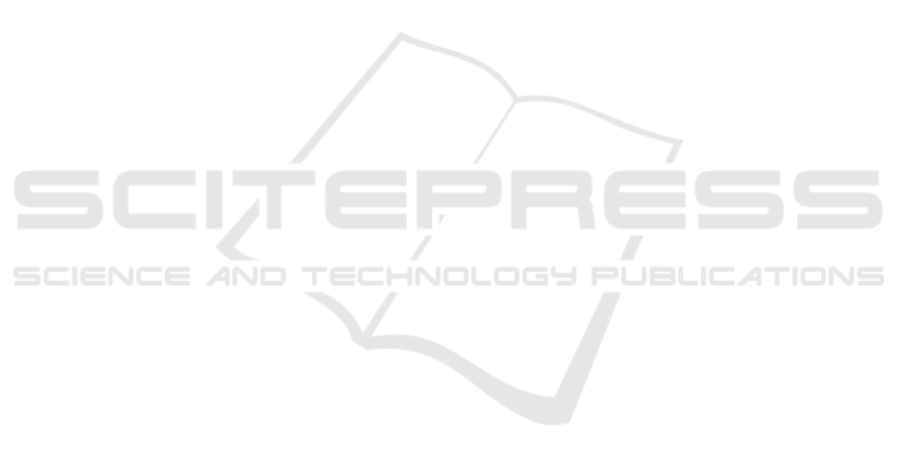
tion, but also it increases correspondingly the classifi-
cation speed.
4 CONCLUSIONS
The taxonomic classification of metagenomics reads
remains a crucial step in many metagenomics anal-
ysis. In this work we presented SKraken an ap-
proach based on filtering uninformative k-mers. We
compared the classification performances of SKraken
on several synthetic and real metagenomics datasets,
showing that SKraken achieves in most cases the
best performances in terms of precision and recall
w.r.t. Kraken. In particular the precision at species
level classification improves by 8%. In the estima-
tion of the abundance ratios in a metagenomic sample
SKraken obtains good results on all datasets. This be-
havior is confirmed also on a real stool metagenomic
sample where SKraken is able to detect species with
high precision. Another desirable property is that
SKraken requires less amount of RAM w.r.t. Kraken.
As future direction of investigation it would be inter-
esting to explore alternative definitions of k-mer qual-
ity incorporating other topological information of the
tree of life.
ACKNOWLEDGEMENTS
The authors would like to thank the anonymous re-
viewers for their valuable comments and suggestions.
This work was supported by the Italian MIUR project
PRIN20122F87B2.
REFERENCES
Ames, S. K., Hysom, D. A., Gardner, S. N., Lloyd, G. S.,
Gokhale, M. B., and Allen, J. E. (2013). Scalable
metagenomic taxonomy classification using a refer-
ence genome database. Bioinformatics, 29.
Antonello, M. and Comin, M. (2013). Fast Computation
of Entropic Profiles for the Detection of Conservation
in Genomes, pages 277–288. Springer Berlin Heidel-
berg, Berlin, Heidelberg.
Antonello, M. and Comin, M. (2014). Fast entropic pro-
filer: An information theoretic approach for the dis-
covery of patterns in genomes. IEEE/ACM Transac-
tions on Computational Biology and Bioinformatics,
11(3):500–509.
Antonello, M. and Comin, M. (2015). Fast alignment-
free comparison for regulatory sequences using mul-
tiple resolution entropic profiles. In Proceedings of
the International Conference on Bioinformatics Mod-
els, Methods and Algorithms (BIOSTEC 2015), pages
171–177.
Brown, C., Hug, L., Thomas, B., Sharon, I., Castelle, C.,
and Singh, A. e. a. (2015). Unusual biology across a
group comprising more than 15% of domain bacteria.
Nature, 523(7559):208–11.
Caporaso, J. G., Kuczynski, J., Stombaugh, J., Bittinger,
K., Bushman, F. D., Costello, E. K., Fierer, N., Pea,
A. G., Goodrich, J. K., Gordon, J. I., Huttley, G. A.,
Kelley, S. T., Knights, D., Koenig, J. E., Ley, R. E.,
Lozupone, C. A., McDonald, D., Muegge, B. D., Pir-
rung, M., Reeder, J., Sevinsky, J. R., Turnbaugh, P. J.,
Walters, W. A., Widmann, J., Yatsunenko, T., Zan-
eveld, J., and Knight, R. (2010). Qiime allows anal-
ysis of high-throughput community sequencing data.
Nature methods, 7(5):335336.
Comin, M., Leoni, A., and Schimd, M. (2015). Clustering
of reads with alignment-free measures and quality val-
ues. Algorithms for Molecular Biology, 10(1):1–10.
Comin, M. and Schimd, M. (2014). Assembly-free
genome comparison based on next-generation se-
quencing reads and variable length patterns. BMC
Bioinformatics, 15(9):1–10.
Comin, M. and Verzotto, D. (2012). Whole-genome phy-
logeny by virtue of unic subwords. In Database and
Expert Systems Applications (DEXA), 2012 23rd In-
ternational Workshop on, pages 190–194.
Comin, M. and Verzotto, D. (2014). Beyond fixed-
resolution alignment-free measures for mammalian
enhancers sequence comparison. IEEE/ACM Trans-
actions on Computational Biology and Bioinformat-
ics, 11(4):628–637.
Consortium, H. M. P. (2012). Structure, function and di-
versity of the healthy human microbiome. Nature,
486(7402):207–214.
Felczykowska, A., Bloch, S. K., Nejman-Faleczyk, B.,
and Baraska, S. (2012). Metagenomic approach
in the investigation of new bioactive compounds in
the marine environment. Acta Biochimica Polonica,
59(4):501505.
Girotto, S., Pizzi, C., and Comin, M. (2016). Metaprob:
accurate metagenomic reads binning based on
probabilistic sequence signatures. Bioinformatics,
32(17):i567–i575.
Goke, J., Schulz, M. H., Lasserre, J., and Vingron, M.
(2012). Estimation of pairwise sequence similarity
of mammalian enhancers with word neighbourhood
counts. Bioinformatics, 28(5):656–663.
Huson, D. H., Auch, A. F., Qi, J., and Schuster, S. C. (2007).
Megan analysis of metagenomic data. Genome Res.,
17.
Kantorovitz, M. R., Robinson, G. E., and Sinha, S. (2007).
A statistical method for alignment-free comparison of
regulatory sequences. Bioinformatics., 23.
Lindgreen, S., Adair, K. L., and Gardner, P. (2016). An
evaluation of the accuracy and speed of metagenome
analysis tools. Scientific Reports, 6:19233.
Liu, B., Gibbons, T., Ghodsi, M., Treangen, T., and Pop,
M. (2011). Accurate and fast estimation of taxonomic
BIOINFORMATICS 2017 - 8th International Conference on Bioinformatics Models, Methods and Algorithms
66