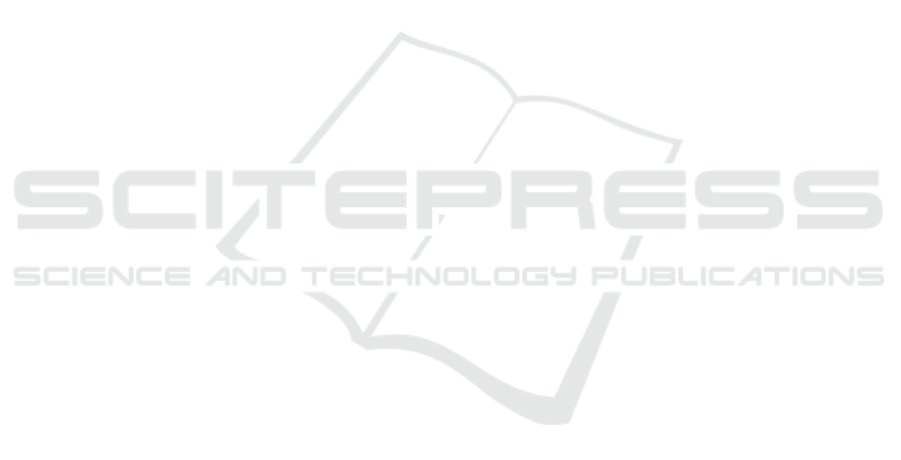
body, which makes this algorithm more flexible than
the other ones. This characteristic and using skele-
tal data, made this algorithm to be used in the case
for which the information of some inactive joints are
missing. The local temporal features were extracted
using overlapped sliding windows over the trajectory
of each joint, and the global temporal information was
taken into account using the HMM classifier. Also,
there is a rich possibility for extensions. In this pa-
per, there is no contribution to feature extraction, and
this belief exists that by using more discriminative
features, the final accuracy of the method can be im-
proved. Thus, as a future work, the state-of-the-art
feature extraction methods can be used. Using more
powerful quantization method instead of K-means can
also improve the results.
REFERENCES
Boubou, S. and Suzuki, E. (2015). Classifying actions
based on histogram of oriented velocity vectors. Jour-
nal of Intelligent Information Systems, 44(1):49–65.
Devanne, M., Wannous, H., Berretti, S., Pala, P., Daoudi,
M., and Del Bimbo, A. (2013). Space-time pose repre-
sentation for 3d human action recognition. In Interna-
tional Conference on Image Analysis and Processing,
pages 456–464. Springer.
Eweiwi, A., Cheema, M. S., Bauckhage, C., and Gall, J.
(2014). Efficient pose-based action recognition. In
Asian Conference on Computer Vision, pages 428–
443. Springer.
F. De la Torre, J. Hodgins, J. M. S. V. R. F. and Macey., J.
(2009). Tech. report cmu-ri-tr-08-22. Technical re-
port, Robotics Institute, Carnegie Mellon University.
Gu, Y., Do, H., Ou, Y., and Sheng, W. (2012). Human ges-
ture recognition through a kinect sensor. In Robotics
and Biomimetics (ROBIO), 2012 IEEE International
Conference on, pages 1379–1384. IEEE.
Hussein, M. E., Torki, M., Gowayyed, M. A., and El-Saban,
M. (2013). Human action recognition using a tem-
poral hierarchy of covariance descriptors on 3d joint
locations. In IJCAI, volume 13, pages 2466–2472.
Junejo, I. N., Dexter, E., Laptev, I., and Perez, P. (2011).
View-independent action recognition from temporal
self-similarities. IEEE transactions on pattern anal-
ysis and machine intelligence, 33(1):172–185.
Li, W., Zhang, Z., and Liu, Z. (2010). Action recognition
based on a bag of 3d points. In 2010 IEEE Computer
Society Conference on Computer Vision and Pattern
Recognition-Workshops, pages 9–14. IEEE.
Liu, Z., Feng, X., and Tian, Y. (2015). An effective view
and time-invariant action recognition method based on
depth videos. In 2015 Visual Communications and
Image Processing (VCIP), pages 1–4. IEEE.
Luo, J., Wang, W., and Qi, H. (2013). Group sparsity and
geometry constrained dictionary learning for action
recognition from depth maps. In Proceedings of the
IEEE International Conference on Computer Vision,
pages 1809–1816.
Oreifej, O. and Liu, Z. (2013). Hon4d: Histogram of ori-
ented 4d normals for activity recognition from depth
sequences. In Proceedings of the IEEE Conference
on Computer Vision and Pattern Recognition, pages
716–723.
Primer, A., Burrus, C. S., and Gopinath, R. A. (1998). In-
troduction to wavelets and wavelet transforms.
Rahmani, H., Mahmood, A., Huynh, D. Q., and Mian, A.
(2014). Real time action recognition using histograms
of depth gradients and random decision forests. In
IEEE Winter Conference on Applications of Computer
Vision, pages 626–633. IEEE.
Shotton, J., Sharp, T., Kipman, A., Fitzgibbon, A., Finoc-
chio, M., Blake, A., Cook, M., and Moore, R. (2013).
Real-time human pose recognition in parts from sin-
gle depth images. Communications of the ACM,
56(1):116–124.
Vemulapalli, R., Arrate, F., and Chellappa, R. (2014). Hu-
man action recognition by representing 3d skeletons
as points in a lie group. In Proceedings of the IEEE
Conference on Computer Vision and Pattern Recogni-
tion, pages 588–595.
Wei, P., Zheng, N., Zhao, Y., and Zhu, S.-C. (2013). Con-
current action detection with structural prediction. In
Proceedings of the IEEE International Conference on
Computer Vision, pages 3136–3143.
Xia, L. and Aggarwal, J. (2013). Spatio-temporal depth
cuboid similarity feature for activity recognition using
depth camera. In Proceedings of the IEEE Conference
on Computer Vision and Pattern Recognition, pages
2834–2841.
Xia, L., Chen, C.-C., and Aggarwal, J. (2012). View invari-
ant human action recognition using histograms of 3d
joints. In 2012 IEEE Computer Society Conference on
Computer Vision and Pattern Recognition Workshops,
pages 20–27. IEEE.
Yang, X. and Tian, Y. (2014). Super normal vector for activ-
ity recognition using depth sequences. In Proceedings
of the IEEE Conference on Computer Vision and Pat-
tern Recognition, pages 804–811.
Yang, X. and Tian, Y. L. (2012). Eigenjoints-based ac-
tion recognition using naive-bayes-nearest-neighbor.
In 2012 IEEE Computer Society Conference on Com-
puter Vision and Pattern Recognition Workshops,
pages 14–19. IEEE.
VISAPP 2017 - International Conference on Computer Vision Theory and Applications
310