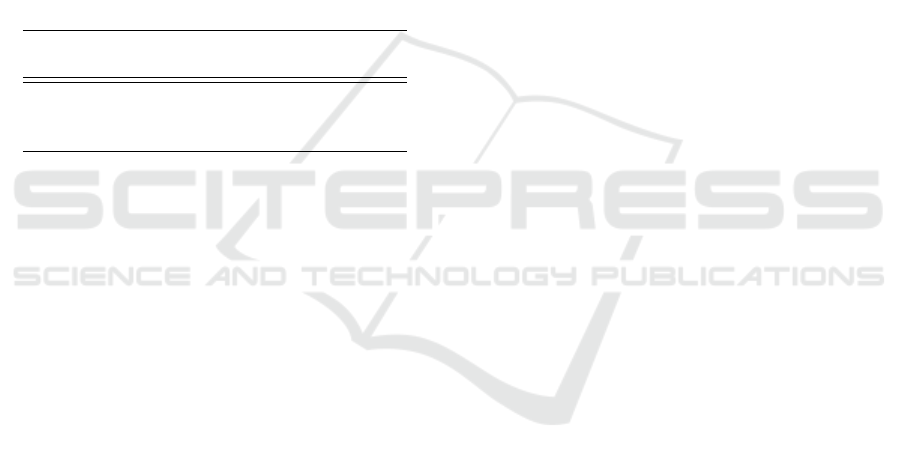
the alarm-specific bins at the input, TW layer
over 5 concatenated MSMP input frames and a
second fully connected hidden layer of 8 units
(SF(±2)+TW+FC, see Table 6)
The results shown in Table 7 are the metric scores
averaged over the 7 considered alarm classes. The
baseline model and GIM have the same number of
training weights for all the classes as they have the
same input. But the CIM structure has a different
number of weights depending on the number of class-
specific frequency components. Namely, for each
alarm class, all its frequency components (shown in
Table 1) were included at the input; thus, each alarm-
class has a different number of network parameters.
Note that the number of network weights provided in
Table 7 for CIM is an average value over all alarm-
specific structures.
Table 7: Best model structure results over all alarm classes.
System
Evaluation metrics (%) Network
weightsMR=FAR PB-ERR
Baseline 23.42 68.68 8218
GIM 17.76 54.80 482
CIM 11.13 53.75 330
Both kinds of input models improve significantly
the baseline results, and the proposed CIM struc-
ture clearly outperforms GIM. It can be seen that the
event-based evaluation was considerably improved by
both input model structures with respect to the base-
line, but it still has much room for improvement.
5 CONCLUSIONS
In this work several neural network based structures
were presented to detect alarm sounds in a NICU en-
vironment. Two kind of models based on different in-
put formats, that either make use or not of the knowl-
edge about the alarm class properties, were proposed
and tested: generic and class-specific. Due to the
scarcity of available annotated data, the number of
layers and nodes was kept small. Both linear and non-
linear pooling techniques were considered in order to
reduce the size of the input. Also, in order to exploit
frequency and temporal information while reducing
that network complexity, two types of partially con-
nected hidden layers were implemented.
As expected, it was observed that the class-
specific input model, which takes advantage of the
knowledge about the alarm frequency components,
yielded better results than the generic input model;
however, the structure of the latter model has does
not need to be adapted to each new alarm class. Also,
both types of partial connections, which make a sig-
nificant reduction of training weights, improved the
error rate, especially the time-based one.
The detection rate is still high, but we believe
that, when a much bigger dataset will be available
and used, the differences in performance among the
various tested neural net structures that have been ob-
served in this work will be substantially kept.
ACKNOWLEDGEMENTS
This work has been supported by the Spanish
government (contracts TEC2012-38939-C03-02 and
TEC2015-69266-P) as well as by the European Re-
gional Development Fund (ERDF/ FEDER). The
authors are grateful to Ana Riverola de Veciana
and Blanca Muoz Mahamud for their work on the
database collection and on the medical aspects of this
study, and to Vanessa Sancho Torrents and Francisco
Alarc
´
on Sanz for their work on the database annota-
tion.
REFERENCES
Abdel-Hamid, O., Deng, L., and Yu, D. (2013). Explor-
ing convolutional neural network structures and op-
timization techniques for speech recognition. In IN-
TERSPEECH, pages 3366–3370.
Beritelli, F., Casale, S., Russo, A., and Serrano, S. (2006).
An Automatic Emergency Signal Recognition System
for the Hearing Impaired. In Proceedings of the IEEE
Digital Signal Processing Workshop, pages 179–182.
Carbonneau, M.-A., Lezzoum, N., Voix, J., and Gagnon,
G. (2013). Detection of alarms and warning signals
on an digital in-ear device. International Journal of
Industrial Ergonomics, 43(6):503–511.
Ellis, D. P. (2001). Detecting alarm sounds. In Consistent
& Reliable Acoustic Cues for Sound Analysis: One-
day Workshop: Aalborg, Denmark, Sunday, Septem-
ber 2nd, 2001, pages 59–62. Department of Electrical
Engineering, Columbia University.
Freudenthal, A., Stuijvenberg, M. v., and Goudoever, J.
B. v. (2013). A quiet NICU for improved infants
health, development and well-being: a systems ap-
proach to reducing noise and auditory alarms. Cog-
nition, Technology & Work, 15(3):329–345.
Golik, P., Doetsch, P., and Ney, H. (2013). Cross-entropy
vs. squared error training: a theoretical and experi-
mental comparison. In INTERSPEECH, pages 1756–
1760.
Lecun, Y., Bottou, L., Orr, G., and M
¨
uller, K. (1998). Effi-
cient backprop.
BIOSIGNALS 2017 - 10th International Conference on Bio-inspired Systems and Signal Processing
90