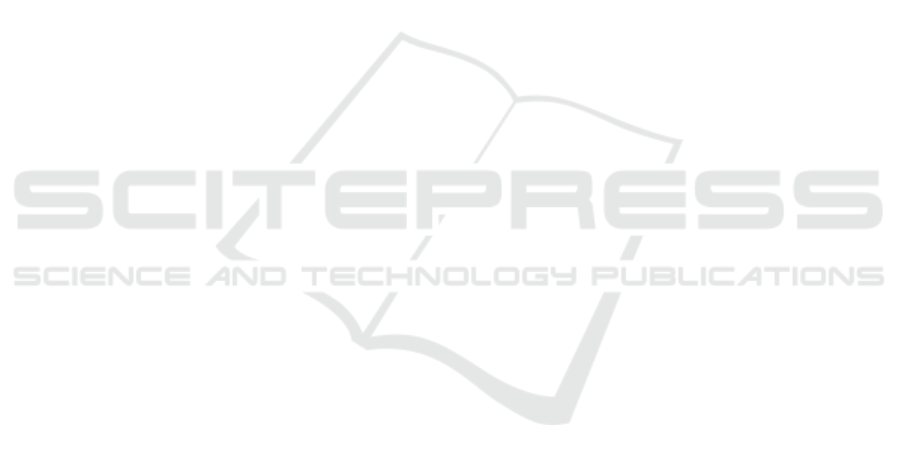
ACKNOWLEDGEMENT
This report is independent research funded by the
National Institute for Health Research Invention for
Innovation (i4i) programme, Enhanced, Personalized
and Integrated Care for Infection Management at
Point of Care (EPIC IMPOC), II-LA-0214-20008.
The authors would like to thank members of Impe-
rial College NHS Healthcare Trust who participated
in the study. The views expressed in this publication
are those of the authors and not necessarily those of
the NHS, the National Institute for Health Research
or the UK Department of Health.
REFERENCES
Aamodt, A. and Plaza, E. (1994). Case-based reasoning:
Foundational issues, methodological variations, and
system approaches. AI communications, 7(1):39–59.
Bangor, A., Kortum, P. T., and Miller, J. T. (2008). An
empirical evaluation of the system usability scale. Intl.
Journal of Human–Computer Interaction, 24(6):574–
594.
Belden, J. L., Grayson, R., and Barnes, J. (2009). Defin-
ing and testing emr usability: Principles and proposed
methods of emr usability evaluation and rating. Tech-
nical report, Healthcare Information and Management
Systems Society (HIMSS).
Boulos, M. N. K., Brewer, A. C., Karimkhani, C., Buller,
D. B., and Dellavalle, R. P. (2014). Mobile medical
and health apps: state of the art, concerns, regula-
tory control and certification. Online journal of public
health informatics, 5(3).
Brooke, J. et al. (1996). Sus-a quick and dirty usability
scale. Usability evaluation in industry, 189(194):4–7.
D
´
ıaz-Agudo, B., Gonz
´
alez-Calero, P. A., Recio-Garc
´
ıa,
J. A., and S
´
anchez-Ruiz-Granados, A. A. (2007).
Building cbr systems with jcolibri. Science of Com-
puter Programming, 69(1):68–75.
Flottorp, S., Oxman, A. D., H
˚
avelsrud, K., Treweek, S., and
Herrin, J. (2002). Cluster randomised controlled trial
of tailored interventions to improve the management
of urinary tract infections in women and sore throat.
Bmj, 325(7360):367.
Heindl, B., Schmidt, R., Schmid, G., Haller, M., Pfaller,
P., Gierl, L., and Pollwein, B. (1997). A case-based
consiliarius for therapy recommendation (icons):
computer-based advice for calculated antibiotic ther-
apy in intensive care medicine. Computer Methods
and Programs in Biomedicine, 52(2):117–127.
Holmes, A. H., Moore, L. S., Sundsfjord, A., Steinbakk,
M., Regmi, S., Karkey, A., Guerin, P. J., and Pid-
dock, L. J. (2016). Understanding the mechanisms
and drivers of antimicrobial resistance. The Lancet,
387(10014):176–187.
Jurisica, I. and Glasgow, J. (2004). Applications of case-
based reasoning in molecular biology. Ai Magazine,
25(1):85.
Kahn, C. E., Roberts, L. M., Shaffer, K. A., and Haddawy, P.
(1997). Construction of a bayesian network for mam-
mographic diagnosis of breast cancer. Computers in
biology and medicine, 27(1):19–29.
Kawamoto, K., Houlihan, C. A., Balas, E. A., and Lobach,
D. F. (2005). Improving clinical practice using clini-
cal decision support systems: a systematic review of
trials to identify features critical to success. BMJ,
330(7494):765.
Kofoed, K., Zalounina, A., Andersen, O., Lisby, G., Paul,
M., Leibovici, L., and Andreassen, S. (2009). Perfor-
mance of the treat decision support system in an envi-
ronment with a low prevalence of resistant pathogens.
Journal of antimicrobial chemotherapy, 63(2):400–
404.
Lucas, P., Schurink, C., Hoepelman, I., and Bonten, M.
(2003). A model-based approach to improved pre-
scription of antibiotics.
Lucas, P. J., van der Gaag, L. C., and Abu-Hanna, A. (2004).
Bayesian networks in biomedicine and health-care.
Artificial intelligence in medicine, 30(3):201–214.
McGregor, J. C., Weekes, E., Forrest, G. N., Standiford,
H. C., Perencevich, E. N., Furuno, J. P., and Harris,
A. D. (2006). Impact of a computerized clinical de-
cision support system on reducing inappropriate an-
timicrobial use: a randomized controlled trial. Jour-
nal of the American Medical Informatics Association,
13(4):378–384.
Moxey, A., Robertson, J., Newby, D., Hains, I., Williamson,
M., and Pearson, S.-A. (2010). Computerized clinical
decision support for prescribing: provision does not
guarantee uptake. Journal of the American Medical
Informatics Association, 17(1):25–33.
Mullett, C. J., Thomas, J. G., Smith, C. L., Sarwari, A. R.,
and Khakoo, R. A. (2004). Computerized antimi-
crobial decision support: an offline evaluation of a
database-driven empiric antimicrobial guidance pro-
gram in hospitalized patients with a bloodstream in-
fection. International journal of medical informatics,
73(5):455–460.
ONeill, J. (2014). Antimicrobial resistance: Tackling a cri-
sis for the health and wealth of nations.
Paul, M., Andreassen, S., Nielsen, A. D., Tacconelli, E.,
Almanasreh, N., Fraser, A., Yahav, D., Ram, R.,
Leibovici, L., Group, T. S., et al. (2006). Pre-
diction of bacteremia using treat, a computerized
decision-support system. Clinical Infectious Diseases,
42(9):1274–1282.
Pearl, J. (1988). Probabilistic inference in intelligent sys-
tems.
Quinlan, J. R. (1986). Induction of decision trees. Machine
Learning, 1(1):81–106.
Rawson, T., Charani, E., Moore, L. S., Hernandez, B.,
Castro-Sanchez, E., Herrero, P., Georgiou, P., and
Holmes, A. H. (2016a). Mapping the decision path-
ways of acute infection management in secondary care
among uk medical physicians: A qualitative study.
BMC medicine [In Press].
Rawson, T. M., Moore, L. S., Hernandez, B., Castro-
Sanchez, E., Charani, E., Georgiou, P., Ahmad, R.,
and Holmes, A. H. (2016b). Patient engagement with
HEALTHINF 2017 - 10th International Conference on Health Informatics
126