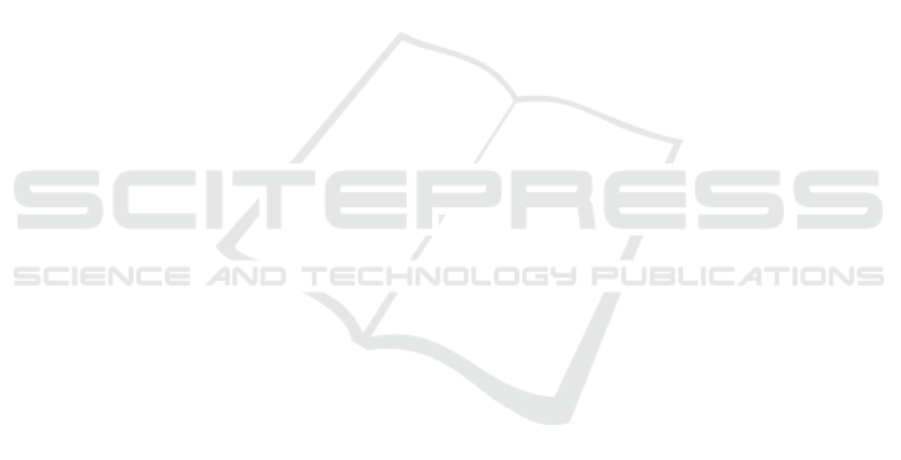
to evaluations in individual directions, we also con-
ducted view-point invariant Re-ID experiments in re-
alistic conditions where people walk in different di-
rections. Three cases studies were conducted in this
regard viz. pseudo, quasi and full view-point in-
variant. It is found that our system is quite robust
and promising with a Rank-1 Re-ID rate of ∼92%
in view-point dependent scenarios and ∼71%, ∼65%
and ∼48% in pseudo, quasi and full view-point in-
dependent scenarios, respectively. Since the direct
comparison with other works are not possible due to
the novelty of the approach, we carry out compara-
tive analysis against the most similar view-point de-
pendent approach (Gianaria et al., 2014) in the front
view, and very similar Re-ID results (19 times and 18
times the chance level, respectively) were reported.
In the future, we envisage to extrapolate this study
by collecting more data in more random directions of
walk. Also, in terms of the feature fusion, we would
like to employ context based fusion or feature selec-
tion strategies (eg: quasi-exhaustive learning strategy
(Barbosa et al., 2012), correlation-based feature sub-
set selection (Andersson and Araujo, 2015)), in order
to fine tune the selection of most informative features
and thus improve the Re-ID accuracy.
ACKNOWLEDGEMENTS
This work was supported by the FCT projects
[UID/EEA/50009/2013], AHA CMUP-
ERI/HCI/0046/2013 and FCT doctoral grant
[SFRH/BD/97258/2013].
REFERENCES
Aarai, K. and Andrie, R. (2013). 3d skeleton model de-
rived from kinect depth sensor camera and its appli-
cation to walking style quality evaluations. In Inter-
national Journal of Advanced Research in Artificial
Intelligence 2.
Andersson, V. O. and Araujo, R. M. (2015). Person identifi-
cation using anthropometric and gait data from kinect
sensor. In Proceedings of the Twenty-Ninth AAAI Con-
ference on Artificial Intelligence.
Ariyanto, G. and Nixon, M. S. (2011). Model-based 3d gait
biometrics. In In International Joint Conference on
Biometrics (IJCB).
Barbosa, I. B., Cristani, M., Alessio, D. B., Bazzani, L.,
and Murino, V. (2012). Re-identification with rgb-d
sensors. In Computer VisionECCV 2012. Workshops
and Demonstrations.
Dantcheva, A., Velardo, C., D’angelo, A., and Dugelay, J.
(2010). Bag of soft biometrics for person identifica-
tion : New trends and challenges. In Mutimedia Tools
and Applications, Springer.
Doretto, G., Sebastian, T., Tu, P., and Rittscher, J. (2011).
Appearance-based person reidentification in camera
networks: Problem overview and current approaches.
In Journal of Ambient Intelligence and Humanized
Computing, 2.
Fernandez, D., Madrid-Cuevas, F., Carmona-Poyato, A.,
Muoz-Salinas, R., and Medina-Carnicer, R. (2016). A
new approach for multi-view gait recognition on un-
constrained paths. In Journal of Visual Communica-
tion and Image Representation 38.
Gabel, M., Gilad-Bachrach, R., Renshaw, E., and Schuste,
A. (2012). Full body gait analysis with kinect. In An-
nual International Conference of the IEEE Engineer-
ing in Medicine and Biology Society (EMBC).
Gianaria, E., Grangetto, M., Lucenteforte, M., and
Balossino, N. (2014). Human classification using gait
features. In Biometric Authentication 8897.
Grother, P. and Phillips, P. J. (2004). Models of large pop-
ulation recognition performance. In Proceedings of
the 2004 IEEE Computer Society Conference on Com-
puter Vision and Pattern Recognition.
Iwashita, Y., Baba, R., Ogawara, K., and Kurazume, R.
(2010). Person identification from spatio-temporal 3d
gait. In Proceedings of the International Conference
on Emerging Security Technologies.
Iwashita, Y., Ogawarab, K., and Kurazume, R. (2014). Iden-
tification of people walking along curved trajectories.
In Pattern Recognition Letters 48.
Lee, L. and Grimson, W. (2002). Gait analysis for recog-
nition and classification. In Proc. IEEE International
Conference on Automatic Face and Gesture Recogni-
tion.
Nixon, M. S., Correia, P. L., Nasrollahi, K., Moeslund,
T. B., Hadidd, A., and Tistarelli, M. (2015). On soft
biometrics. In Pattern Recognition Letters 68.
Riccio, D., Marsico, M., Distasi, R., and Ricciardi, S.
(2014). A comparison of approaches for person re-
identification. In International Conference on Pattern
Recognition Applications and Methods.
Seely, R. D., Samangooei, S., Middleton, L., Carter, J. N.,
and Nixon, M. S. (2008). The university of southamp-
ton multi-biometric tunnel and introducing a novel 3d
gait dataset. In 2nd IEEE International Conference on
Biometrics: Theory, Applications and Systems BTAS.
Shotton, J., Sharp, T., Kipman, A., Fitzgibbon, A., Finoc-
chio, M., Blake, A., Cook, M., and Moore, R. (2013).
Real-time human pose recognition in parts from sin-
gle depth images. In Communications of the ACM
(CACM), 56(1).
Sivapalan, S., Chen, D., Denman, S., Sridharan, S., and
Fookes, C. (2011). 3d ellipsoid fitting for multiview
gait recognition. In In Proceedings of 8th IEEE Inter-
national Conference on Advanced Video and Signal-
Based Surveillance (AVSS).
Zhao, G., Liu, G., Li, H., and Pietikinen, M. (2006). 3d
gait recognition using multiple cameras. In 7th Inter-
national Conference on Automatic Face and Gesture
Recognition.
Towards View-point Invariant Person Re-identification via Fusion of Anthropometric and Gait Features from Kinect Measurements
119