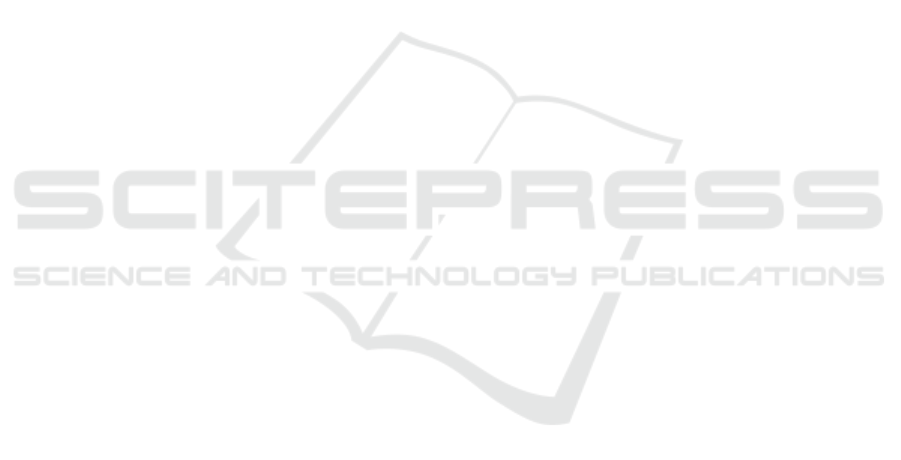
Li, J., Wang, Z. J., Palmer, S. J., and McKeown, M. J.
(2008). Dynamic bayesian network modeling of fmri:
a comparison of group-analysis methods. Neuroim-
age, 41(2):398–407.
Li, R., Wu, X., Chen, K., Fleisher, A., Reiman, E., and
Yao, L. (2013). Alterations of directional connectiv-
ity among resting-state networks in alzheimer disease.
American Journal of Neuroradiology, 34(2):340–345.
Libby, L. A., Ekstrom, A. D., Ragland, J. D., and
Ranganath, C. (2012). Differential connectivity
of perirhinal and parahippocampal cortices within
human hippocampal subregions revealed by high-
resolution functional imaging. The Journal of Neu-
roscience, 32(19):6550–6560.
Maruszak, A. and Thuret, S. (2015). Why looking at the
whole hippocampus is not enougha critical role for
anteroposterior axis, subfield and activation analyses
to enhance predictive value of hippocampal changes
for alzheimers disease diagnosis. 2015: Which new
directions for Alzheimer’s disease?, page 6.
Mumford, J. A. and Ramsey, J. D. (2014). Bayesian net-
works for fmri: a primer. Neuroimage, 86:573–582.
Neapolitan, R. E. et al. (2004). Learning bayesian networks.
Patel, R. S., Bowman, F. D., and Rilling, J. K. (2006). A
bayesian approach to determining connectivity of the
human brain. Human brain mapping, 27(3):267–276.
Penny, W. D., Friston, K. J., Ashburner, J. T., Kiebel, S. J.,
and Nichols, T. E. (2011). Statistical parametric map-
ping: the analysis of functional brain images. Aca-
demic press.
Rajapakse, J. C. and Zhou, J. (2007). Learning effective
brain connectivity with dynamic bayesian networks.
Neuroimage, 37(3):749–760.
Rykhlevskaia, E., Gratton, G., and Fabiani, M. (2008).
Combining structural and functional neuroimaging
data for studying brain connectivity: a review. Psy-
chophysiology, 45(2):173–187.
Santos, F. P. and Maciel, C. D. (2014). A pso approach for
learning transition structures of higher-order dynamic
bayesian networks. In Biosignals and Biorobotics
Conference (2014): Biosignals and Robotics for Bet-
ter and Safer Living (BRC), 5th ISSNIP-IEEE, pages
1–6.
Schulz, M., Chau, W., Graham, S. J., McIntosh, A. R., Ross,
B., Ishii, R., and Pantev, C. (2004). An integrative
meg–fmri study of the primary somatosensory cortex
using cross-modal correspondence analysis. NeuroIm-
age, 22(1):120–133.
Smith, S. M., Miller, K. L., Salimi-Khorshidi, G., Web-
ster, M., Beckmann, C. F., Nichols, T. E., Ramsey,
J. D., and Woolrich, M. W. (2011). Network mod-
elling methods for fmri. Neuroimage, 54(2):875–891.
Smith, V. A., Yu, J., Smulders, T. V., Hartemink, A. J., and
Jarvis, E. D. (2006). Computational inference of neu-
ral information flow networks. PLoS computational
biology, 2(11):e161.
Valdes-Sosa, P. A., Roebroeck, A., Daunizeau, J., and Fris-
ton, K. (2011). Effective connectivity: influence,
causality and biophysical modeling. Neuroimage,
58(2):339–361.
Vinh, N. X., Chetty, M., Coppel, R., and Wangikar, P. P.
(2011a). Globalmit: learning globally optimal dy-
namic bayesian network with the mutual information
test criterion. Bioinformatics, 27(19):2765–2766.
Vinh, N. X., Chetty, M., Coppel, R., and Wangikar, P. P.
(2011b). Polynomial time algorithm for learning glob-
ally optimal dynamic bayesian network. In Interna-
tional Conference on Neural Information Processing,
pages 719–729. Springer.
Wang, C., Xu, J., Zhao, S., and Lou, W. (2015). Graph the-
oretical analysis of eeg effective connectivity in vas-
cular dementia patients during a visual oddball task.
Clinical Neurophysiology.
Wisse, L., Kuijf, H., Honingh, A., Wang, H., Pluta, J.,
Das, S., Wolk, D., Zwanenburg, J., Yushkevich, P.,
and Geerlings, M. (2016). Automated hippocampal
subfield segmentation at 7t mri. American Journal of
Neuroradiology.
Wisse, L. E., Biessels, G. J., Heringa, S. M., Kuijf, H. J.,
Luijten, P. R., Geerlings, M. I., Group, U. V. C. I.
V. S., et al. (2014). Hippocampal subfield volumes
at 7t in early alzheimer’s disease and normal aging.
Neurobiology of aging, 35(9):2039–2045.
Wu, X., Wen, X., Li, J., and Yao, L. (2014). A new dynamic
bayesian network approach for determining effective
connectivity from fmri data. Neural Computing and
Applications, 24(1):91–97.
Xuan, N., Chetty, M., Coppel, R., and Wangikar, P. P.
(2012). Gene regulatory network modeling via global
optimization of high-order dynamic bayesian net-
work. BMC bioinformatics, 13(1):131.
Yushkevich, P. A., Pluta, J. B., Wang, H., Xie, L., Ding, S.-
L., Gertje, E. C., Mancuso, L., Kliot, D., Das, S. R.,
and Wolk, D. A. (2015). Automated volumetry and
regional thickness analysis of hippocampal subfields
and medial temporal cortical structures in mild cogni-
tive impairment. Human brain mapping, 36(1):258–
287.
Zhang, L., Samaras, D., Alia-Klein, N., Volkow, N., and
Goldstein, R. (2005). Modeling neuronal interactiv-
ity using dynamic bayesian networks. In Advances in
neural information processing systems, pages 1593–
1600.
Zhu, D., Zhang, T., Jiang, X., Hu, X., Chen, H., Yang, N.,
Lv, J., Han, J., Guo, L., and Liu, T. (2014). Fusing dti
and fmri data: a survey of methods and applications.
Neuroimage, 102:184–191.
BIOSIGNALS 2017 - 10th International Conference on Bio-inspired Systems and Signal Processing
184