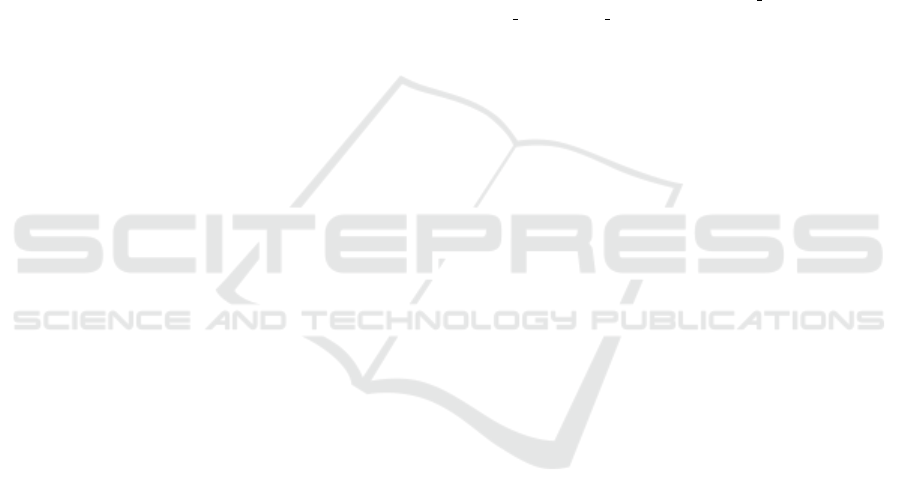
tive learning framework for enhancing the detection
of malicious pdf files. In Intelligence and Security In-
formatics Conference (JISIC), 2014 IEEE Joint, pages
91–98. IEEE.
Ofek, N., Katz, G., Shapira, B., and Bar-Zev, Y. (2015).
Sentiment analysis in transcribed utterances. In
Pacific-Asia Conference on Knowledge Discovery and
Data Mining, pages 27–38. Springer.
Ofek, N., Poria, S., Rokach, L., Cambria, E., Hussain, A.,
and Shabtai, A. (2016). Unsupervised commonsense
knowledge enrichment for domain-specific sentiment
analysis. Cognitive Computation, 8(3):467–477.
Ofek, N., Rokach, L., and Mitra, P. (2014). Methodology
for connecting nouns to their modifying adjectives. In
International Conference on Intelligent Text Process-
ing and Computational Linguistics, pages 271–284.
Springer.
Ofek, N. and Shabtai, A. (2014). Dynamic latent expertise
mining in social networks. IEEE Internet Computing,
18(5):20–27.
Onix (2016). Onix text retrieval toolkit.
http://www.lextek.com/manuals/onix/stopwords1.html.
[Online; accessed April 2016].
Oommen, T., Baise, L. G., and Vogel, R. M. (2011).
Sampling bias and class imbalance in maximum-
likelihood logistic regression. Mathematical Geo-
sciences, 43(1):99–120.
Pang, B., Lee, L., and Vaithyanathan, S. (2002). Thumbs
up?: sentiment classification using machine learn-
ing techniques. In Proceedings of the ACL-02 con-
ference on Empirical methods in natural language
processing-Volume 10, pages 79–86. Association for
Computational Linguistics.
Portier, K., Greer, G. E., Rokach, L., Ofek, N., Wang, Y.,
Biyani, P., Yu, M., Banerjee, S., Zhao, K., Mitra, P.,
et al. (2013). Understanding topics and sentiment in
an online cancer survivor community. JNCI Mono-
graphs, 47:195–198.
Portokalidis, G., Homburg, P., Anagnostakis, K., and Bos,
H. (2010). Paranoid android: versatile protection for
smartphones. In Proceedings of the 26th Annual Com-
puter Security Applications Conference, pages 347–
356. ACM.
Quinlan, J. R. (1993). C4. 5: Programming for machine
learning. Morgan Kauffmann, page 38.
Ranveer, S. and Hiray, S. (2015). Comparative analy-
sis of feature extraction methods of malware detec-
tion. International Journal of Computer Applications,
120(5).
Rastogi, V., Chen, Y., and Jiang, X. (2013). Droid-
chameleon: evaluating android anti-malware against
transformation attacks. In Proceedings of the 8th
ACM SIGSAC symposium on Information, computer
and communications security, pages 329–334. ACM.
Shabtai, A., Tenenboim-Chekina, L., Mimran, D., Rokach,
L., Shapira, B., and Elovici, Y. (2014). Mobile mal-
ware detection through analysis of deviations in ap-
plication network behavior. Computers & Security,
43:1–18.
Singh, Y., Kaur, A., and Malhotra, R. (2009). Comparative
analysis of regression and machine learning methods
for predicting fault proneness models. International
journal of computer applications in technology, 35(2-
4):183–193.
snoopwall (2014). Summarized privacy and risk
analysis of top 10 android flashlight apps.
http://www.snoopwall.com/threat-reports-10-01-
2014/. [Online; accessed April 2016].
Statista (2016). Number of available apps in the
apple app store from july 2008 to june 2015.
http://www.statista.com/statistics/263795/number-
of-available-apps-in-the-apple-app-store/. [Online;
accessed April 2016].
Total, V. (2016). Virustotal, a free online service that
analyzes files and urls enabling the identification of
viruses, worms, trojans and other kinds of malicious
content. https://www.virustotal.com/. [Online; ac-
cessed April 2016].
Twitter (2016). Twitter dictionary: A
guide to understanding twitter lingo.
http://www.webopedia.com/quick
ref/Twitter
Dictionaryx Guide.asp. [Online; accessed April
2016].
ˇ
Srndic, N. and Laskov, P. (2013). Detection of malicious pdf
files based on hierarchical document structure. In Pro-
ceedings of the 20th Annual Network & Distributed
System Security Symposium.
Walker, S. H. and Duncan, D. B. (1967). Estimation of the
probability of an event as a function of several inde-
pendent variables. Biometrika, 54(1-2):167–179.
Wang, K. and Stolfo, S. J. (2004). Anomalous payload-
based network intrusion detection. In International
Workshop on Recent Advances in Intrusion Detection,
pages 203–222. Springer.
WEKA (2016). http://www.cs.waikato.ac.nz/ml/weka/.
[Online; accessed April 2016].
Xie, L., Zhang, X., Seifert, J.-P., and Zhu, S. (2010). pb-
mds: a behavior-based malware detection system for
cellphone devices. In Proceedings of the third ACM
conference on Wireless network security, pages 37–
48. ACM.
Yang, Z., Yang, M., Zhang, Y., Gu, G., Ning, P., and
Wang, X. S. (2013). Appintent: Analyzing sensitive
data transmission in android for privacy leakage detec-
tion. In Proceedings of the 2013 ACM SIGSAC confer-
ence on Computer & communications security, pages
1043–1054. ACM.
Ye, Q., Zhang, Z., and Law, R. (2009). Sentiment classifica-
tion of online reviews to travel destinations by super-
vised machine learning approaches. Expert Systems
with Applications, 36(3):6527–6535.
Zhang, Y., Yang, M., Xu, B., Yang, Z., Gu, G., Ning, P.,
Wang, X. S., and Zang, B. (2013). Vetting undesir-
able behaviors in android apps with permission use
analysis. In Proceedings of the 2013 ACM SIGSAC
conference on Computer & communications security,
pages 611–622. ACM.
Zheng, M., Sun, M., and Lui, J. C. (2013). Droid analyt-
ics: a signature based analytic system to collect, ex-
tract, analyze and associate android malware. In 2013
12th IEEE International Conference on Trust, Secu-
rity and Privacy in Computing and Communications,
pages 163–171. IEEE.
ICISSP 2017 - 3rd International Conference on Information Systems Security and Privacy
94