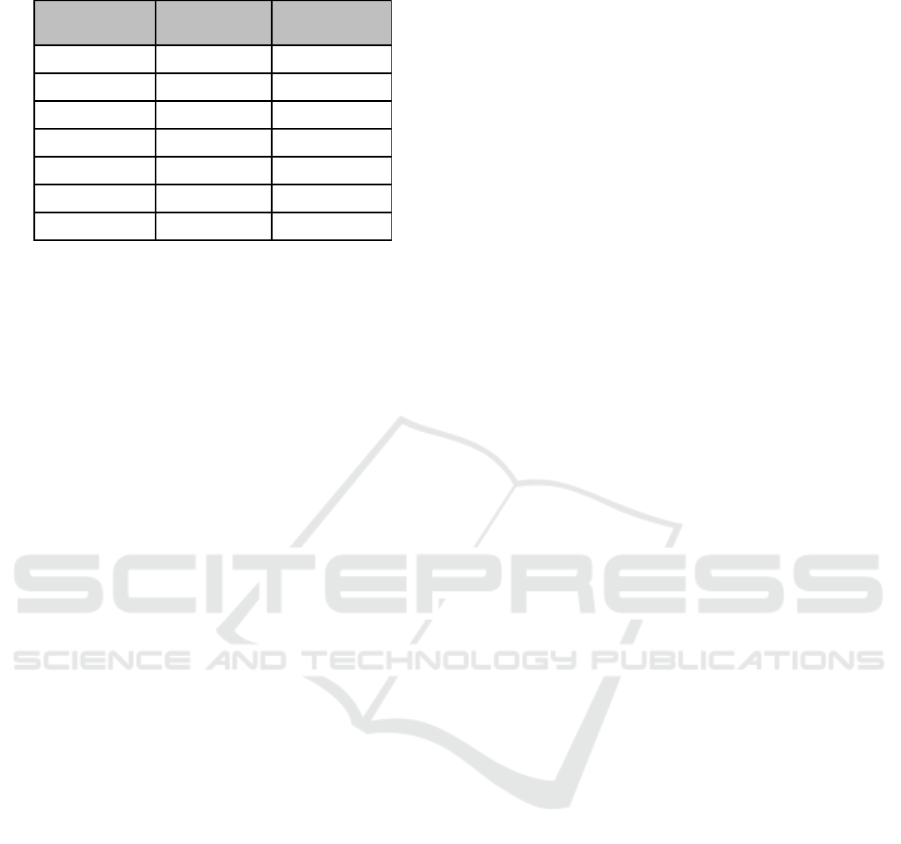
Table 2: Pathway categorized by regulation direction across
two FLI1 expression studies.
Gain-of-
Function FLI1
Loss-of-
Function
Pathways
153
64
69
33
35
35
33
lections represent the possible regulation patterns for
genes affected by both conditions. Each subset, as
well as the full dataset, were subjected to pathway
analysis using iPathwayGuide (Draghici et al., 2007).
Each subset is labeled with its significant (α = 0.05)
pathways (Figure 4).
The top 100 pathways for genes up-regulated in
GOF, down-regulated in GOF, and so on for LOF
were identified and similarly categorized in Table 2.
These tables demonstrate how subsets of genes may
translate into subsets of pathways. For example, of
the original 400 pathways, 33 (8.25%) involve genes
up-regulated in GOF and down-regulated in LOF.
Closer investigation is necessary to determine if each
pathway as a whole was up- or down-regulated. Path-
ways that are up-regulated in one condition and down-
regulated by the other are often of particular interest,
as these may indicate which cellular processes are di-
rectly correlated with a condition.
4 DISCUSSION
DEACT’s interactive user interface for rapid visual-
ization and categorization of expression data is intu-
itive for researchers with little or no programming ex-
perience. It supports any two complementary studies
and can compare biological replicates or contrasting
experimental conditions. DEACT automatically cat-
egorizes significant data points by their response to
each condition. These practically relevant categories
may be downloaded as subsets for further study, for
example, in a file format accepted by iPathwayGuide
and other programs. Alternatively, unique subsets can
be selected directly from the user interface. The re-
sponsive user interface allows users to instantly iden-
tify and select any set of genes, thus achieving a level
of engagement that neither scripts nor traditional plots
offer.
The simple, interactive design makes DEACT an
effective collaboration tool for research laboratories.
Unlike biclustering, DEACT can be effectively used
to interpret small datasets and does not require the
additional time or training required to optimize a bi-
clustering algorithm. Instead, it is built to quickly an-
swer preliminary questions about new RNA-seq data
to prompt downstream analyses and encourage a flow
of discussion. With DEACT, researchers may easily
create highly customizable datasets to fit any ques-
tion, however specific. In the future, pathway analysis
may be integrated with DEACT in order to visualize
cellular responses on the pathway level as well as the
gene level. This feature may be incorporated into the
user interface, as shown in Figure 3.
DEACT adds to a growing set of meta-analytical
tools for RNA-Seq data. With a tool like DEACT,
non-computationally trained researchers can mine
their data for novel insights on gene expression and
function. Such meta-analyses not only augment our
understanding of cellular processes, but they have the
potential to lead to novel life-saving therapeutics.
ACKNOWLEDGEMENTS
The authors would like to thank the College of
Charleston for hosting the NSF Omics REU which
is supervised by the National Science Foundation
DBI Award 1359301. We also acknowledge sup-
port from the Genomics Shared Resource, Hollings
Cancer Center, Medical University of South Car-
olina. This shared resource is supported in part by the
Hollings Cancer Center, Medical University of South
Carolina Support Grant (P30 CA 138313).
REFERENCES
Bioinformatics, B. (2011). Fastqc a quality control tool
for high throughput sequence data. Cambridge, UK:
Babraham Institute.
Bolger, A. and Giorgi, F. (2014). Trimmomatic: a flex-
ible read trimming tool for illumina ngs data. URL
http://www. usadellab. org/cms/index. php.
Draghici, S., Khatri, P., Tarca, A. L., Amin, K., Done, A.,
Voichita, C., Georgescu, C., and Romero, R. (2007).
A systems biology approach for pathway level analy-
sis. Genome research, 17(10):1537–1545.
Love, M. I., Huber, W., and Anders, S. (2014). Moderated
estimation of fold change and dispersion for rna-seq
data with deseq2. Genome biology, 15(12):1.
Luo, W. and Brouwer, C. (2013). Pathview: an
r/bioconductor package for pathway-based data
integration and visualization. Bioinformatics,
29(14):1830–1831.
BIOINFORMATICS 2017 - 8th International Conference on Bioinformatics Models, Methods and Algorithms
158