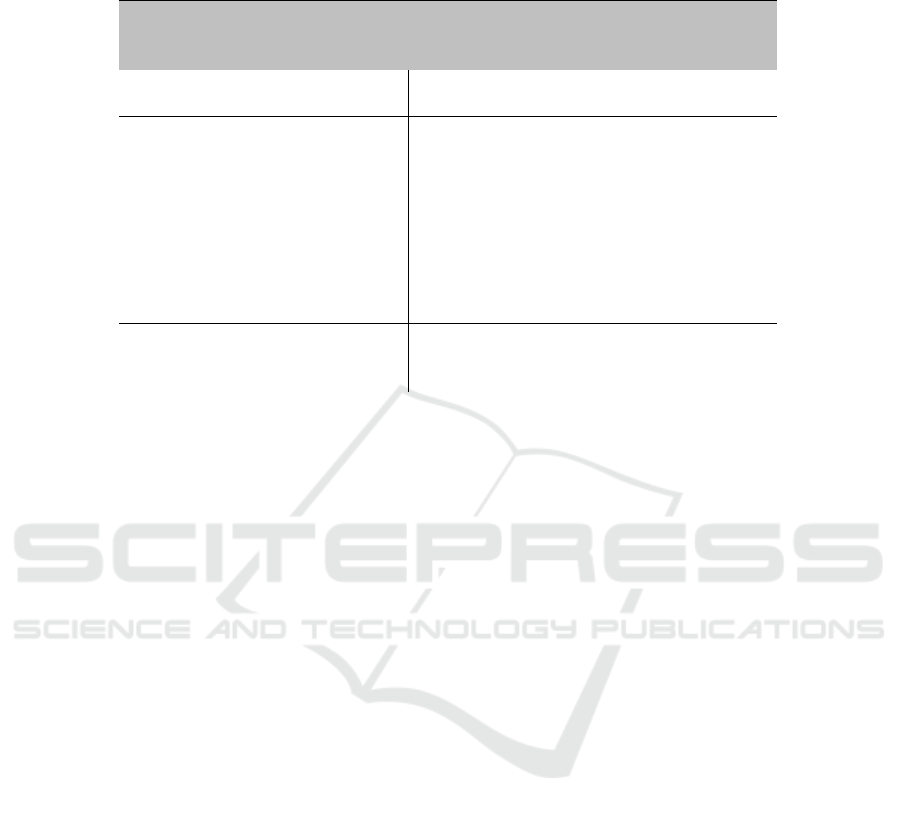
Table 3: Cross-dataset material classification results. Training and testing are performed using 3 different databases of real-
world images. The name on the top denotes the training database, while the name on the bottom implies the testing database.
Bold font highlights the leading mean result for every experiment.
FMD
ImageNet7
FMD
MINC-2500
MINC-2500
ImageNet7
Method Image Aug.
mAP mAP mAP
(a) CNN F (C) f s 78.23 71.87 85.11
(b) CNN S (C) f s 83.50 72.95 86.18
(c) CNN M - 82.40 73.06 87.64
(d) CNN M (C) f s 81.68 74.82 85.79
(e) CNN M (C) f m 81.69 75.46 86.55
(f) CNN M (C) s s 79.52 73.56 89.88
(g) CNN M (C) t t 80.22 74.19 89.53
(h) CNN M (C) f - 80.31 73.83 82.71
(i) CNN M (F) f - 81.91 73.01 91.03
(j) CNN M GS - 71.82 66.78 89.37
(k) CNN M GS (C) f s 75.95 69.05 87.87
(l) CNN M 2048 (C) f s 80.27 76.35 86.82
(m) CNN M 1024 (C) f s 82.55 74.85 87.89
(n) CNN M 128 (C) f s 82.90 73.99 88.13
REFERENCES
Bell, S., Upchurch, P., Snavely, N., and Bala, K. (2015).
Material recognition in the wild with the materials in
context database. In IEEE Conference on Computer
Vision and Pattern Recognition, CVPR, 2015, Boston,
MA, USA, June 7-12, 2015, pages 3479–3487.
Chatfield, K., Simonyan, K., Vedaldi, A., and Zisserman,
A. (2014). Return of the devil in the details: Delv-
ing deep into convolutional nets. In British Machine
Vision Conference, BMVC 2014, Nottingham, UK,
September 1-5, 2014.
Cimpoi, M., Maji, S., Kokkinos, I., Mohamed, S., and
Vedaldi, A. (2014). Describing textures in the wild. In
2014 IEEE Conference on Computer Vision and Pat-
tern Recognition, CVPR 2014, Columbus, OH, USA,
June 23-28, 2014, pages 3606–3613.
Csurka, G., Bray, C., Dance, C., and Fan, L. (2004). Visual
categorization with bags of keypoints. Workshop on
Statistical Learning in Computer Vision, ECCV, pages
1–22.
Dana, K. J., van Ginneken, B., Nayar, S. K., and Koen-
derink, J. J. (1999). Reflectance and texture of real-
world surfaces. ACM Trans. Graph., 18(1):1–34.
Deng, J., Dong, W., Socher, R., Li, L., Li, K., and Li, F.
(2009). Imagenet: A large-scale hierarchical image
database. In 2009 IEEE Computer Society Conference
on Computer Vision and Pattern Recognition (CVPR
2009), 20-25 June 2009, Miami, Florida, USA, pages
248–255.
Donahue, J., Jia, Y., Vinyals, O., Hoffman, J., Zhang, N.,
Tzeng, E., and Darrell, T. (2014). Decaf: A deep con-
volutional activation feature for generic visual recog-
nition. In Proceedings of the 31th International Con-
ference on Machine Learning, ICML 2014, Beijing,
China, 21-26 June 2014, pages 647–655.
Fritz, M., Hayman, E., Caputo, B., and olof Eklundh, J.
(2004). THE KTH-TIPS database.
Girshick, R., Donahue, J., Darrell, T., and Malik, J. (2014).
Rich feature hierarchies for accurate object detection
and semantic segmentation. In The IEEE Conference
on Computer Vision and Pattern Recognition (CVPR).
Girshick, R. B., Donahue, J., Darrell, T., and Malik, J.
(2013). Rich feature hierarchies for accurate ob-
ject detection and semantic segmentation. CoRR,
abs/1311.2524.
Hu, D., Bo, L., and Ren, X. (2011). Toward robust material
recognition for everyday objects. In British Machine
Vision Conference, BMVC 2011, Dundee, UK, August
29 - September 2, 2011. Proceedings, pages 1–11.
Huang, Y., Huang, K., Yu, Y., and Tan, T. (2011). Salient
coding for image classification. In Computer Vision
and Pattern Recognition (CVPR), 2011 IEEE Confer-
ence on, pages 1753–1760. IEEE.
Jia, Y., Shelhamer, E., Donahue, J., Karayev, S., Long, J.,
Girshick, R., Guadarrama, S., and Darrell, T. (2014).
Caffe: Convolutional architecture for fast feature em-
bedding. In Proceedings of the 22Nd ACM Inter-
national Conference on Multimedia, MM ’14, pages
675–678, New York, NY, USA. ACM.
Krizhevsky, A., Sutskever, I., and Hinton, G. E. (2012). Im-
agenet classification with deep convolutional neural
networks. In Advances in Neural Information Pro-
cessing Systems 25: 26th Annual Conference on Neu-
ral Information Processing Systems 2012. Proceed-
ings of a meeting held December 3-6, 2012, Lake
Tahoe, Nevada, United States., pages 1106–1114.
LeCun, Y., Boser, B., Denker, J. S., Henderson, D., Howard,
R. E., Hubbard, W., and Jackel, L. D. (1989). Back-
propagation applied to handwritten zip code recogni-
tion. Neural Computation, 1(4):541–551.
Evaluating Deep Convolutional Neural Networks for Material Classification
351