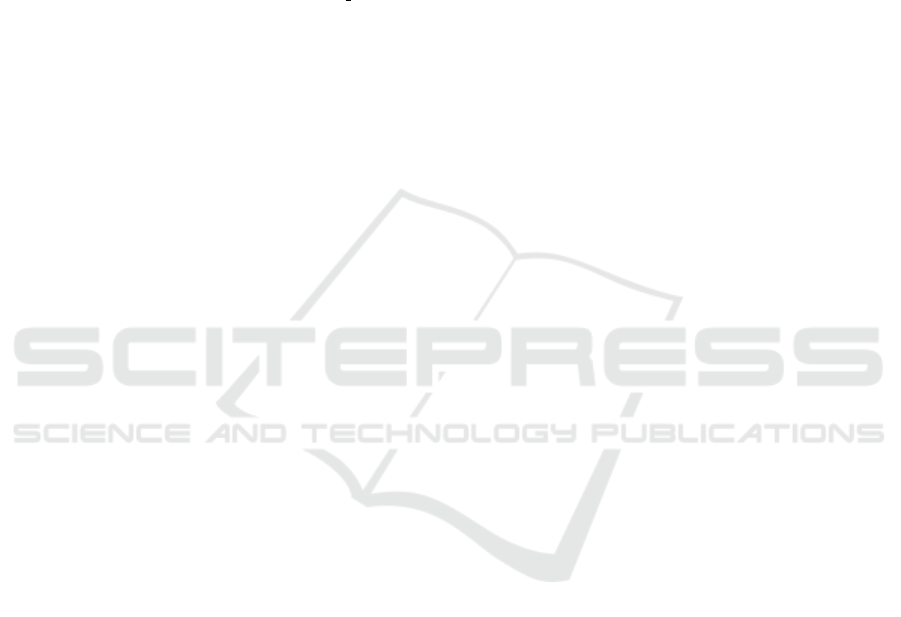
Black, M. J. and Anandan, P. (1996). The Robust Estima-
tion of Multiple Motions: Parametric and Piecewise-
Smooth Flow Fields. Computer Vision and Image Un-
derstanding, 63(1):75–104.
Brox, T. and Malik, J. (2011). Large Displacement Optical
Flow: Descriptor Matching in Variational Motion Es-
timation. IEEE Transactions on Pattern Analysis and
Machine Intelligence, 33(3):500–513.
Brox, T. and Weickert, J. (2002). Nonlinear Matrix Diffu-
sion for Optic Flow Estimation. In Gool, L. V., editor,
Pattern Recognition, number 2449 in Lecture Notes
in Computer Science, pages 446–453. Springer Berlin
Heidelberg. DOI: 10.1007/3-540-45783-6 54.
Chen, Z., Jin, H., Lin, Z., Cohen, S., and Wu, Y.
(2013). Large Displacement Optical Flow from Near-
est Neighbor Fields. pages 2443–2450. IEEE.
Farneback, G. (2000). Fast and accurate motion estimation
using orientation tensors and parametric motion mod-
els. volume 1, pages 135–139. IEEE Comput. Soc.
Horn, B. K. and Schunck, B. G. (1981). Determining optical
flow. Artificial Intelligence, 17(1-3):185–203.
Kim, T. H., Lee, H. S., and Lee, K. M. (2013). Optical Flow
via Locally Adaptive Fusion of Complementary Data
Costs. pages 3344–3351. IEEE.
Liu, H., Chellappa, R., and Rosenfeld, A. (2003). Accurate
dense optical flow estimation using adaptive structure
tensors and a parametric model. IEEE Transactions
on Image Processing, 12(10):1170–1180.
Lucas, B. D. and Kanade, T. (1981). An Iterative Image
Registration Technique with an Application to Stereo
Vision. pages 674–679.
Middendorf, M. and Nagel, H. H. (2001). Estimation and
interpretation of discontinuities in optical flow fields.
In Eighth IEEE International Conference on Com-
puter Vision, 2001. ICCV 2001. Proceedings, vol-
ume 1, pages 178–183 vol.1.
Nagel, H.-H. and Gehrke, A. (1998). Spatiotemporally
adaptive estimation and segmentation of OF-fields. In
Burkhardt, H. and Neumann, B., editors, Computer
Vision — ECCV’98, number 1407 in Lecture Notes
in Computer Science, pages 86–102. Springer Berlin
Heidelberg. DOI: 10.1007/BFb0054735.
Sun, D., Roth, S., and Black, M. J. (2010). Secrets of opti-
cal flow estimation and their principles. pages 2432–
2439. IEEE.
Wedel, A., Pock, T., Zach, C., Bischof, H., and Cremers,
D. (2009). An Improved Algorithm for TV-L 1 Op-
tical Flow. In Hutchison, D., Kanade, T., Kittler, J.,
Kleinberg, J. M., Mattern, F., Mitchell, J. C., Naor, M.,
Nierstrasz, O., Pandu Rangan, C., Steffen, B., Sudan,
M., Terzopoulos, D., Tygar, D., Vardi, M. Y., Weikum,
G., Cremers, D., Rosenhahn, B., Yuille, A. L., and
Schmidt, F. R., editors, Statistical and Geometrical
Approaches to Visual Motion Analysis, volume 5604,
pages 23–45. Springer Berlin Heidelberg, Berlin, Hei-
delberg.
Weinzaepfel, P., Revaud, J., Harchaoui, Z., and Schmid, C.
(2013). DeepFlow: Large displacement optical flow
with deep matching. In IEEE Intenational Conference
on Computer Vision (ICCV), Sydney, Australia.
Xu, L., Jia, J., and Matsushita, Y. (2010). Motion detail
preserving optical flow estimation. pages 1293–1300.
IEEE.
Yang, J. and Li, H. (2015). Dense, accurate optical flow
estimation with piecewise parametric model. pages
1019–1027. IEEE.
yves Bouguet, J. (2000). Pyramidal implementation of the
lucas kanade feature tracker. Intel Corporation, Mi-
croprocessor Research Labs.
VISAPP 2017 - International Conference on Computer Vision Theory and Applications
458