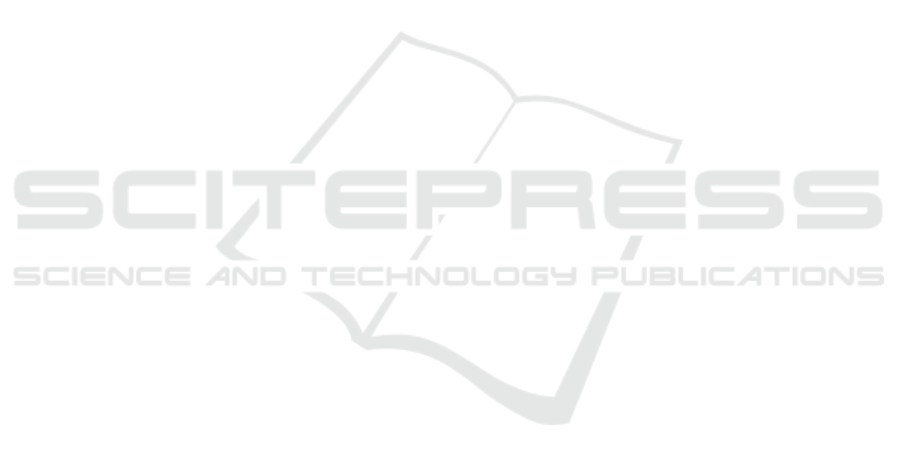
CONCLUSIONS
In this paper we have presented a new 3D lidar dataset
oriented to people detection in indoor environments.
We consider that the differences between scenes cover
a wide range of situations and problems that can oc-
cur in this kind of locations. In addition, we have
tested simple algorithms to demonstrate that these dif-
ferences are reflected in the classification and tracking
accuracy of each sequence.
The current dataset is complex and challenging
enough to test different people detection and tracking
algorithms. However, we plan to extend it in the near
future with new sequences in different large indoor
places (hallways and halls in different buildings).
ACKNOWLEDGEMENTS
This work has been partially funded by FEDER funds
and the Spanish Government (MICINN) through
projects TIN2013-46638-C3-3-P, TIN2015-65686-
C5-3-R, and DPI2013-40534-R and by Consejer
´
ıa
de Educaci
´
on, Cultura y Deportes of the JCCM re-
gional government through project PPII-2014-015-P.
Cristina Romero-Gonz
´
alez is funded by the MECD
grant FPU12/04387.
REFERENCES
Andriluka, M., Roth, S., and Schiele, B. (2008).
People-tracking-by-detection and people-detection-
by-tracking. In Computer Vision and Pattern Recog-
nition, 2008. CVPR 2008. IEEE Conference on, pages
1–8. IEEE.
Benenson, R., Omran, M., Hosang, J., and Schiele, B.
(2015). Ten Years of Pedestrian Detection, What Have
We Learned?, pages 613–627. Springer International
Publishing, Cham.
Bernardin, K. and Stiefelhagen, R. (2008). Evaluating mul-
tiple object tracking performance: The clear mot met-
rics. EURASIP Journal on Image and Video Process-
ing, 2008(1):1–10.
Blanco, J.-L., Moreno, F.-A., and Gonz
´
alez-Jim
´
enez, J.
(2014). The m
´
alaga urban dataset: High-rate stereo
and lidars in a realistic urban scenario. International
Journal of Robotics Research, 33(2):207–214.
Dollar, P., Wojek, C., Schiele, B., and Perona, P. (2012).
Pedestrian detection: An evaluation of the state of the
art. IEEE transactions on pattern analysis and ma-
chine intelligence, 34(4):743–761.
Everingham, M., Van Gool, L., Williams, C. K. I., Winn,
J., and Zisserman, A. (2010). The pascal visual ob-
ject classes (voc) challenge. International Journal of
Computer Vision, 88(2):303–338.
Geiger, A., Lenz, P., Stiller, C., and Urtasun, R. (2013).
Vision meets robotics: The kitti dataset. International
Journal of Robotics Research (IJRR).
Geronimo, D., Lopez, A. M., Sappa, A. D., and Graf, T.
(2010). Survey of pedestrian detection for advanced
driver assistance systems. IEEE Transactions on Pat-
tern Analysis and Machine Intelligence, 32(7):1239–
1258.
Leal-Taix
´
e, L., Milan, A., Reid, I., Roth, S., and Schindler,
K. (2015). MOTChallenge 2015: Towards a bench-
mark for multi-target tracking. arXiv:1504.01942
[cs]. arXiv: 1504.01942.
Mart
´
ınez-G
´
omez, J., Garc
´
ıa-Varea, I., Cazorla, M., and
Morell, V. (2015). Vidrilo: The visual and depth
robot indoor localization with objects information
dataset. The International Journal of Robotics Re-
search, 34(14):1681–1687.
Mu
˜
noz-Salinas, R., Aguirre, E., and Garc
´
ıa-Silvente, M.
(2007). People detection and tracking using stereo
vision and color. Image and Vision Computing,
25(6):995–1007.
Navarro-Serment, L. E., Mertz, C., and Hebert, M.
(2010). Pedestrian detection and tracking using three-
dimensional ladar data. The International Journal of
Robotics Research, 29(12):1516–1528.
Nguyen, D. T., Li, W., and Ogunbona, P. O. (2016). Human
detection from images and videos: A survey. Pattern
Recognition, 51:148–175.
Pandey, G., McBride, J. R., and Eustice, R. M. (2011). Ford
campus vision and lidar data set. The International
Journal of Robotics Research, 30(13):1543–1552.
Rusu, R. B. (2009). Semantic 3D Object Maps for Ev-
eryday Manipulation in Human Living Environments.
PhD thesis, Computer Science department, Technis-
che Universitaet Muenchen, Germany.
Rusu, R. B., Bradski, G., Thibaux, R., and Hsu, J. (2010).
Fast 3D recognition and pose using the viewpoint fea-
ture histogram. In Intelligent Robots and Systems
(IROS), 2010 IEEE/RSJ International Conference on,
pages 2155–2162. IEEE.
Rusu, R. B. and Cousins, S. (2011). 3D is here: Point Cloud
Library (PCL). In Robotics and Automation (ICRA),
2011 IEEE International Conference on, pages 1–4.
Smith, M., Baldwin, I., Churchill, W., Paul, R., and New-
man, P. (2009). The new college vision and laser data
set. The International Journal of Robotics Research,
28(5):595–599.
Spinello, L. and Arras, K. O. (2011). People detection in
rgb-d data. In 2011 IEEE/RSJ International Confer-
ence on Intelligent Robots and Systems, pages 3838–
3843. IEEE.
Wohlkinger, W. and Vincze, M. (2011). Ensemble of shape
functions for 3d object classification. In Robotics and
Biomimetics (ROBIO), 2011 IEEE International Con-
ference on, pages 2987–2992. IEEE.
InLiDa: A 3D Lidar Dataset for People Detection and Tracking in Indoor Environments
491