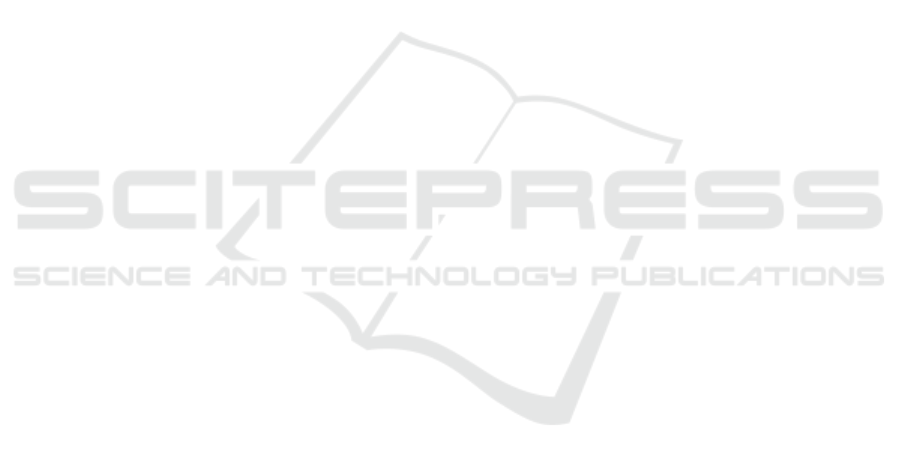
support vector machines. ACM Transactions on Intel-
ligent Systems and Technology (TIST), 2(3):27.
Kaltwang, S., Rudovic, O., and Pantic, M. (2012). Con-
tinuous pain intensity estimation from facial expres-
sions. In Advances in Visual Computing, pages 368–
377. Springer.
Khan, R. A., Meyer, A., Konik, H., and Bouakaz, S. (2013).
Pain detection through shape and appearance features.
In Multimedia and Expo (ICME), 2013 IEEE Interna-
tional Conference on, pages 1–6. IEEE.
Krig, S. (2014). Computer Vision Metrics: Survey, Taxon-
omy, and Analysis. Apress.
Lowe, D. G. (2004). Distinctive image features from scale-
invariant keypoints. International journal of computer
vision, 60(2):91–110.
Lucey, P., Cohn, J. F., Matthews, I., Lucey, S., Sridharan,
S., Howlett, J., and Prkachin, K. M. (2011a). Auto-
matically detecting pain in video through facial action
units. IEEE Transactions on Systems, Man, and Cy-
bernetics, Part B (Cybernetics), 41(3):664–674.
Lucey, P., Cohn, J. F., Prkachin, K. M., Solomon, P. E.,
Chew, S., and Matthews, I. (2012). Painful moni-
toring: Automatic pain monitoring using the unbc-
mcmaster shoulder pain expression archive database.
Image and Vision Computing, 30(3):197–205.
Lucey, P., Cohn, J. F., Prkachin, K. M., Solomon, P. E.,
and Matthews, I. (2011b). Painful data: The unbc-
mcmaster shoulder pain expression archive database.
In Automatic Face & Gesture Recognition and Work-
shops (FG 2011), 2011 IEEE International Confer-
ence on, pages 57–64. IEEE.
Neshov, N. and Manolova, A. (2015). Pain detection
from facial characteristics using supervised descent
method. In Intelligent Data Acquisition and Ad-
vanced Computing Systems: Technology and Appli-
cations (IDAACS), 2015 IEEE 8th International Con-
ference on, volume 1, pages 251–256. IEEE.
Ojala, T., Pietik
¨
ainen, M., and Harwood, D. (1996). A com-
parative study of texture measures with classification
based on featured distributions. Pattern recognition,
29(1):51–59.
Ojala, T., Pietik
¨
ainen, M., and M
¨
aenp
¨
a
¨
a, T. (2002). Mul-
tiresolution gray-scale and rotation invariant texture
classification with local binary patterns. Pattern Anal-
ysis and Machine Intelligence, IEEE Transactions on,
24(7):971–987.
Rathee, N. and Ganotra, D. (2015). A novel approach for
pain intensity detection based on facial feature defor-
mations. Journal of Visual Communication and Image
Representation, 33:247–254.
Roy, S. D., Bhowmik, M. K., Saha, P., and Ghosh, A. K.
(2016). An approach for automatic pain detection
through facial expression. Procedia Computer Sci-
ence, 84:99–106.
Scovanner, P., Ali, S., and Shah, M. (2007). A 3-
dimensional sift descriptor and its application to ac-
tion recognition. In Proceedings of the 15th inter-
national conference on Multimedia, pages 357–360.
ACM.
Vedaldi, A. and Fulkerson, B. (2010). Vlfeat: An open
and portable library of computer vision algorithms.
In Proceedings of the 18th ACM international confer-
ence on Multimedia, pages 1469–1472. ACM.
Zhao, G. and Pietikainen, M. (2007). Dynamic texture
recognition using local binary patterns with an appli-
cation to facial expressions. Pattern Analysis and Ma-
chine Intelligence, IEEE Transactions on, 29(6):915–
928.
HEALTHINF 2017 - 10th International Conference on Health Informatics
296