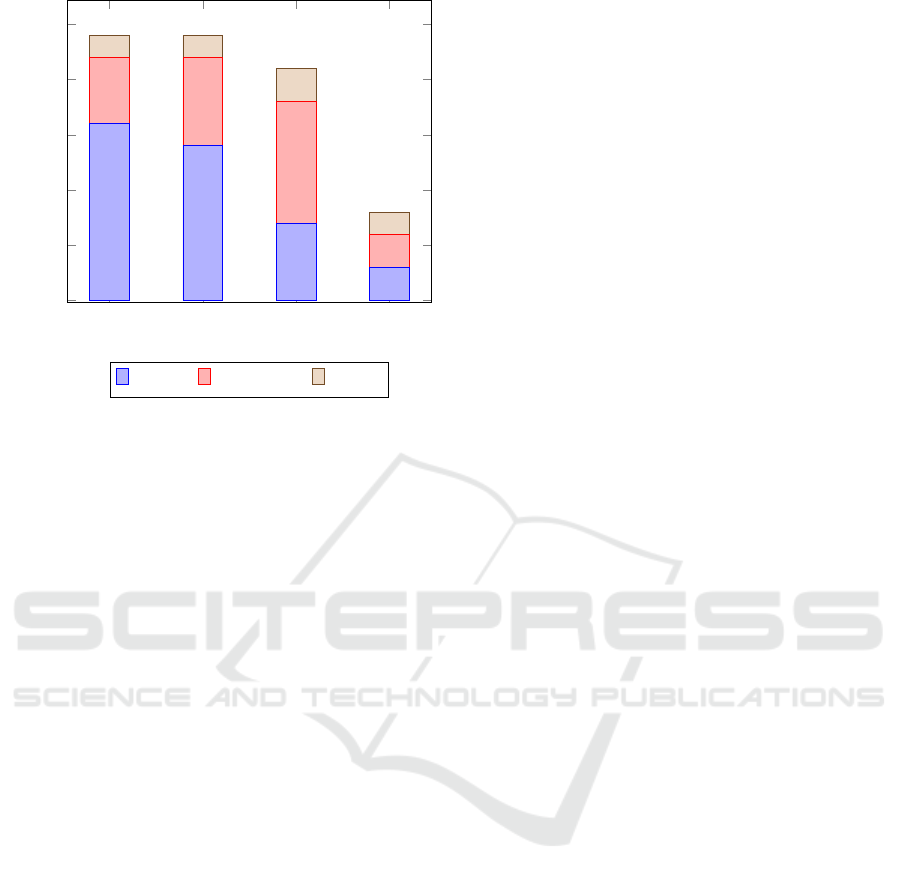
18-26 27-33 34-44 49-63
0
5
10
15
20
25
2 2
3
2
6
8
11
3
16
14
7
3
# of subjects
Normal Overweight Obese
Figure 1: Distribution of BMI groups over age groups.
5 CONCLUSION
This study provides some basis for the selection of
sampling rates and window sizes for human activity
recognition. The analysis indicates that 10s/50Hz is
statistically the best combination for data collected
with a single hip-worn accelerometer. Most of the ex-
periments carried out preferred larger windows and
high sampling rates though some low intensity ac-
tivities and demographics can perform better with
smaller windows. Our analysis further suggests that
window size can vary between 2-10 seconds and sam-
pling rate 25-100Hz for different situations without
a significant loss in performance. While our study
has shown that larger windows are preferable, smaller
windows can still provide significant results if power
consumption is an issue. Additionally, lower values
are preferable for studies involving the less dynamic
activities or subjects who are more liable to be less
active.
Future work in this field should be done to un-
derstand aspects of Human Activity Recognition bet-
ter. This study was performed under some assump-
tions that can be scrutinized. The placement of the
accelerometer could be shown to affect classifier per-
formance for different activities — a combination of
sensors can also be used. Other sensors, such as heart
rate monitors or video image processors, provide new
avenues. This study can also be replicated using dif-
ferent classifiers or learning methods with different
feature sets. Extensive analysis on the statistical value
of other machine learning and data mining methods
could also help the field as a whole.
REFERENCES
ActiGraph. Actisoft analysis software 3.2 user’s manual.
fort walton beach, fl: Mti health services.
Banos, O., Galvez, J.-M., Miguel Damas, H. P., and Ro-
jas, I. (2014). Window size impact in human activity
recognition. Sensors, pages 6474–6499.
Beiber, G., Voskamp, J., and Urban, B. (2009). Activity
recognition for everyday life on mobile phones. Inter-
national Conference on Universal Access in Human-
Computer Interaction, pages 289–296.
Demsar, J. (2006). Statistical comparisons of classifiers
over multiple data sets. Journal of Machine Learning
Research, 7:1–30.
Hall, M., Frank, E., Holmes, G., Pfahringer, B., Reutemann,
P., and Witten, I. H. (2009). The weka data min-
ing software: An update. SIGKDD Explor. Newsl.,
11(1):10–18.
Lara, O. D. and Labrador, M. A. (2013). A survey
on human activity recognition using wearable sen-
sors. IEEE Communication Surveys and Tutorials,
15:1192–1209.
Lau, S. and David, K. (2010). Movement recognition us-
ing the accelerometer in smartphones. IEEE Future
Network ands Mobile Summit 2010, pages 1–9.
Maurer, U., Smailagic, A., Siewiorek, D. P., and Deisher,
M. (2006). Activity recognition and monitoring using
multiple sensors on different body position. Interna-
tional Workshop on Wearable and Implantable Body
Sensor Networks.
Niazi, A., Yazdensepas, D., Gay, J., Maier, F., Rasheed, K.,
Ramaswamy, L., and Buman, M. (2016). A hierar-
chical meta-classifier for human activity recognition.
IEEE International Conference on Machine Learning
and Applications.
Preece, S. J., Goulermas, J. Y., Kenney, L. P. J., Howard,
D., Meijer, K., and Crompton, R. (2009). Activ-
ity identification using body-mounted sensorsa review
of classification techniques. Physiological Measure-
ment, 30(4):R1.
RStudio Team (2015). RStudio: Integrated Development
Environment for R. RStudio, Inc., Boston, MA.
Tapia, E., Intille, S., Haskell, W., Larson, K., Wright, J.,
King, A., and Friedman, R. (2007). Real-time recog-
nition of physical activities and their intensities us-
ing wireless accelerometers and a heart rate monitor.
IEEE 11th IEEE international symposium on wear-
able computers, pages 37–40.
Yazdansepas, D., Niazi, A. H., Gay, J. L., Maier, F. W., Ra-
maswamy, L., Rasheed, K., and Buman, M. P. (2016).
A multi-featured approach for wearable sensor-based
human activity recognition. IEEE International Con-
ference on Healthcare Informatics (ICHI), Chicago,
IL.
Statistical Analysis of Window Sizes and Sampling Rates in Human Activity Recognition
325