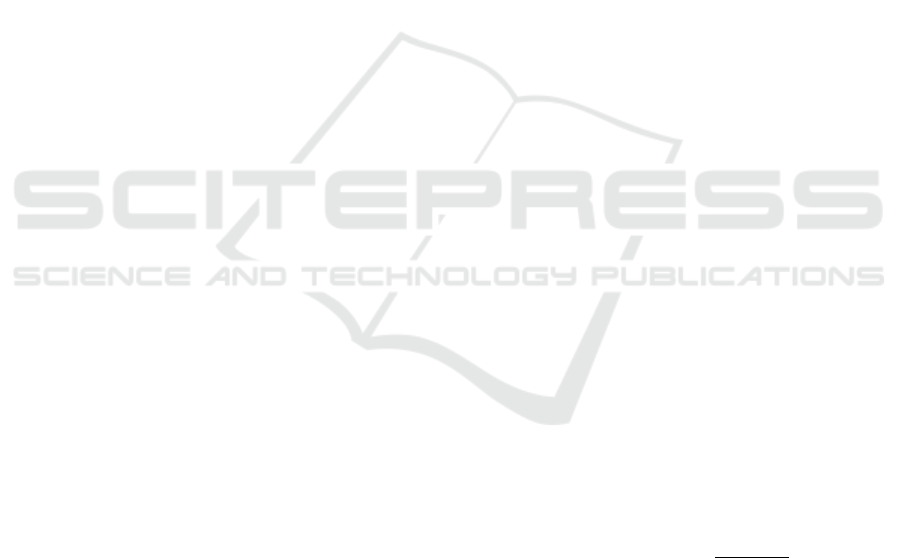
the head. However, light sources are usually located
above the head. Due to that, there is some reflection
and regions with different luminance.
The studies (M.Z.Poh and Picard, 2011), (Y. Sun
and Greenwald, 2013) evaluate the HRV parameters
in comparison with the contact photoplethysmogra-
phy (PPG). However, the most examined and reliable
way to determine the changes of the autonomic ner-
vous system by means of the HRV evaluation is based
on electrocardiography (ECG).
Earlier, we have presented the possibilities of as-
sessing the VLF and LF parameters assessment by
using the PCA-based rPPG (Kublanov and Purtov,
2015). However, it was shown that this method does
not give a correct estimation of the HF band.
The main idea of this work is to evaluate the pos-
sibilities of rPPG for detecting changes of HRV in the
HF band. To do this, we evaluate the accuracy of the
rPPG methods compared with the parameters of HRV
obtained by ECG.
Besides, we measure the respiratory rate (RR) by
using the motion tracking techniques with preprocess-
ing by Eulerian Video Magnification method. Such
techniques now are very popular, but they work only
if the person has relatively stable position in time.
2 MATERIALS AND METHODS
2.1 Methods
In recent years researchers have presented a number
of new methods for recovering rPPG signals. Their
full comparison with all the components and condi-
tions is not possible in a single article. Therefore, in
this paper we consider the methods, which:
1. Allow to process a signals in real-time;
2. Work with only one digital RGB camera;
3. Have varying physiological models of signals.
Thus, we choose the following methods: RoverG,
XoverY, CHROM, ICA, PCA, 2SR, POS. These
methods were proposed in the works (M.Z.Poh
and Picard, 2011),(D. McDuff and Picard, 2014),
(M. Lewandowska and Kocejko, 2011), (de Haan
and Jeanne, 2013), (W. Wang and de Haan, 2015),
(W. Wang and de Haan, 2016).
The settings for each of the rPPG method were
used in accordance with recommendations of the au-
thors. For example, the ICA method was imple-
mented in accordance with the specifications: we split
the signals to the 30 seconds overlap-add windows,
and use the JADE ICA algorithm. The pulse compo-
nent selection was based on the FFT analysis After
the selection, the signals were inverted according to
the rule: µ
peakamp
< µ
troughamp
,
where µ
peakamp
is the mean absolute peak value,
µ
troughamp
is the mean absolute trough value
Each rPPG method was used on the same skin
areas (pixels) to ensure the correctness of compari-
son. For each subject, the choice of skin boundaries
was made in HSV and YC
b
C
r
color formats. Before
the processing, the color boundaries were determined
manually to get the maximum skin area on the face
without areas with glare, because reflectance has great
influence on the parameters of rPPG signal.
In the first image of the video, the face area was
detected by using the Viola-Jones method (Viola and
Jones, 2001). In other images, it was tracked by us-
ing implementation of KCF method (F. Henriques and
Batista, 2015) which allows to work in real-time.
The method presented in a master thesis (Balakr-
ishnan, 2014) was used to RR detection. It allows to
estimate the RR signals by tracking the chest move-
ments with motion preprocessing by Eulerian video
magnification.
2.1.1 Evaluation Metrics
For comparison of different rPPG methods, we evalu-
ate their performance by the following metrics.
• The Pearson correlation coefficient was used to
evaluate the accuracy of different rPPG methods.
It allow to compare HR and HRV signals mea-
sured by rPPG with reference to PPG and ECG
signals.
• The signal to noise ratio (SNR) was used to as-
sess the quality of measured rPPG signals. It is
derived by the ratio between the energy around
the first HR harmonic and the remaining parts in
the 4 - 0.5 Hz frequency band. The location of the
first harmonic is determined by the contact PPG-
signal. It is was measured as follows:
SNR = 10 ·lg
(U
signal
)
2
(U
noise
)
2
, (1)
where U
signal
is the intensity of the first heart rate
harmonic, U
noise
is the intensity of the remaining
parts in the (4 - 0.5 Hz) frequency band.
2.2 Experiment
This work is performed at the Research and Devel-
opment Medical and Biological Engineering Center
of High Technologies, Ural Federal University (Rus-
sian Federation) with partisipation of employees of