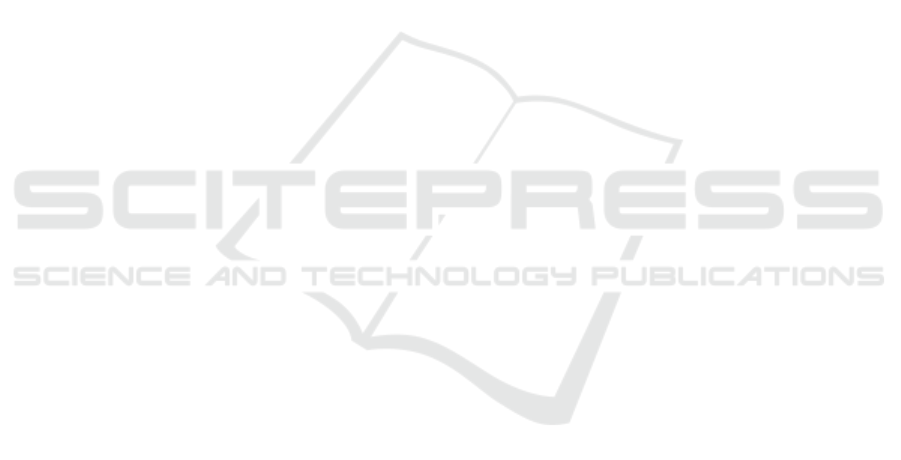
ACKNOWLEDGEMENT
We would like to acknowledge the support of the
Engineering and Physical Sciences Research Council
(EPSRC), grant references EP/K009931, EP/J015180
and a James Watt Scholarship.
REFERENCES
Ahmed, E., Jones, M., and Marks, T. K. (2015). An
improved deep learning architecture for person re-
identification. In The IEEE Conference on Computer
Vision and Pattern Recognition (CVPR), pages 3908–
3916.
Appel, R., Fuchs, T., Dollar, P., and Perona, P. (2013).
Quickly boosting decision trees – pruning under-
achieving features early. In ICML, volume 28, pages
594–602.
Bernardin, K. and Stiefelhagen, R. (2008). Evaluating mul-
tiple object tracking performance: The CLEAR MOT
metrics. J. Image Video Process., pages 1:1–1:10.
Bourgeois, F. and Lassalle, J.-C. (1971). An extension of
the munkres algorithm for the assignment problem to
rectangular matrices. Commun. ACM, 14(12):802–
804.
Cai, Y., de Freitas, N., and JJ, L. (2006). Robust visual
tracking for multiple targets. In IN ECCV, pages 107–
118.
Cham, T.-J. and Rehg, J. M. (1999). A multiple hypothesis
approach to figure tracking. In CVPR, pages 2239–
2245. IEEE Computer Society.
Choi, W. (2015). Near-online multi-target tracking with ag-
gregated local flow descriptor. In The IEEE Interna-
tional Conference on Computer Vision (ICCV).
Dollar, P., Appel, R., Perona, P., and Belongie, S. (2014).
Fast feature pyramids for object detection. IEEE
Transactions on Pattern Analysis and Machine Intel-
ligence, 99:14.
Liu, J. and Carr, P. (2014). Detecting and tracking sports
players with random forests and context-conditioned
motion models. In Computer Vision in Sports, pages
113–132. Springer.
Maggio, E., Taj, M., and Cavallaro, A. (2008). Efficient
multi-target visual tracking using random finite sets.
IEEE Transactions On Circuits And Systems For Video
Technology, pages 1016–1027.
Mahler, R. P. (2003). Multitarget bayes filtering via first-
order multitarget moments. IEEE Trans. on Aerospace
and Electronic Systems, 39(4):1152–1178.
Matzka, P., Wallace, A., and Petillot, Y. (2012). Efficient re-
source allocation for automotive attentive vision sys-
tems. IEEE Trans. on Intelligent Transportation Sys-
tems, 13(2):859–872.
Pasha, S., Vo, B.-N., Tuan, H. D., and Ma, W.-K. (2009).
A gaussian mixture PHD filter for jump markov sys-
tem models. Aerospace and Electronic Systems, IEEE
Transactions on, 45(3):919–936.
Rasmussen, C. and Hager, G. D. (2001). Probabilistic data
association methods for tracking complex visual ob-
jects. IEEE Transactions on Pattern Analysis and Ma-
chine Intelligence, 23:560–576.
Ristic, B., Clark, D., and Vo, B.-N. (2010). Improved SMC
implementation of the PHD filter. In Information Fu-
sion (FUSION), 2010 13th Conference on, pages 1–8.
Ristic, B., Clark, D. E., Vo, B.-N., and Vo, B.-T. (2012).
Adaptive target birth intensity for PHD and CPHD fil-
ters. IEEE Transactions on Aerospace and Electronic
Systems, 48(2):1656–1668.
Schumacher, D., Vo, B.-T., and Vo, B.-N. (2008). A con-
sistent metric for performance evaluation of multi-
object filters. Signal Processing, IEEE Transactions
on, 56(8):3447–3457.
Vo, B.-N. and Ma, W.-K. (2006). The Gaussian mixture
probability hypothesis density filter. Signal Process-
ing, IEEE Transactions on, 54(11):4091–4104.
Vo, B.-N., Singh, S., and Doucet, A. (2005). Sequential
monte carlo methods for multitarget filtering with ran-
dom finite sets. IEEE Transactions on Aerospace and
Electronic Systems, 41(4):1224–1245.
Wei, Y., Yaowen, F., Jianqian, L., and Xiang, L.
(2012). Joint detection, tracking, and classification
of multiple targets in clutter using the PHD filter.
Aerospace and Electronic Systems, IEEE Transac-
tions on, 48(4):3594–3609.
Yang, W., Fu, Y., and Li, X. (2014). Joint target tracking and
classification via RFS-based multiple model filtering.
Information Fusion, 18:101–106.
Yoon, J. H., Lee, C.-R., Yang, M.-H., and Yoon, K.-J.
(2016). Online multi-object tracking via structural
constraint event aggregation. In CVPR.
Zhou, X., Li, Y., He, B., and Bai, T. (2014). GM-PHD-
based multi-target visual tracking using entropy distri-
bution and game theory. Industrial Informatics, IEEE
Transactions on, 10(2):1064–1076.
Multiple Target, Multiple Type Visual Tracking using a Tri-GM-PHD Filter
477