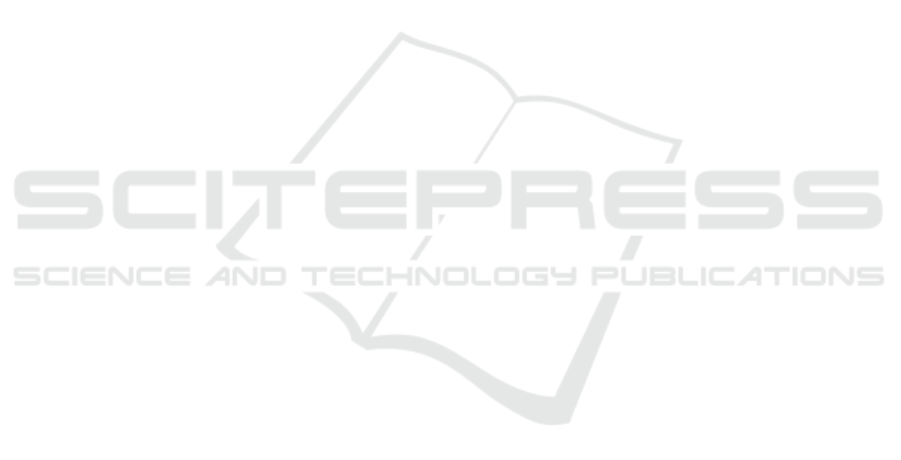
the characteristics of each point of the vascular tree
structure to classify the vessels. We use the k-means
clustering technique with the feature vectors obtained
from the extracted vessel profiles. The employed fea-
tures consist of two components, the mean of the H
component (from HSL color space) and the variance
of the R component (from RGB color space).
The methodology was tested with 19 near-infrared
reflectance retinographies included in 19 OCT patient
scans. The method was trained with 5 images whereas
the validation set included the rest of 14 images. From
these images, 405 vessel segments and 14,745 vessel
coordinates were identified and manually labeled by
an expert clinician. As shown, the method offered
promising results. Regarding the vessel coordinates,
the method provided an accuracy of 88.38% as veins
or arteries. In the case of vascular segments, the re-
sults obtained are around a 93%. The reason of this
increase in the performance is the majority voting pro-
cess (propagation) that discriminates each vessel into
artery or vein. Finally, we made a comparison be-
tween various methods proposed in the literature. The
obtained results show a correct result in comparison
with the rest of the approaches.
Our study has some drawbacks. First, the differen-
tiation of retinal vessels depends on the image quality.
We can see that in the small vessels of the retina char-
acteristics are similar to both clusters. This indicates
the need of a study with a larger set of features. Sec-
ondly, this study does not consider the problems that
normally appear at the intersections: crossings and bi-
furcations. These landmarks provide important infor-
mation that can be used to construct a graph connect-
ing the vessel segments. Increasing vascular struc-
ture would enhance the efficiency of the methodology
mainly in the voting phase and propagation of classi-
fied points.
Despite of the promising results, there still ex-
ists some points that will be attempt as future works.
First of all, we need to improve the phases of the
method, in order to increase the success rates that
were achieved. A greater set of features can be con-
sidered as well as testing other classifiers can increase
the success rate. Future plans include development of
automated methods for calculation the arterio-venous
ratio (AVR). We validated the proposal with the near-
infrared reflectance retinographies that are provided
in combination with the histological sections of the
OCT images. Future versions of the methodology
will combine the depth information of the histologi-
cal sections to analyze the real layout, 3D, of the eye
fundus. Ultimately, this methodology could be incor-
porated into a computer-aided system for detection of
diabetic retinopathy, or other eye-related diseases.
ACKNOWLEDGEMENTS
This work is supported by the Instituto de Salud Car-
los III, Government of Spain and FEDER funds of
the European Union through the PI14/02161 and the
DTS15/00153 research projects and by the Ministerio
de Econom
´
ıa y Competitividad, Government of Spain
through the DPI2015-69948-R research project.
REFERENCES
Abu, A. (2008). Oct in diabetic dacular edema. Acta Oph-
thalmologica, 86.
Blanco, M., Penedo, M. G., Barreira, N., Penas, M., and
Carreira, M. J. (2006). Localization and extraction
of the optic disc using the fuzzy circular hough trans-
form. Artificial Intelligence and Soft Computing,
pages 712–721.
Brezinski, M. E. (2006). Optical coherence tomography.
Elsevier Academic Press, Burlington, Mass.
Caderno, I. G., Penedo, M. G., Barreira, N., Marino, C., and
Gonzalez, F. (2005). Precise detection and measure-
ment of the retina vascular tree. Pattern Recognition
and Image Analysis: Advances in Mathematical The-
ory and Applications, 15:523–526.
Calvo, D., Ortega, M., Penedo, M., and Rouco, J. (2011).
Automatic detection and characterisation of retinal
vessel tree bifurcations and crossovers in eye fun-
dus images. Computer Methods and Programs in
Biomedicine, 103:28–38.
Cordero, A. (2011). Scientific realism and the divide et im-
pera strategy: The ether saga revisited. Philosophy of
Science, 78(5):1120–1130.
Dashtbozorg, B., Mendonca, Maria, A., and Campilho,
A. (2014). An automatic graph-based approach for
artery/vein classification in retinal images. IEEE
Transactions on Image Processing, 23:1073–1083.
Duker, J. S., Waheed, N. K., and Goldman, D. (2014).
Handbook of retinal OCT.
Grisan, E. and Ruggeri, A. (2003). A divide et impera strat-
egy for automatic classification of retinal vessels into
arteries and veins. Engineering in Medicine and Biol-
ogy Society, 1:890–893.
Joshi, V. S., Reinhardt, J. M., Garvin, M. K., and Abramoff,
M. D. (2014). Automated method for identification
and artery-venous classification of vessel trees in reti-
nal vessel networks. PLoS ONE, 9(2).
Kondermann, C., Kondermann, D., and Yan, M. (2007).
Blood vessel classification into arteries and veins in
retinal images. Proc. SPIE, 6512.
Pascolini, DonatellaMariotti, S. P. (2011). Global estimates
of visual impairment: 2010. British Journal of Oph-
thalmology, 96(5):614–618.
V
´
azquez, S. G., Cancela, B., Barreira, N., Penedo, M. G.,
Rodr
´
ıguez-Blanco, M., Pena Seijo, M., de Tuero,
G. C., Barcel
´
o, M. A., and Saez, M. (2013). Improv-
ing retinal artery and vein classification by means of a
VISAPP 2017 - International Conference on Computer Vision Theory and Applications
376