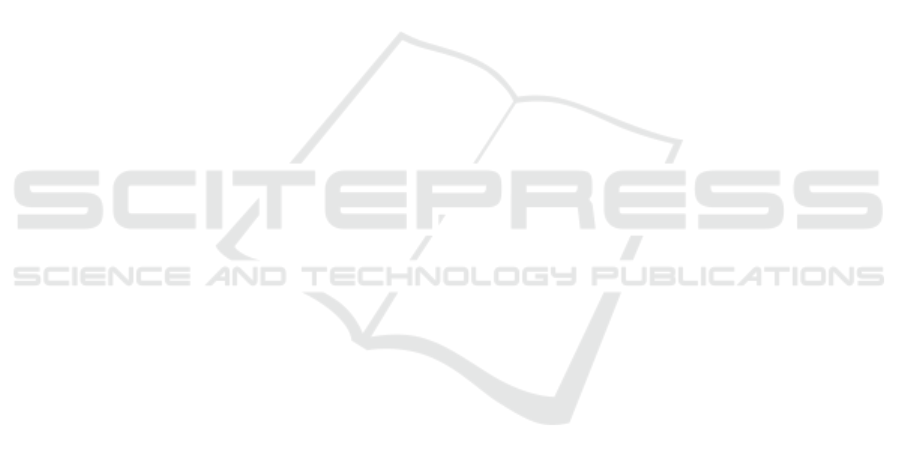
used for sleep period and vital sign recognition, kinect
sensors enable more accurate monitoring of walking
activity, and beacon sensors with smart phones allow
more precise understanding of outdoor activities.
New reasoning techniques are studied to corre-
late identified statistical changes with overall changes
in behavior toward better adaptation of provided ser-
vices; e.g., decrease in weight indicates negative nu-
tritional change and triggers sending of personalized
notifications to improve nutritional status.
ACKNOWLEDGEMENT
We give our special thanks to Saint Vincent de Paul
nursing home in Occagnes, France. Our deployment
in this nursing home is also supported by VHP in-
ter@ctive project and the Quality Of Life chair.
Our work is part of the European project City4Age
that received funding from the Horizon 2020 research
and innovation program under grant agreement num-
ber 689731.
REFERENCES
Allin, S., Bharucha, A., Zimmerman, J., Wilson, D., Robin-
son, M., Stevens, S., Wactlar, H., and Atkeson, C.
(2003). Toward the automatic assessment of behav-
ioral distrubances of dementia.
Aloulou, H. (2013). Framework for ambient assistive liv-
ing: handling dynamism and uncertainty in real time
semantic services provisioning. PhD thesis, Evry, In-
stitut national des t
´
el
´
ecommunications.
Aloulou, H., Mokhtari, M., Tiberghien, T., Biswas, J., Phua,
C., Lin, J. H. K., and Yap, P. (2013). Deployment of
assistive living technology in a nursing home environ-
ment: methods and lessons learned. BMC medical
informatics and decision making, 13(1):1.
Andersson, J. (2014). Locating multiple change-points us-
ing a combination of methods.
Avvenuti, M., Baker, C., Light, J., Tulpan, D., and Vec-
chio, A. (2010). Non-intrusive patient monitoring of
alzheimers disease subjects using wireless sensor net-
works. In 2009 World Congress on Privacy, Security,
Trust and the Management of e-Business.
Barberger-Gateau, P., Commenges, D., Gagnon, M., Leten-
neur, L., Sauvel, C., and Dartigues, J.-F. (1992). In-
strumental activities of daily living as a screening
tool for cognitive impairment and dementia in elderly
community dwellers. Journal of the American Geri-
atrics Society, 40(11):1129–1134.
Basseville, M., Nikiforov, I. V., et al. (1993). Detection of
abrupt changes: theory and application, volume 104.
Prentice Hall Englewood Cliffs.
Bland, J. M. and Altman, D. G. (1995). Comparing
methods of measurement: why plotting difference
against standard method is misleading. The lancet,
346(8982):1085–1087.
Cao, L. (2010). In-depth behavior understanding and use:
the behavior informatics approach. Information Sci-
ences, 180(17):3067–3085.
Cho, H. (2015). Change-point detection in panel data via
double cusum statistic.
City4Age (2016). Elderly-friendly city services
for active and healthy aging. available as
http://www.city4ageproject.eu/.
Cockrell, J. R. and Folstein, M. F. (2002). Mini-mental state
examination. Principles and practice of geriatric psy-
chiatry, pages 140–141.
Cummings, J. L., Mega, M., Gray, K., Rosenberg-
Thompson, S., Carusi, D. A., and Gornbein, J. (1994).
The neuropsychiatric inventory comprehensive as-
sessment of psychopathology in dementia. Neurology,
44(12):2308–2308.
Hayes, T. L., Abendroth, F., Adami, A., Pavel, M., Zitzel-
berger, T. A., and Kaye, J. A. (2008). Unobtru-
sive assessment of activity patterns associated with
mild cognitive impairment. Alzheimer’s & Dementia,
4(6):395–405.
Hayes, T. L., Larimer, N., Adami, A., and Kaye, J. A.
(2009). Medication adherence in healthy elders: small
cognitive changes make a big difference. Journal of
aging and health.
Kaye, J., Mattek, N., Dodge, H. H., Campbell, I., Hayes,
T., Austin, D., Hatt, W., Wild, K., Jimison, H., and
Pavel, M. (2014). Unobtrusive measurement of daily
computer use to detect mild cognitive impairment.
Alzheimer’s & Dementia, 10(1):10–17.
Kibria, G. (2016). Cumulative sum and exponen-
tially weighted moving average control charts.
preprint, available as http://www2.fiu.edu/ kibriag
/Stat5666/Handout/Chapter99.pdf.
Lafont, S., Barberger-Gateau, P., Sourgen, C., and Dar-
tigues, J. (1999). Relation entre performances cog-
nitives globales et d
´
ependance
´
evalu
´
ee par la grille
aggir. Revue d’
´
epid
´
emiologie et de sant
´
e publique,
47(1):7–17.
Lavikainen, H. M., Lintonen, T., and Kosunen, E. (2009).
Sexual behavior and drinking style among teenagers:
a population-based study in finland. Health promotion
international, 24(2):108–119.
Ledolter, J. and Kardon, R. (2013). Detecting the pro-
gression of eye disease: Cusum charts for assessing
the visual field and retinal nerve fiber layer thickness.
Translational vision science & technology, 2(6):2–2.
Liu, S., Yamada, M., Collier, N., and Sugiyama, M. (2013).
Change-point detection in time-series data by relative
density-ratio estimation. Neural Networks, 43:72–83.
Magill, E. and Blum, J. M. (2012). Personalised ambi-
ent monitoring: supporting mental health at home.
Advances in home care technologies: Results of the
Match project, pages 67–85.
Mathias, S., Nayak, U., and Isaacs, B. (1986). Balance in
elderly patients: the” get-up and go” test. Archives of
physical medicine and rehabilitation, 67(6):387–389.
Mesnil, B. and Petitgas, P. (2009). Detection of changes in
HEALTHINF 2017 - 10th International Conference on Health Informatics
104