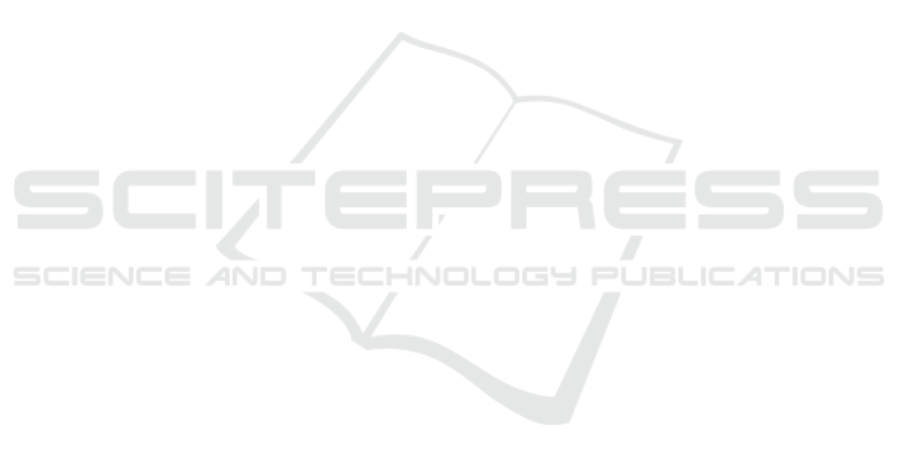
ference on Computer Vision and Pattern Recognition
(CVPR) Workshops.
Baroffio, L., Bondi, L., Cesana, M., Redondi, A. E., and
Tagliasacchi, M. (2015). A visual sensor network for
parking lot occupancy detection in smart cities. In In-
ternet of Things (WF-IoT), 2015 IEEE 2nd World Fo-
rum on, pages 745–750.
Bhaskar, H., Werghi, N., and Al-Mansoori, S. (2011).
Rectangular empty parking space detection using sift
based classification. In VISAPP, pages 214–220.
De Almeida, P. R. L., Oliveira, L. S., Britto, A. S., Silva,
E. J., and Koerich, A. L. (2015). PKLot-A robust
dataset for parking lot classification. Expert Systems
with Applications, 42(11):4937–4949.
Funck, S., Mohler, N., and Oertel, W. (2004). Determining
Car-Park Occupancy from Single Images. Intelligent
Vehicles Symposium, 2004 IEEE, pages 325–328.
Glorot, X., Bordes, A., and Bengio, Y. (2011). Deep sparse
rectifier neural networks. In Gordon, G. J. and Dun-
son, D. B., editors, Proceedings of the Fourteenth In-
ternational Conference on Artificial Intelligence and
Statistics (AISTATS-11), volume 15, pages 315–323.
Journal of Machine Learning Research - Workshop
and Conference Proceedings.
Huang, C. C. and Vu, H. T. (2015). A multi-layer dis-
criminative framework for parking space detection. In
2015 IEEE 25th International Workshop on Machine
Learning for Signal Processing (MLSP), pages 1–6.
Huang, J. and You, S. (2016). Vehicle detection in urban
point clouds with orthogonal-view convolutional neu-
ral network. In 2016 IEEE International Conference
on Image Processing (ICIP), pages 2593–2597.
Klosowski, M., Wojcikowski, M., and Czyzewski, A.
(2015). Vision-based parking lot occupancy evalua-
tion system using 2D separable discrete wavelet trans-
form. Bull. Polish Acad. Sci. Tech. Sci., 63(3):569–
573.
Krizhevsky, A., Sutskever, I., and Hinton, G. E. (2012). Im-
ageNet Classification with Deep Convolutional Neu-
ral Networks. Adv. Neural Inf. Process. Syst., pages
1–9.
Lecun, Y., Bengio, Y., and Hinton, G. (2015). Deep learn-
ing. Nature, 521(1):436–444.
Masmoudi, I., Wali, A., Jamoussi, A., and Alimi, A. M.
(2014). Vision based system for vacant parking lot de-
tection: Vpld. In Proceedings of the 9th International
Conference on Computer Vision Theory and Applica-
tions (VISIGRAPP 2014), pages 526–533.
Masmoudi, I., Wali, A., Jamoussi, A., and Alimi, M. A.
(2016). Trajectory analysis for parking lot vacancy
detection system. IET Intelligent Transport Systems,
10(7):461–468.
Russakovsky, O., Deng, J., Su, H., Krause, J., Satheesh,
S., Ma, S., Huang, Z., Karpathy, A., Khosla, A.,
Bernstein, M., Berg, A. C., and Fei-Fei, L. (2015).
ImageNet Large Scale Visual Recognition Challenge.
International Journal of Computer Vision (IJCV),
115(3):211–252.
Shoup, D. C. (2006). Cruising for parking. Transport Pol-
icy, 13(6):479–486.
Simard, P., Steinkraus, D., and Platt, J. C. (2003). Best Prac-
tices for Convolutional Neural Networks Applied to
Visual Document Analysis. Proc. 7th Int. Conf. Doc.
Anal. Recognit., pages 958–963.
Sukhinskiy, I. V., Nepovinnykh, E. A., and Radchenko,
G. I. (2016). Developing a parking monitoring sys-
tem based on the analysis of images from an out-
door surveillance camera. In 2016 39th International
Convention on Information and Communication Tech-
nology, Electronics and Microelectronics (MIPRO),
pages 1603–1607.
Theano Development Team (2016). Theano: A Python
framework for fast computation of mathematical ex-
pressions. arXiv e-prints, abs/1605.02688.
True, N. (2007). Vacant parking space detection in static
images. University of California, San Diego.
Tschentscher, M., Koch, C., Konig, M., Salmen, J., and
Schlipsing, M. (2015). Scalable real-time parking lot
classification: An evaluation of image features and su-
pervised learning algorithms. In 2015 International
Joint Conference on Neural Networks (IJCNN), pages
1–8.
Valipour, S., Siam, M., Stroulia, E., and Jagersand, M.
(2016). Parking stall vacancy indicator system based
on deep convolutional neural networks.
Wilson, D. and Martinez, T. R. (2003). The general ineffi-
ciency of batch training for gradient descent learning.
Neural Networks, 16(10):1429 – 1451.
Zheng, N. and Geroliminis, N. (2016). Modeling and op-
timization of multimodal urban networks with lim-
ited parking and dynamic pricing. Transportation Re-
search Part B: Methodological, 83:36 – 58.
VISAPP 2017 - International Conference on Computer Vision Theory and Applications
318