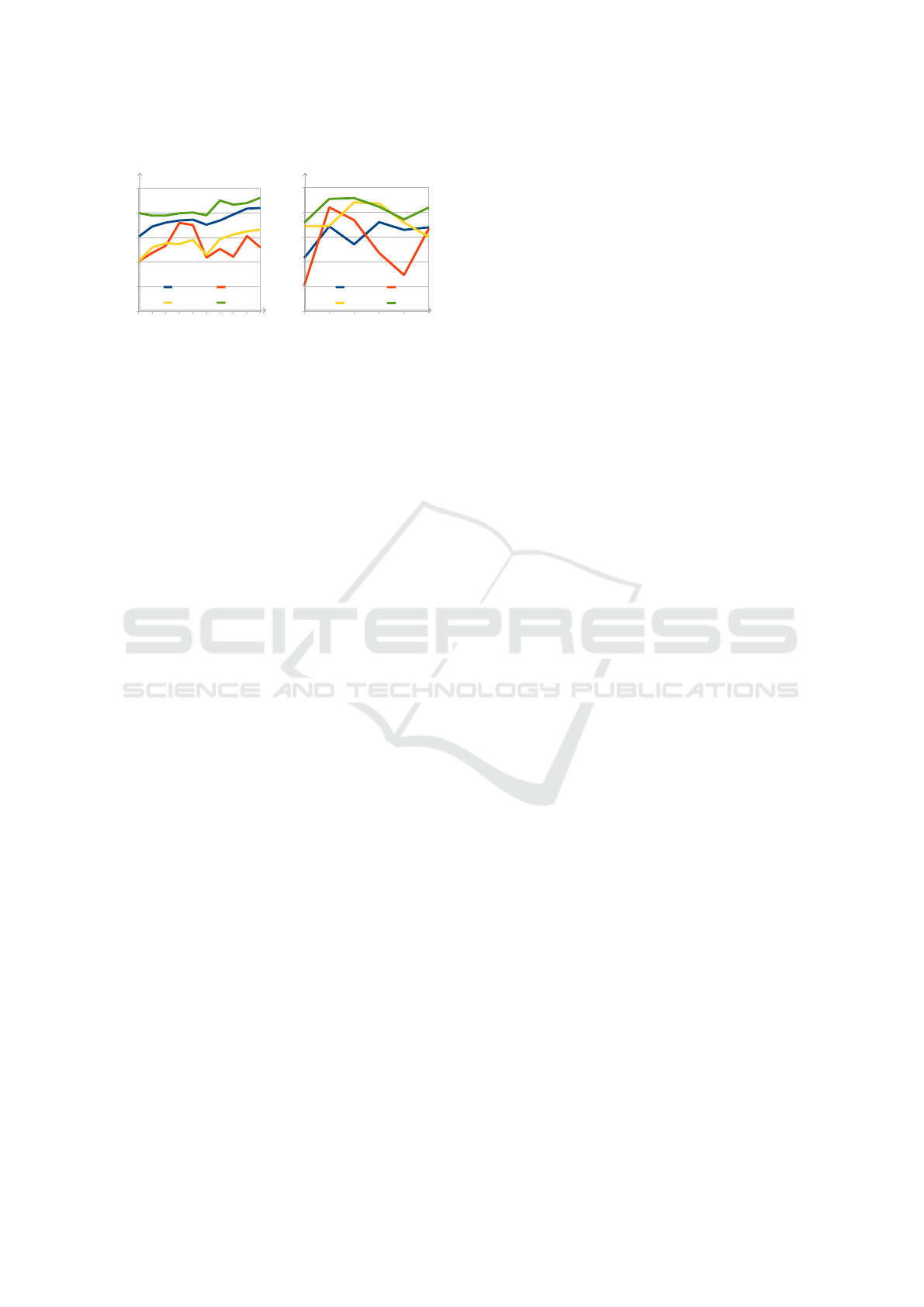
1 2 3 4 5 6 7 8 9 10
0.2
0.3
0.4
0.5
0.6
0.7
MOG2 GMG
ViBe Ours
F
1
image
1 2 3 4 5 6
0
0.2
0.4
0.6
0.8
1
MOG2 GMG
ViBe Ours
F
1
image
Figure 11: Comparison of the best configuration of each
algorithm for the football and Toscana data. Our algorithm
consistently outperforms the other methods for the football
dataset and is better for most images of the Toscana dataset.
ing with fast-moving objects that blend with the back-
ground. We plan to explore solutions to these prob-
lems using texture and geometric information. An-
other direction would be to experiment with color
spaces other than RGB whose channels are less cor-
related as it could increase the quality of our least
squares solution. Lastly, we plan to extend the pro-
posed method to operate under variable illumina-
tion conditions, dynamic backgrounds, and non-static
cameras.
REFERENCES
Barnich, O. and Van Droogenbroeck, M. (2011). ViBe:
A Universal Background Subtraction Algorithm for
Video Sequences. IEEE Transactions on Image Pro-
cessing, 20(6):1709–1724.
Chen, C.-C. and Aggarwal, J. (2010). Human Shadow Re-
moval with Unknown Light Source. In 20th Interna-
tional Conference on Pattern Recognition, volume 27,
pages 2407–2410, Istanbul, Turkey.
Duncan, K. and Sarkar, S. (2012). Saliency in images and
video: a brief survey. IET Computer Vision, 6(6):514–
523.
Godbehere, A. B. and Goldberg, K. (2014). Algorithms for
Visual Tracking of Visitors Under Variable-Lighting
Conditions for a Responsive Audio Art Installation.
In Controls and Art, pages 181–204. Springer Inter-
national Publishing, Cham.
Graham, T. (2012). Sports tv applications of computer
vision. BBC Research & Development White Paper
WHP220.
Hsieh, J.-W., Hu, W.-F., Chang, C.-J., and Chen, Y.-
S. (2003). Shadow elimination for effective moving
object detection by Gaussian shadow modeling. Im-
age and Vision Computing, 21(6):505–516.
Huerta, I., Amato, A., Roca, X., and Gonz
´
alez, J. (2013).
Exploiting multiple cues in motion segmentation
based on background subtraction. Neurocomputing,
100:183–196.
Kaimakis, P. and Tsapatsoulis, N. (2013). Background
Modeling Methods for Visual Detection of Maritime
Targets. In In Proc. Int. Workshop on Analysis and
Retrieval of Tracked Events and Motion in Imagery
Stream, pages 67–76, Barcelona, Spain.
Khan, S. H., Bennamoun, M., Sohel, F., and Togneri,
R. (2014). Automatic Feature Learning for Robust
Shadow Detection. In Proc. Conf. Comp. Vision and
Pattern Recognition, pages 1939–1946, Columbus,
Ohio, USA.
Leone, A. and Distante, C. (2007). Shadow detection for
moving objects based on texture analysis. Pattern
Recognition, 40(4):1222–1233.
Levin, A., Lischinski, D., and Weiss, Y. (2008). A Closed-
Form Solution to Natural Image Matting. Trans. Pat-
tern Analysis and Machine Intelligence, 30(2):228–
242.
Maddalena, L. and Petrosino, A. (2015). Towards Bench-
marking Scene Background Initialization. In Proc
Int. Conf. Image Analysis and Processing, volume
9281, pages 469–476.
Perazzi, F., Krahenbuhl, P., Pritch, Y., and Hornung,
A. (2012). Saliency filters: Contrast based filtering
for salient region detection. In Proc. Conf. Comp. Vi-
sion and Pattern Recognition, pages 733–740.
Pettersen, S. A., Halvorsen, P., Johansen, D., Johansen,
H., Berg-Johansen, V., Gaddam, V. R., Mortensen,
A., Langseth, R., Griwodz, C., and Stensland, H. K.
(2014). Soccer video and player position dataset. In
Proc. Multimedia Systems Conf., pages 18–23, New
York, USA.
Rijsbergen, C. J. V. (1979). Information Retrieval.
Butterworth-Heinemann, Newton, MA, USA, 2nd
edition.
Sanin, A., Sanderson, C., and Lovell, B. C. (2010). Im-
proved Shadow Removal for Robust Person Tracking
in Surveillance Scenarios. In Proc. Int. Conf. on Pat-
tern Recognition, pages 141–144.
Sanin, A., Sanderson, C., and Lovell, B. C. (2012). Shadow
detection: A survey and comparative evaluation of re-
cent methods. Pattern Recognition, 45(4):1684–1695.
Shahrian, E., Rajan, D., Price, B., and Cohen, S. (2013). Im-
proving Image Matting Using Comprehensive Sam-
pling Sets. In Proc. Conf. Computer Vision and Pat-
tern Recognition, pages 636–643.
Stone, J. E., Gohara, D., and Shi, G. (2010). OpenCL:
A Parallel Programming Standard for Heterogeneous
Computing Systems. Computing in Science & Engi-
neering, 12(3):66–73.
Wang, J. and Cohen, M. F. (2007). Image and Video Mat-
ting: A Survey. Foundations and Trends in Computer
Graphics and Vision, 3(2):97–175.
Yan, Q., Xu, L., Shi, J., and Jia, J. (2013). Hierarchical
saliency detection. In Proceedings of the IEEE Con-
ference on Computer Vision and Pattern Recognition,
pages 1155–1162.
Zivkovic, Z. (2004). Improved adaptive Gaussian mixture
model for background subtraction. In Proc. 17th In-
ternational Conf. on Pattern Recognition, volume 2,
pages 28–31 Vol.2, Washington, DC, USA.
Probabilistic Background Modelling for Sports Video Segmentation
525