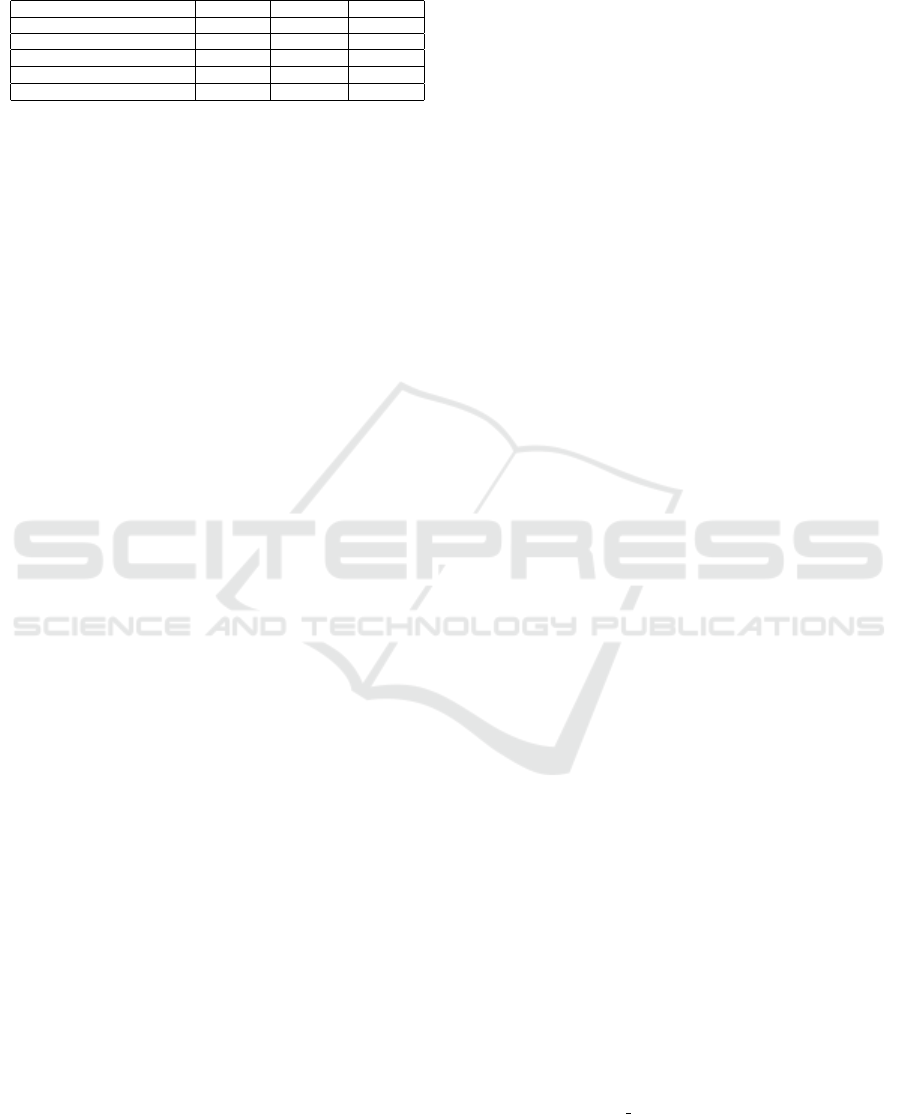
Table 2: Comparative performance of ILAM modeling
combined with an EM classifier to different features used
as inputs.
Method Face Det. False Det. Det. rates
HOG features 293 8 97.6%
LBP/LTP representation 294 6 98.1%
Patch-based SIFT-LBP 296 6 98.6%
LBP
4,1
+ LBP
8,2
+ LBP
u2
8,1
trait 294 9 98.0%
f
i
= { f
p
i
, f
g
i
, f
a
i
} trait 298 5 99.3%
• did not need to impose anyone constraint like his-
togram equalization; and
• principally needs a simple EM classifier to esti-
mates the latent data, than using a series of SVM
classifiers (Hadid et al., 2004; Vapnik, 1998).
7 CONCLUSION
The appearance representation of face class presented
in this paper offers robust properties such as tolerance
to geometric transforms and illumination changes. It
captures well the viewpoints variations and especially
intra-class variability. It has a geometric localiza-
tion sufficiently accurate and its magnitude remains
roughly constant with respect to size of object in im-
age. The ILAM model based on combination of lo-
cal appearance of Extended Harris-Laplace descriptor
and texture of LBP feature provides a low degree of
supervision. The experimentation reveals that the fa-
cial formulation is useful and has high capability to
classify new face instances, of course this representa-
tion can be applied to another object class.
REFERENCES
Agarwal, S., Awan, A., and Roth, D. (2004). Learning to
detect objects in images via a sparse, part-based rep-
resentation. In PAMI, 26(11), pp. 1475–1490.
Ahonen, T., Hadid, A., and Pietikinen, M. (2004). Face
recognition with local binary patterns. In Proc. of the
8th ECCV Conference.
AT&T-Database (1994). At&t: The database of faces. In
Cambridge University, http://www.cl.cam.ac.uk/.
Bart, E., Byvatov, E., and Ullman, S. (2004). View-invariant
recognition using corresponding object fragments. In
ECCV, pp 152-165.
CMU-Database (2009). Cmu face group and face detection
project, frontal and profile face images databases. In
http://vasc.ri.cmu.edu/idb/html/face/.
D
´
eniz, O., Bueno, G., Salido, J., and la Torre, F. D. (2011).
Face recognition using histograms of oriented gradi-
ents. In Pattern Recognition Letters, vol.32, pp:1598-
1603.
Etemad, K. and Chellappa, R. (1997). Discriminant analysis
for recognition of human face images. In Journal of
the Optical Society of America, vol.14, pp:1724-1733.
Fei-Fe, L., Fergus, R., and Perona, P. (2003). A bayesian
approach to unsupervised one-shot learning of object
categories. In ICCV, Nice, France, pp. 1134–1141.
FERET-Database (2009). Color feret face database. In
www.itl.nist.gov/iad/humanid/colorferet.
Fergus, R., Perona, P., and Zisserman, A. (2003). Ob-
ject class recognition by unsupervised scale-invariant
learning. In CVPR, Madison, Wisconsin, pp. 264–271.
Hadid, A. and Pietikinen, M. (2004). Selecting models
from videos for appearance-based face recognition. In
Proc. of the 17th International Conference on Pattern
Recognition (ICPR).
Hadid, A., Pietikinen, M., and Ahonen, T. (2004). A dis-
criminative feature space for detecting and recogniz-
ing faces. In CVPR Proceedings, Vol. 2, pp. 797–804.
Heisele, B., Poggio, T., and Pontil, M. (2000). Face detec-
tion in still gray images. In Technical Report 1687,
Center for Biological and Computational Learning,
MIT.
Kadir, T. and Brady, M. (2001). Saliency, scale and image
description. In IJCV, 45(2), pp. 83–105.
Lindeberg, T. (1998). Feature detection with automatic
scale selection. In International Journal of Computer
Vision, vol. 30(2), pp. 79-116.
Liu, C. (2003). A bayesian discriminating features method
for face detection. In IEEE Trans. on PAMI, vol. 25,
pp:725-740.
Lowe, D. (2004). Distinctive image features from scale-
invariant keypoints. In IJCV, 60(2), pp. 91–110.
Mikolajczyk, K. and Schmid, C. (2004). Scale and affine in-
variant interest point detectors. In IJCV, 60(1), pp. 63-
86.
Nanni, L., Brahnam, S., and Lumini, A. (2012). Random
interest regions for object recognition based on texture
descriptors and bag of features. In Expert Systems with
Applications, Elsevier Journal, vol.39, pp:973-977.
Ojala, T., Pietikinen, M., and Menp, T. (2002). Multires-
olution gray-scale and rotation invariant texture clas-
sification with local binary patterns. In IEEE Trans-
actions on Pattern Analysis and Machine Intelligence
(PAMI), vol.24, pp:971-987.
Penev, P. and Atick, J. (1996). Local feature analysis: a gen-
eral statistical theory for object representation. In Net-
work: Computation in Neural Systems, vol.7, pp:477-
500.
Phillips, P., Moon, H., Rizvi, S. A., and Rauss, P. J. (2000).
The feret evaluation methodology for face recognition
algorithms. In IEEE Trans. on PAMI, vol.22, pp:1090-
1104.
PIE-Database (2009). Cmu pose, illumination, and ex-
pression (pie) database. In http:/www.ri.cmu.edu/
projects/project 418.html.
Pope, A. and Lowe, D. (2000). Probabilistic models of ap-
pearance for 3-d object recognition. In IJCV, 40(2),
pp. 149–167.
ICPRAM 2017 - 6th International Conference on Pattern Recognition Applications and Methods
136