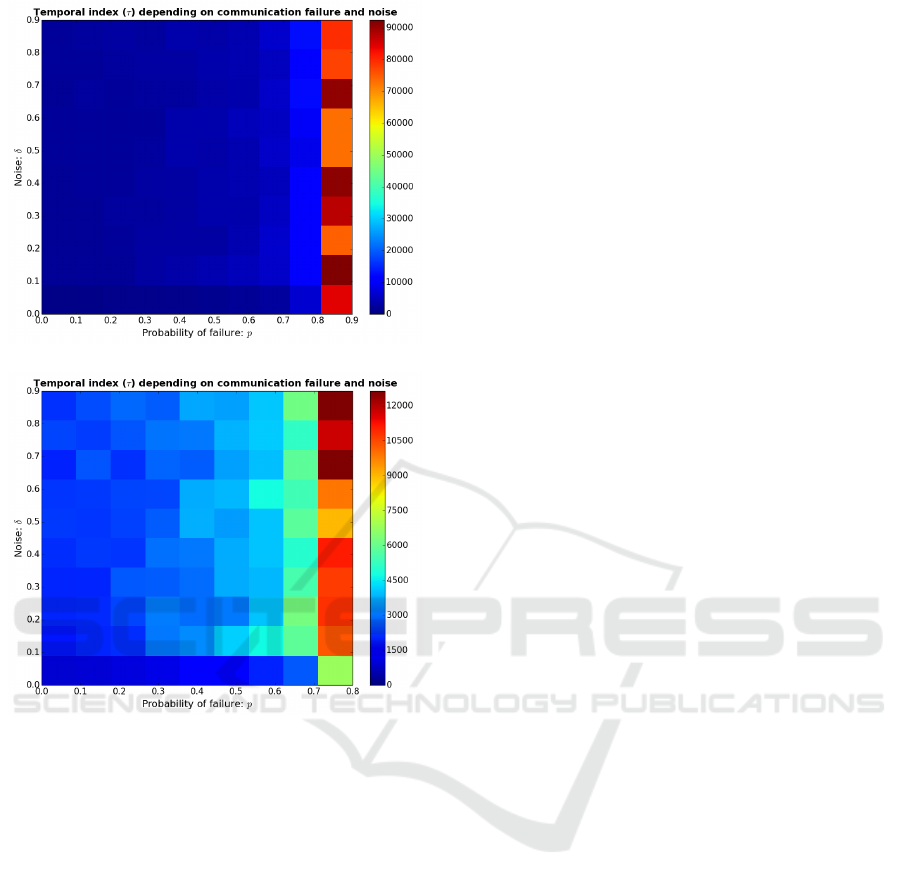
(a)
(b)
Figure 7: Temporal index (τ) depending on the probability
of communication failure p and a random noise ε ∈ [−δ, δ],
for S-2.2, with α
a
= 8, α
av
= 9 and α
cv
= 4. (a) communi-
cation failure with p ∈ [0, 0.9], (b) Zooming to p ∈ [0, 0.8].
Color represents τ.
tal issues as the presence of wind or terrain irregu-
larities. Results have shown that our model is quite
resilient to communication failures, and the presence
of noise, since their impact was small, specially for
noise.
Considering that agents have sensory limitations,
one can use this model as a pre-step before switching
to another model with more controls, since its princi-
ple is to group agents into a bounded region.
ACKNOWLEDGEMENTS
The authors would like to thank the Conselho Na-
cional de Desenvolvimento Cientifico e Tecnologico
- CNPq, and the Coordenacao de Aperfeicoamento
de Pessoal de Nivel Superior - CAPES, for the fi-
nancial support. EENM thanks FAPESP, processes
2011/50151-0 and 2015/50122-0, and CNPq, process
458070/2014-9, for their support.
REFERENCES
Chapman, A. D., Study., A. B. R., and Australia. (2009).
Numbers of living species in Australia and the world /
Arthur D. Chapman. Department of the Environment,
Water, Heritage and the Arts [Canberra, ACT].
Couzin, I. D., Krause, J., James, R., Ruxton, G. D., and
Franks, N. R. (2002). Collective memory and spatial
sorting in animal groups. Journal of Theoretical Biol-
ogy, 218(1):1 – 11.
Cucker, F. and Smale, S. (2007). Emergent behavior in
flocks. IEEE Transactions on Automatic Control,
pages 852–862.
Czir
´
ok, A., Barab
´
asi, A.-L., and Vicsek, T. (1999). Collec-
tive motion of self-propelled particles: Kinetic phase
transition in one dimension. Phys. Rev. Lett., 82:209–
212.
Dafflon, B., Gechter, F., Gruer, P., and Koukam, A. (2013).
Vehicle platoon and obstacle avoidance: A reactive
agent approach. IET Intelligent Transport Systems,
7(3):257–264.
Duarte, M., Costa, V., Gomes, J., Rodrigues, T., Silva, F.,
Oliveira, S. M., and Christensen, A. L. (2016). Evolu-
tion of collective behaviors for a real swarm of aquatic
surface robots. PLOS ONE, 11(3):1–25.
Herbert-Read, J. E., Perna, A., Mann, R. P., Schaerf, T. M.,
Sumpter, D. J. T., and Ward, A. J. W. (2011). Inferring
the rules of interaction of shoaling fish. Proceedings
of the National Academy of Sciences, 108(46):18726–
18731.
Katz, Y., Tunstrom, K., Ioannou, C. C., Huepe, C., and
Couzin, I. D. (2011). Inferring the structure and dy-
namics of interactions in schooling fish. Proceedings
of the National Academy of Sciences, 108(46):18720–
18725.
Kerman, S., Brown, D., and Goodrich, M. A. (2012). Sup-
porting human interaction with robust robot swarms.
In Resilient Control Systems (ISRCS), 2012 5th Inter-
national Symposium on, pages 197–202.
Klein, J. K. (2005). Controlled Collective Motion for Mul-
tivehicle Trajectory Tracking. Master’s thesis, Univer-
sity of Washington, USA.
Leonard, N., Paley, D., Lekien, F., Sepulchre, R., Fratan-
toni, D., and Davis, R. (2007). Collective motion, sen-
sor networks, and ocean sampling. Proceedings of the
IEEE, 95(1):48–74.
Paley, D., Leonard, N. E., Sepulchre, R., Gr
¨
unbaum, D.,
and Parrish, J. K. (2007). Oscillator models and col-
lective motion: Spatial patterns in the dynamics of en-
gineered and biological networks. IEEE Control Sys-
tems Magazine, 27(4):89–105.
Paley, D. A. (2007). Cooperative control of collective mo-
tion for ocean sampling with autonomous vehicles.
PhD thesis, Princeton University.
ICAART 2017 - 9th International Conference on Agents and Artificial Intelligence
172