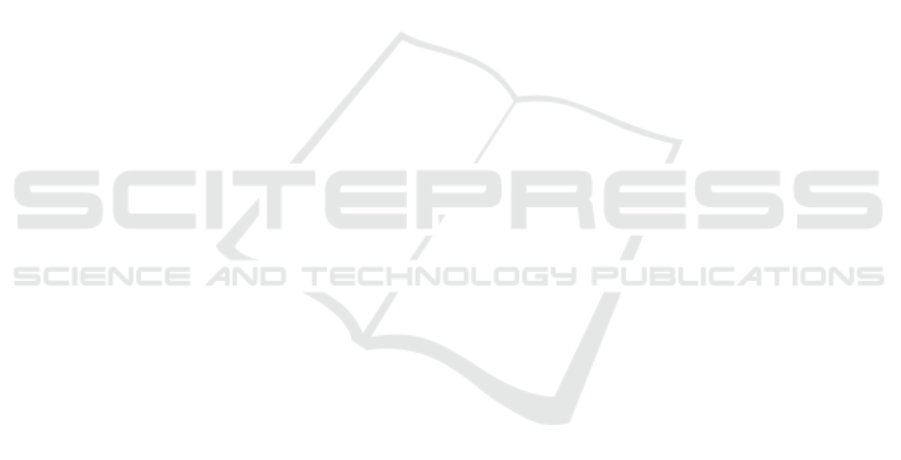
Moreau, Y. (2012). An unbiased evaluation of gene
prioritization tools. Bioinformatics, 28(23):3081–
3088.
Bourgon, R., Gentleman, R., and Huber, W. (2010). Inde-
pendent filtering increases detection power for high-
throughput experiments. Proceedings of the National
Academy of Sciences of the United States of America,
107:9546–9551.
Cancer, T. and Atlas, G. (2012). Comprehensive molecu-
lar characterization of human colon and rectal cancer.
Nature, 487(7407):330–7.
Carvalho, B. S. and Irizarry, R. a. (2010). A framework for
oligonucleotide microarray preprocessing. Bioinfor-
matics, 26(19):2363–2367.
Cattaneo, E., Laczko, E., Buffoli, F., Zorzi, F., Bianco,
M. A., Menigatti, M., Bartosova, Z., Haider, R.,
Helmchen, B., Sabates-Bellver, J., Tiwari, A., Jiricny,
J., and Marra, G. (2011). Preinvasive colorectal le-
sion transcriptomes correlate with endoscopic mor-
phology (polypoid vs. nonpolypoid). EMBO molec-
ular medicine, 3(6):334–47.
de Klerk, E. and a.C. t Hoen, P. (2015). Alternative
mRNA transcription, processing, and translation: in-
sights from RNA sequencing. Trends in Genetics,
31(3):128–139.
Dudoit, S., Dudoit, S., Shaffer, J. P., Shaffer, J. P., Boldrick,
J. C., and Boldrick, J. C. (2003). Multiple hypothesis
testing in microarray experiments. Statistical Science,
18(1):71–103.
Foss, E. J., Radulovic, D., Shaffer, S. a., Ruderfer, D. M.,
Bedalov, A., Goodlett, D. R., and Kruglyak, L. (2007).
Genetic basis of proteome variation in yeast. Nature
genetics, 39(11):1369–1375.
Geiger, T., Wehner, a., Schaab, C., Cox, J., and Mann, M.
(2012). Comparative Proteomic Analysis of Eleven
Common Cell Lines Reveals Ubiquitous but Varying
Expression of Most Proteins. Molecular & Cellular
Proteomics, 11:M111.014050–M111.014050.
Gygi, S. P., Rochon, Y., Franza, B. R., and Aebersold,
R. (1999). Correlation between protein and mRNA
abundance in yeast. Molecular and cellular biology,
19(3):1720–1730.
Haider, S. and Pal, R. (2013). Integrated analysis of tran-
scriptomic and proteomic data. Current genomics,
14(2):91–110.
Kanehisa, M., Goto, S., Sato, Y., Kawashima, M., Furu-
michi, M., and Tanabe, M. (2014). Data, informa-
tion, knowledge and principle: Back to metabolism in
KEGG. Nucleic Acids Research, 42(D1):199–205.
Kelder, T., Pico, A. R., Hanspers, K., Van Iersel, M. P.,
Evelo, C., and Conklin, B. R. (2009). Mining biologi-
cal pathways using WikiPathways web services. PLoS
ONE, 4(7):2–5.
Kumar, D., Bansal, G., Narang, A., Basak, T., Abbas, T.,
and Dash, D. (2016). Integrating transcriptome and
proteome profiling: Strategies and applications. Pro-
teomics, pages 1–12.
Kuo, T.-C., Tian, T.-F., and Tseng, Y. J. (2013). 3Omics: a
web-based systems biology tool for analysis, integra-
tion and visualization of human transcriptomic, pro-
teomic and metabolomic data. BMC systems biology,
7(1):64–78.
Larance, M. and Lamond, A. I. (2015). Multidimensional
proteomics for cell biology. Nature Reviews Molecu-
lar Cell Biology, 16(5):268–80.
Luo, B. and Lee, a. S. (2012). The critical roles of en-
doplasmic reticulum chaperones and unfolded protein
response in tumorigenesis and anticancer therapies.
Oncogene, 32(7):805–818.
Matys, V., Kel-Margoulis, O. V., Fricke, E., Liebich, I.,
Land, S., Barre-Dirrie, A., Reuter, I., Chekmenev,
D., Krull, M., Hornischer, K., Voss, N., Stegmaier,
P., Lewicki-Potapov, B., Saxel, H., Kel, a. E., and
Wingender, E. (2006). TRANSFAC and its mod-
ule TRANSCompel: transcriptional gene regulation
in eukaryotes. Nucleic acids research, 34(Database
issue):D108–D110.
Neely, B. A., Wilkins, C. E., Marlow, L. A., Malyarenko,
D., Kim, Y., Ignatchenko, A., Sasinowska, H., Sasi-
nowski, M., Nyalwidhe, J. O., Kislinger, T., Cop-
land, J. A., and Drake, R. R. (2016). Proteotranscrip-
tomic Analysis Reveals Stage Specific Changes in the
Molecular Landscape of Clear-Cell Renal Cell Carci-
noma. PloS one, 11(4):e0154074.
Pirhaji, L., Milani, P., Leidl, M., Curran, T., Avila-
Pacheco, J., Clish, C. B., White, F. M., Saghatelian,
A., and Fraenkel, E. (2016). Revealing disease-
associated pathways by network integration of untar-
geted metabolomics. Nature methods, 13(9):770–776.
Piruzian, E., Bruskin, S., Ishkin, A., Abdeev, R.,
Moshkovskii, S., Melnik, S., Nikolsky, Y., and Nikol-
skaya, T. (2010). Integrated network analysis of tran-
scriptomic and proteomic data in psoriasis. BMC sys-
tems biology, 4(41).
Polpitiya, A. D., Qian, W. J., Jaitly, N., Petyuk, V. a.,
Adkins, J. N., Camp, D. G., Anderson, G. a., and
Smith, R. D. (2008). DAnTE: A statistical tool for
quantitative analysis of -omics data. Bioinformatics,
24(13):1556–1558.
Robinson, M. D. and Smyth, G. K. (2007). Mod-
erated statistical tests for assessing differences in
tag abundance. Bioinformatics (Oxford, England),
23(21):2881–7.
Shimwell, N. J., Bryan, R. T., Wei, W., James, N. D.,
Cheng, K. K., Zeegers, M. P., Johnson, P. J., Martin,
a., and Ward, D. G. (2013). Combined proteome and
transcriptome analyses for the discovery of urinary
biomarkers for urothelial carcinoma. British journal
of cancer, 108(9):1854–61.
Smyth, G. K. (2004). Linear Models and Empirical Bayes
Methods for Assessing Differential Expression in Mi-
croarray Experiments Linear Models and Empirical
Bayes Methods for Assessing Differential Expression
in Microarray Experiments. Statistical applications in
genetics and molecular biology, 3(1):1–26.
Talloen, W., Clevert, D.-A., Hochreiter, S., Amaratunga, D.,
Bijnens, L., Kass, S., and G
¨
ohlmann, H. W. H. (2007).
I/NI-calls for the exclusion of non-informative genes:
a highly effective filtering tool for microarray data.
Bioinformatics (Oxford, England), 23(21):2897–902.
Complementary Domain Prioritization: A Method to Improve Biologically Relevant Detection in Multi-Omic Data Sets
79