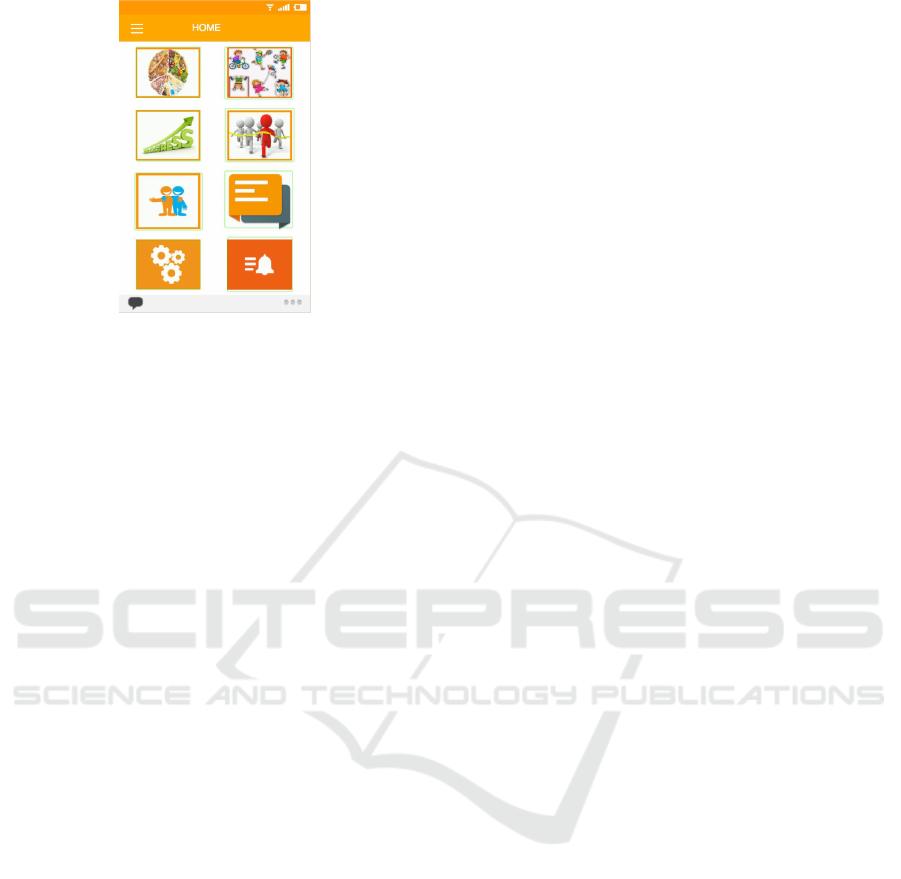
Figure 10: Home page for kids.
children and young persons (under 16) to reach a
healthier state with the supervision of healthcare prac-
titioners, families, and other users in a similar situa-
tion.
Along the paper, the system has been described,
and the first version of MATCHuP presented. Next
steps include the integration of smartwatches or sim-
ilar wearable able to automatically detect the physi-
cal activity, as well as artificial intelligence tools to
improve the aggregation methods and additional ad-
vices for further personalization and fast adaptation.
Moreover, a the evaluation of the tool in for medical
evidence is also required.
In that regard, the main challenge is to keep kids
engaged in the platform. Clinicians argue that about 6
month of using the platform could be sufficient for ob-
taining some behaviour change. However, some stud-
ies have shown that having a kid engaged in a game
more than 3 months is a great success. The long trial
of the tool will provide inputs to that concern, and
work for alternative artifaxts (Hevner et al., 2004) .
A secondary, technological challenge, is the fact that
sensitive data is stored in mobiles. The recent study
(Blenner et al., 2016) highlights the necessity of con-
sider privacy implications before using health apps.
ACKNOWLEDGEMENTS
This project has received funding from the grant of
the University of Girona 2016-2018 (MPCUdG2016),
ans has been developed with the support of
the research group SITES awarded with distinc-
tion by the Generalitat de Catalunya (SGR 2014-
2016). Sreynoch Soung received financial support
from the European Commission (Erasmus Mundus
project Techno II, ref. 372228-1-2012-1-FR-ERA
MUNDUS-EMA21).
REFERENCES
Ainsworth, B. E., Haskell, W. L., Herrmann, S. D., Meckes,
N., Bassett, D. R., Tudor-Locke, C., Greer, J. L., Vez-
ina, J., Whitt-Glover, M. C., and Leon, A. S. (2011).
2011 Compendium of Physical Activities: a second
update of codes and MET values. Medicine and sci-
ence in sports and exercise, 43(8):1575–81.
Ainsworth, B. E., Haskell, W. L., Leon, A. S., Jacobs, D. R.,
Montoye, H. J., Sallis, J. F., and Paffenbarger, R. S.
(1993). Compendium of physical activities: clas-
sification of energy costs of human physical activi-
ties. Medicine and science in sports and exercise,
25(1):71–80.
Aitker, M. (2015). Patient Adoption of mHealth. Use, Ev-
idence and Remainding Barriers to Mainstream Ac-
ceptance. Technical Report September.
Blenner, S. R., K
¨
ollmer, M., Rouse, A. J., Daneshvar,
N., Williams, C., Andrews, L. B., J, W., and AM,
B. (2016). Privacy Policies of Android Diabetes
Apps and Sharing of Health Information. JAMA,
315(10):1051.
Herrero, P., Lopez, B., and Martin, C. (2016). Pepper:
Patient empowerment through predictive personalised
decision support. In ECAI Workshop on Artificial In-
telligence for Diabetes, pages 8–9.
Hevner, A. R., March, S. T., Park, J., and Ram, S. (2004).
Design science in information systems research. MIS
Quarterly, 28(1):75–105.
L
´
opez, B., Coll, J., Gamero, F., BargallL
´
o, E., and L
´
opez-
Bermejo, A. (2013). Intelligent systems for support-
ing premature babies healthcare with mobile devices.
In Mobilemed, page 4 pages.
Sanders, L. M., Shaw, J. S., Guez, G., Baur, C., and Rudd,
R. (2009). Health literacy and child health promotion:
implications for research, clinical care, and public pol-
icy. Pediatrics, (124 Suplement 3):306–14.
Tate, E. B., Spruijt-Metz, D., O’Reilly, G., Jordan-Marsh,
M., Gotsis, M., Pentz, M. A., and Dunton, G. F.
(2013). mHealth approaches to child obesity preven-
tion: successes, unique challenges, and next direc-
tions. Translational behavioral medicine, 3(4):406–
15.
HEALTHINF 2017 - 10th International Conference on Health Informatics
318