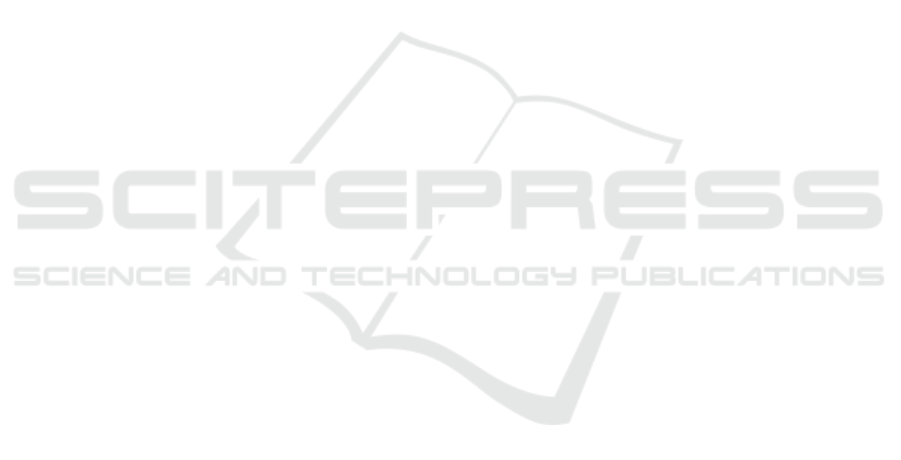
a previous study. Due to the lack of data correlat-
ing all the necessary variables It was modeled using
a manual construction methodology, certainty expres-
sions from the scientific literature were converted into
probabilities.
The analysis was divided into three parts: Caries
Risk, Treatment, and Return. The sampled data were
presented in contingency tables and in radar charts,
allowing a visual analysis of the dependence of the
CDSS suggestions and input variables states.
Results showed the behavior of CDDS model in
different scenarios. The certainty about suggestions
is present in some scenarios, e.g., the patient return
is equal to 1 year if Caries Risk is low, and Lesion
Activity is no. For return before 6 moths, Lesion Ac-
tivity variable needs to be true, and Caries Risk needs
to be high. In contrast, uncertainty is present in oth-
ers suggestions states, e.g., in scenarios with a Low
Caries Risk.
The analysis shows the system deals with the un-
certainty inherent in the clinical processes. Further-
more, its infers according to the clinical standards pre-
sented in the literature of cariology. It can be helpful
as a second opinion during dental caries clinical man-
agement. The next steps are to quantify the different
levels of uncertainty present in the model and evalu-
ate the performance of the system by comparison with
experts decisions.
ACKNOWLEDGMENTS
The authors would like to thank CAPES - Brazil-
ian Federal Agency for Support and Evaluation of
Graduate Education within the Ministry of Educa-
tion of Brazil; and to CNPq - National Counsel of
Technological and Scientific Development - Project
475064/2013-5.
REFERENCES
Baelum, V. (2008). Caries management: technical solutions
to biological problems or evidence-based care? Jour-
nal of oral rehabilitation, 35(2):135–51.
Berner, E. S. (2007). Clinical decision support systems:
theory and practice. Springer Science & Business
Media.
Bessani, M., Lins, E. C. C. C., Delbem, A. C. B., and Ma-
ciel, C. D. (2014). Construction of a Clinical Decision
Support System for Dental Caries Management using
Bayesian Networks. In XXIV Brazilian Congress on
Biomedical Engineering - CBEB 2014, pages 517–
520.
Castaneda, C., Nalley, K., Mannion, C., Bhattacharyya, P.,
Blake, P., Pecora, A., Goy, A., and Suh, K. S. (2015).
Clinical decision support systems for improving di-
agnostic accuracy and achieving precision medicine.
Journal of clinical bioinformatics, 5(1):1.
Deshpande, R. R., DeMarco, J., Sayre, J. W., and Liu, B. J.
(2016). Knowledge-driven decision support for as-
sessing dose distributions in radiation therapy of head
and neck cancer. International journal of computer
assisted radiology and surgery, pages 1–13.
Ferreira Zandon
´
a, A., Santiago, E., Eckert, G. J., Katz, B. P.,
Pereira de Oliveira, S., Capin, O. R., Mau, M., and
Zero, D. T. (2012). The natural history of dental caries
lesions: a 4-year observational study. Journal of den-
tal research, 91(9):841–6.
Genes, N., Kim, M. S., Thum, F. L., Rivera, L., Beato, R.,
Song, C., Soriano, J., Kannry, J., Baumlin, K., Hwang,
U., et al. (2016). Usability evaluation of a clinical de-
cision support system for geriatric ed pain treatment.
Applied clinical informatics, 7(1):128–142.
Goh, W. P., Tao, X., Zhang, J., and Yong, J. (2016). Deci-
sion support systems for adoption in dental clinics: a
survey. Knowledge-Based Systems, 104:195–206.
Guo, H. and Hsu, W. (2002). A survey of algo-
rithms for real-time bayesian network inference. In
AAAI/KDD/UAI02 Joint Workshop on Real-Time De-
cision Support and Diagnosis Systems. Edmonton,
Canada.
Hayduk, L., Cummings, G., Stratkotter, R., Nimmo, M.,
Grygoryev, K., Dosman, D., Gillespie, M., Pazderka-
Robinson, H., and Boadu, K. (2003). Pearl’s d-
separation: One more step into causal thinking. Struc-
tural Equation Modeling: A Multidisciplinary Jour-
nal, 10(2):289–311.
Hogenboom, F., Frasincar, F., Kaymak, U., de Jong, F., and
Caron, E. (2016). A survey of event extraction meth-
ods from text for decision support systems. Decision
Support Systems, 85:12–22.
Holmes, D. and Jain, L. (2008). Introduction to bayesian
networks. In Holmes, D. and Jain, L., editors, Inno-
vations in Bayesian Networks, volume 156 of Stud-
ies in Computational Intelligence, pages 1–5. Springer
Berlin Heidelberg.
Jensen, F. and Nielsen, T. (2007). Bayesian networks and
decision graphs. Springer Science+Business Media,
New York, 2nd ed edition.
Kjaerulff, U. B. and Madsen, A. L. (2010). Bayesian Net-
works and Influence Diagrams: A Guide to Construc-
tion and Analysis. Springer Publishing Company, In-
corporated, 1st edition.
Koller, D. and Friedman, N. (2009). Probabilistic graphical
models: principles and techniques. MIT press.
Lambin, P., van Stiphout, R. G., Starmans, M. H., Rios-
Velazquez, E., Nalbantov, G., Aerts, H. J., Roelofs,
E., van Elmpt, W., Boutros, P. C., Granone, P., et al.
(2013). Predicting outcomes in radiation oncology-
multifactorial decision support systems. Nature Re-
views Clinical Oncology, 10(1):27–40.
Lepar, V. and Shenoy, P. P. (1998). A comparison of
lauritzen-spiegelhalter, hugin, and shenoy-shafer ar-
chitectures for computing marginals of probability
Evaluation of a Dental Caries Clinical Decision Support System
203