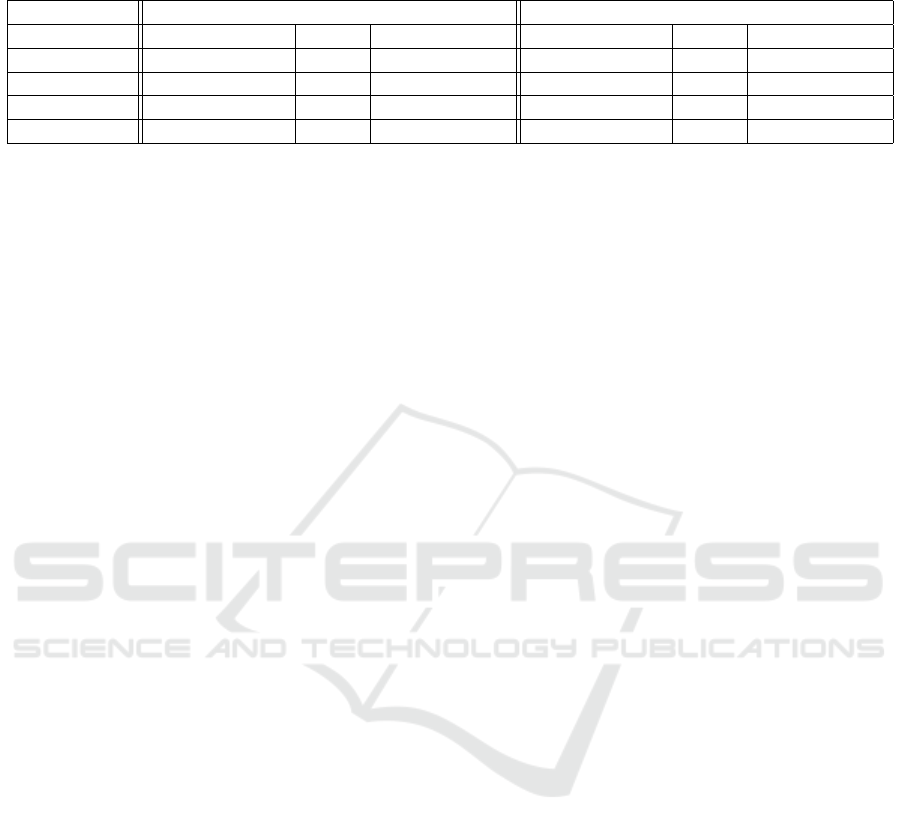
Table 3: Re-identification results of the BIWI methods in (Munaro et al., 2014b) and our volume-based (VB) approach on the
BIWI RGBD-ID dataset.
Still Walking
Single (Rank-1) nAUC Multi (Rank-1) Single (Rank-1) nAUC Multi (Rank-1)
BIWI (SVM) 11.60 84.50 10.70 13.80 81.70 17.90
BIWI (NN) 26.60 89.70 32.10 21.10 86.60 39.30
VB 12.74 73.91 17.86 6.88 71.24 17.86
VB-Skel. 32.12 91.79 42.86 18.93 82.66 42.86
REFERENCES
Barbosa, I. B., Cristani, M., Del Bue, A., Bazzani, L., and
Murino, V. (2012). Re-identification with rgb-d sen-
sors. In European Conference on Computer Vision,
pages 433–442. Springer.
Bedagkar-Gala, A. and Shah, S. K. (2014). A survey of ap-
proaches and trends in person re-identification. Image
and Vision Computing, 32(4):270 – 286.
Bellotto, N. and Hu, H. (2010). A bank of unscented
kalman filters for multimodal human perception with
mobile service robots. International Journal of Social
Robotics, 2(2):121–136.
Chen, D., Yuan, Z., Hua, G., Zheng, N., and Wang, J.
(2015). Similarity learning on an explicit polyno-
mial kernel feature map for person re-identification. In
2015 IEEE Conference on Computer Vision and Pat-
tern Recognition (CVPR), pages 1565–1573.
Cortes, C. and Vapnik, V. (1995). Support-vector networks.
Machine Learning, 20(3):273–297.
Farenzena, M., Bazzani, L., Perina, A., Murino, V., and
Cristani, M. (2010). Person re-identification by
symmetry-driven accumulation of local features. In
Computer Vision and Pattern Recognition (CVPR),
2010 IEEE Conference on, pages 2360–2367.
Ferland, F., Cruz-Maya, A., and Tapus, A. (2015). Adapting
an hybrid behavior-based architecture with episodic
memory to different humanoid robots. In Robot and
Human Interactive Communication (RO-MAN), 2015
24th IEEE International Symposium on, pages 797–
802.
Gordon, C. C., Churchill, T., Clauser, C. E., Bradtmiller, B.,
and McConville, J. T. (1989). Anthropometric survey
of US Army personnel: Summary statistics, interim
report for 1988. Technical report, DTIC Document.
Koide, K. and Miura, J. (2016). Identification of a spe-
cific person using color, height, and gait features for
a person following robot. Robotics and Autonomous
Systems, 84:76 – 87.
Kviatkovsky, I., Adam, A., and Rivlin, E. (2013). Color in-
variants for person reidentification. IEEE Trans. Pat-
tern Anal. Mach. Intell., 35(7):1622–1634.
Li, W., Zhao, R., Xiao, T., and Wang, X. (2014). Deep-
reid: Deep filter pairing neural network for person re-
identification. In The IEEE Conference on Computer
Vision and Pattern Recognition (CVPR).
Mitzel, D. and Leibe, B. (2012). Close-range human detec-
tion and tracking for head-mounted cameras. In Pro-
ceedings of the British Machine Vision Conference,
pages 8.1–8.11. BMVA Press.
Munaro, M., Basso, A., Fossati, A., Gool, L. V., and
Menegatti, E. (2014a). 3d reconstruction of freely
moving persons for re-identification with a depth
sensor. In 2014 IEEE International Conference on
Robotics and Automation (ICRA), pages 4512–4519.
Munaro, M., Fossati, A., Basso, A., Menegatti, E.,
and Van Gool, L. (2014b). One-shot person re-
identification with a consumer depth camera. In Gong,
S., Cristani, M., Yan, S., and Loy, C. C., editors, Per-
son Re-Identification, pages 161–181. Springer Lon-
don, London.
Munaro, M., Ghidoni, S., Dizmen, D. T., and Menegatti,
E. (2014c). A feature-based approach to people re-
identification using skeleton keypoints. In 2014 IEEE
International Conference on Robotics and Automation
(ICRA), pages 5644–5651.
Nanni, L., Munaro, M., Ghidoni, S., Menegatti, E., and
Brahnam, S. (2016). Ensemble of different ap-
proaches for a reliable person re-identification system.
Applied Computing and Informatics, 12(2):142 – 153.
Paisitkriangkrai, S., Shen, C., and van den Hengel, A.
(2015). Learning to rank in person re-identification
with metric ensembles. In The IEEE Conference on
Computer Vision and Pattern Recognition (CVPR).
Pala, F., Satta, R., Fumera, G., and Roli, F. (2016). Mul-
timodal person reidentification using rgb-d cameras.
IEEE Transactions on Circuits and Systems for Video
Technology, 26(4):788–799.
Shotton, J., Fitzgibbon, A., Cook, M., Sharp, T., Finocchio,
M., Moore, R., Kipman, A., and Blake, A. (2011).
Real-time human pose recognition in parts from sin-
gle depth images. In Proceedings of the 2011 IEEE
Conference on Computer Vision and Pattern Recogni-
tion, CVPR ’11, pages 1297–1304, Washington, DC,
USA. IEEE Computer Society.
Vezzani, R., Baltieri, D., and Cucchiara, R. (2013). People
reidentification in surveillance and forensics: A sur-
vey. ACM Comput. Surv., 46(2):29:1–29:37.
Wang, X., Doretto, G., Sebastian, T., Rittscher, J., and Tu,
P. (2007). Shape and appearance context modeling. In
IN: PROC. ICCV (2007.
Weinrich, C., Volkhardt, M., and Gross, H. M. (2013).
Appearance-based 3d upper-body pose estimation and
person re-identification on mobile robots. In 2013
IEEE International Conference on Systems, Man, and
Cybernetics, pages 4384–4390.
VISAPP 2017 - International Conference on Computer Vision Theory and Applications
396