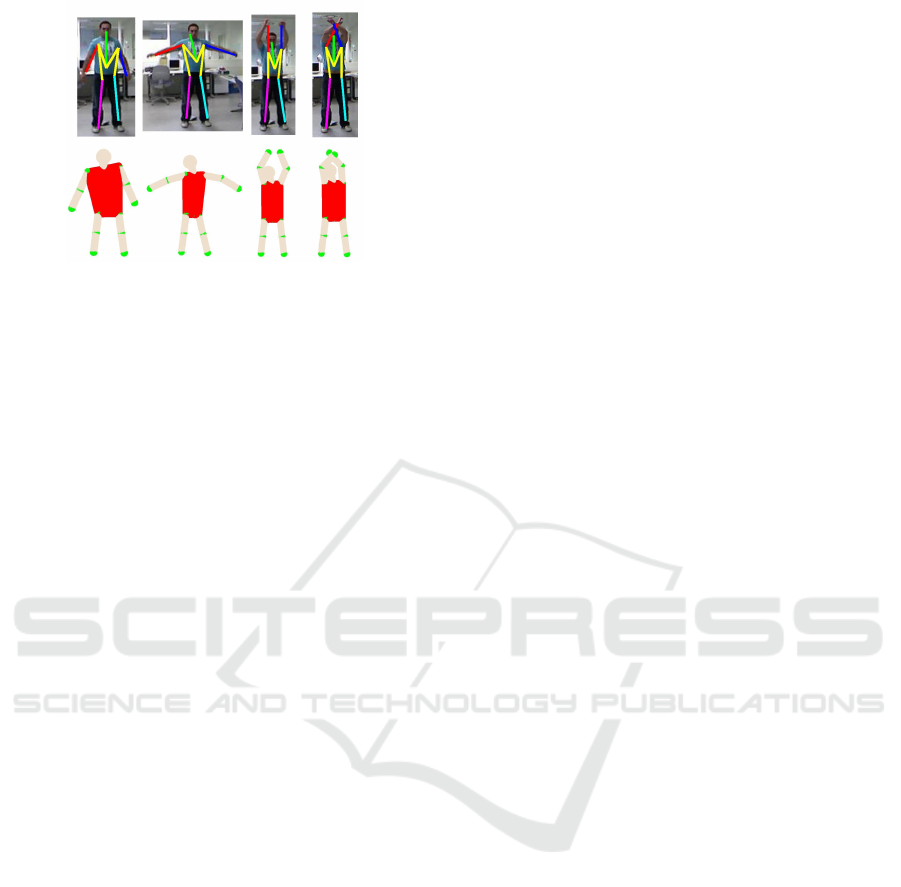
Figure 8: Body human model calculated after obtain the
points by our proposed DPM model.
technique ideal for Depth channels of RGBD data that
helps us improve our results further.
Finally, we reduce the computational cost of our
new DPM model by a novel approach solving kine-
matic equations. Our results show significant results
over the standard DPM model in our dataset and in
the publicly available CAD60 dataset.
ACKNOWLEDGEMENTS
This work was partially financed by Plan Nacional
de I+D, Comision Interministerial de Ciencia y Tec-
nologa (FEDER-CICYT) under the project DPI2013-
44227-R.
REFERENCES
Berti, E. M., Salmer
´
on, A. J. S., and Benimeli, F. (2012).
Human–robot interaction and tracking using low cost
3d vision systems. Romanian Journal of Technical
Sciences - Applied Mechanics, 7(2):1–15.
Everingham, M., Van Gool, L., Williams, C. K., Winn, J.,
and Zisserman, A. (2010). The pascal visual object
classes (voc) challenge. International journal of com-
puter vision, 88(2):303–338.
Felzenszwalb, P., McAllester, D., and Ramanan, D. (2008).
A discriminatively trained, multiscale, deformable
part model. In 2013 IEEE Conference on Computer
Vision and Pattern Recognition, pages 1–8. IEEE.
Felzenszwalb, P. F., Girshick, R. B., McAllester, D., and
Ramanan, D. (2010). Object detection with discrim-
inatively trained part-based models. Pattern Analy-
sis and Machine Intelligence, IEEE Transactions on,
32(9):1627–1645.
Felzenszwalb, P. F. and Huttenlocher, D. P. (2005). Pic-
torial structures for object recognition. International
Journal of Computer Vision, 61(1):55–79.
Gupta, R., Chia, A. Y.-S., and Rajan, D. (2013). Human
activities recognition using depth images. In Proceed-
ings of the 21st ACM international conference on Mul-
timedia, pages 283–292. ACM.
Khalil, W. and Dombre, E. (2004). Modeling, identification
and control of robots. Butterworth-Heinemann.
Matas, J., Chum, O., Urban, M., and Pajdla, T. (2004).
Robust wide-baseline stereo from maximally sta-
ble extremal regions. Image and vision computing,
22(10):761–767.
Ni, B., Pei, Y., Moulin, P., and Yan, S. (2013). Multi-
level depth and image fusion for human activity detec-
tion. Cybernetics, IEEE Transactions on, 43(5):1383–
1394.
R. Faria, D., Premebida, C., and Nunes, U. (2014). A
probalistic approach for human everyday activities
recognition using body motion from rgb-d images.
IEEE RO-MAN’14: IEEE International Symposium
on Robot and Human Interactive Communication.
Saffari, A., Leistner, C., Santner, J., Godec, M., and
Bischof, H. (2009). On-line random forests. In
Computer Vision Workshops (ICCV Workshops), 2009
IEEE 12th International Conference on, pages 1393–
1400. IEEE.
Shan, J. and Akella, S. (2014). 3d human action segmenta-
tion and recognition using pose kinetic energy. IEEE
Workshop on Advanced Robotics and its Social Im-
pacts (ARSO).
Shotton, J., Sharp, T., Kipman, A., Fitzgibbon, A., Finoc-
chio, M., Blake, A., Cook, M., and Moore, R. (2013).
Real-time human pose recognition in parts from sin-
gle depth images. Communications of the ACM,
56(1):116–124.
Song, S. and Xiao, J. (2014). Sliding shapes for 3d object
detection in depth images. In Computer Vision–ECCV
2014, pages 634–651. Springer.
Viala, C. R., Salmeron, A. J. S., and Martinez-Berti, E.
(2011). Calibration of a wide angle stereoscopic sys-
tem. OPTICS LETTERS, ISSN 0146-9592, pag 3064-
3067.
Viala, C. R., Salmeron, A. J. S., and Martinez-Berti, E.
(2012). Accurate calibration with highly distorted im-
ages. APPLIED OPTICS, ISSN 0003-6935, pag 89-
101.
Waldron Prof, K. and Schmiedeler Prof, J. (2008). Kine-
matics. Springer Berlin Heidelberg.
Wang, J., Liu, Z., and Wu, Y. (2014). Learning actionlet
ensemble for 3d human action recognition. In Human
Action Recognition with Depth Cameras, pages 11–
40. Springer.
Wang, Y., Tran, D., Liao, Z., and Forsyth, D. (2012). Dis-
criminative hierarchical part-based models for human
parsing and action recognition. The Journal of Ma-
chine Learning Research, 13(1):3075–3102.
Yang, Y. and Ramanan, D. (2013). Articulated human de-
tection with flexible mixtures of parts. Pattern Anal-
ysis and Machine Intelligence, IEEE Transactions on,
35(12):2878–2890.
VISAPP 2017 - International Conference on Computer Vision Theory and Applications
288