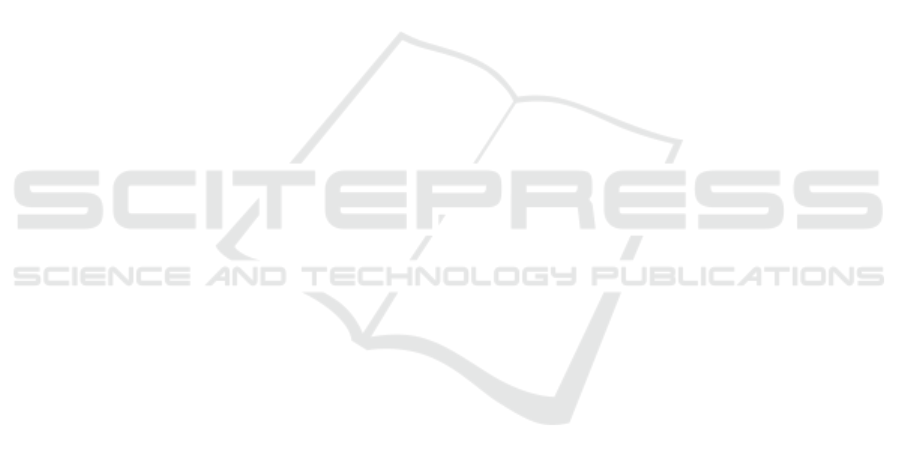
de Oliveira, M. F. and Levkowitz, H. (2003). From visual
data exploration to visual data mining: a survey. IEEE
Transactions on Visualization and Computer Graph-
ics, 9(3):378–394.
Fayyad, U. M., Wierse, A., and Grinstein, G. G. (2002). In-
formation visualization in data mining and knowledge
discovery. Morgan Kaufmann.
Ferdosi, B. J., Buddelmeijer, H., Trager, S., Wilkinson,
M. H., and Roerdink, J. B. (2010). Finding and
visualizing relevant subspaces for clustering high-
dimensional astronomical data using connected mor-
phological operators. In Visual Analytics Science and
Technology (VAST), 2010 IEEE Symposium on, pages
35–42. IEEE.
Gao, J., Li, J., Zhang, Z., and Tan, P.-N. (2005). An in-
cremental data stream clustering algorithm based on
dense units detection. In Pacific-Asia Conference on
Knowledge Discovery and Data Mining, pages 420–
425. Springer.
Goil, S., Nagesh, H., and Choudhary, A. (1999). Mafia: Ef-
ficient and scalable subspace clustering for very large
data sets. In Proceedings of the 5th ACM SIGKDD In-
ternational Conference on Knowledge Discovery and
Data Mining, pages 443–452. ACM.
Havre, S., Hetzler, E., Whitney, P., and Nowell, L. (2002).
Themeriver: Visualizing thematic changes in large
document collections. IEEE transactions on visual-
ization and computer graphics, 8(1):9–20.
Hawkins, D. M. (1980). Identification of outliers, vol-
ume 11. Springer.
Hund, M., B
¨
ohm, D., Sturm, W., Sedlmair, M., Schreck, T.,
Ullrich, T., Keim, D. A., Majnaric, L., and Holzinger,
A. (2016). Visual analytics for concept exploration in
subspaces of patient groups. Brain Informatics, pages
1–15.
Keim, D. A. (2002). Information visualization and visual
data mining. IEEE transactions on Visualization and
Computer Graphics, 8(1):1–8.
Keim, D. A., Mansmann, F., Schneidewind, J., and Ziegler,
H. (2006). Challenges in visual data analysis. In
Tenth International Conference on Information Visu-
alisation (IV’06), pages 9–16. IEEE.
Kovalerchuk, B. and Schwing, J. (2005). Visual and spa-
tial analysis: advances in data mining, reasoning, and
problem solving. Springer Science & Business Media.
Kriegel, H.-P., Kr
¨
oger, P., Ntoutsi, I., and Zimek, A. (2011).
Density based subspace clustering over dynamic data.
In International Conference on Scientific and Statisti-
cal Database Management, pages 387–404. Springer.
Lichman, M. (2013). Uci machine learning repository.
https://archive.ics.uci.edu/ml/datasets/KDD+Cup+19
99+Data. (consulted on: 11.12.2015).
Louhi, I., Boudjeloud-Assala, L., and Tamisier, T. (2016).
Incremental nearest neighborhood graph for data
stream clustering. In 2016 International Joint Con-
ference on Neural Networks, IJCNN 2016, Vancouver,
BC, Canada, July 24-29, 2016, pages 2468–2475.
Muller, E., Assent, I., Krieger, R., Jansen, T., and Seidl,
T. (2008). Morpheus: interactive exploration of sub-
space clustering. In Proceedings of the 14th ACM
SIGKDD international conference on Knowledge dis-
covery and data mining, pages 1089–1092. ACM.
Pearson, K. (1901). Liii. on lines and planes of closest fit to
systems of points in space. The London, Edinburgh,
and Dublin Philosophical Magazine and Journal of
Science, 2(11):559–572.
Soukup, T. and Davidson, I. (2002). Visual data mining:
Techniques and tools for data visualization and min-
ing. John Wiley & Sons.
Tatu, A., Zhang, L., Bertini, E., Schreck, T., Keim, D.,
Bremm, S., and Von Landesberger, T. (2012). Clust-
nails: Visual analysis of subspace clusters. Tsinghua
Science and Technology, 17(4):419–428.
Torgerson, W. S. (1958). Theory and methods of scaling.
Vadapalli, S. and Karlapalem, K. (2009). Heidi matrix:
nearest neighbor driven high dimensional data visu-
alization. In Proceedings of the ACM SIGKDD Work-
shop on Visual Analytics and Knowledge Discovery:
Integrating Automated Analysis with Interactive Ex-
ploration, pages 83–92. ACM.
Subspace Clustering and Visualization of Data Streams
265