vocabularies for image categorization. In 2007 IEEE
Conference on Computer Vision and Pattern Recogni-
tion, pages 1–8. IEEE.
Ren, S., He, K., Girshick, R., and Sun, J. (2015). Faster
r-cnn: Towards real-time object detection with region
proposal networks. In Advances in neural information
processing systems, pages 91–99.
Russakovsky, O., Deng, J., Su, H., Krause, J., Satheesh, S.,
Ma, S., Huang, Z., Karpathy, A., Khosla, A., Bern-
stein, M., et al. (2015). Imagenet large scale visual
recognition challenge. International Journal of Com-
puter Vision, 115(3):211–252.
Rust, N. C., Schwartz, O., Movshon, J. A., and Simoncelli,
E. P. (2005). Spatiotemporal elements of macaque v1
receptive fields. Neuron, 46(6):945–956.
Sharan, L., Liu, C., Rosenholtz, R., and Adelson, E. H.
(2013). Recognizing materials using perceptually in-
spired features. International journal of computer vi-
sion, 103(3):348–371.
Simonyan, K. and Zisserman, A. (2014). Very deep con-
volutional networks for large-scale image recognition.
arXiv preprint arXiv:1409.1556.
Szegedy, C., Liu, W., Jia, Y., Sermanet, P., Reed, S.,
Anguelov, D., Erhan, D., Vanhoucke, V., and Rabi-
novich, A. (2015). Going deeper with convolutions.
In Proceedings of the IEEE Conference on Computer
Vision and Pattern Recognition, pages 1–9.
Wang, N. and Yeung, D.-Y. (2013). Learning a deep
compact image representation for visual tracking. In
Advances in neural information processing systems,
pages 809–817.
Wu, P., Manjunath, B., Newsam, S., and Shin, H. (2000).
A texture descriptor for browsing and similarity re-
trieval. Signal Processing: Image Communication,
16(1):33–43.
Zeiler, M. D. and Fergus, R. (2014). Visualizing and under-
standing convolutional networks. In European Con-
ference on Computer Vision, pages 818–833. Springer.
Zheng, S., Jayasumana, S., Romera-Paredes, B., Vineet, V.,
Su, Z., Du, D., Huang, C., and Torr, P. H. (2015). Con-
ditional random fields as recurrent neural networks. In
Proceedings of the IEEE International Conference on
Computer Vision, pages 1529–1537.
Evaluation of Deep Image Descriptors for Texture Retrieval
257