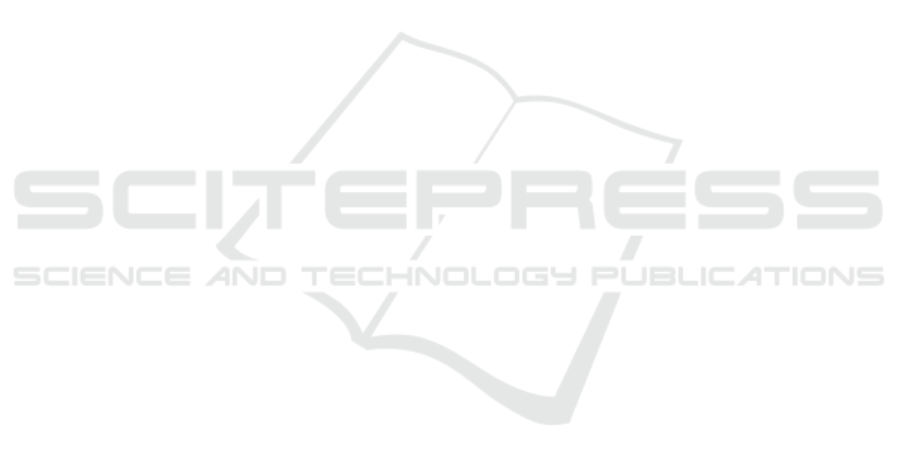
4 CONCLUSION AND FUTURE
WORK
The paper proposed a novel method for scene classi-
fication for endoscopic images into classes, based on
ink and blood vessel content using SVM with Cubic
kernel. The features were based on colour, edges in-
formation and texture. The blood vessel containing
non-dyed images were used for blood vessel extrac-
tion. The blood vessel extraction process is based on
the Frangi vesselness filter. The originality added by
the proposed method lies in its ability to differentiate
the edges extracted by Frangi filter into blood vessel
and non-blood vessel edges. The proposed algorithm
achieves this aim by doing background subtraction
and filtering using a custom intensity-based dissym-
metry detection filter. Blood vessel delineation for
dyed images is a topic of future work. Another work
is to apply this research to 3D recovery of polyp with
absolute size and shape for supporting medical image
diagnosis.
ACKNOWLEDGEMENT
This research was done while Mayank Golhar visited
Iwahori Lab. of Chubu University for his research
internship. Iwahori’s research is supported by JSPS
Grant-in-Aid for Scientific Research (C) (#26330210)
and (B) (#15H02768), and Chubu University Grant.
REFERENCES
Azzopardi, G., Strisciuglio, N., Vento, M., and Petkov, N.
(2015). Trainable cosfire filters for vessel delineation
with application to retinal images. Medical image
analysis, 19(1):46–57.
Frangi, A. F., Niessen, W. J., Vincken, K. L., and Viergever,
M. A. (1998). Multiscale vessel enhancement fil-
tering. In International Conference on Medical Im-
age Computing and Computer-Assisted Intervention,
pages 130–137. Springer.
Fraz, M. M., Remagnino, P., Hoppe, A., Uyyanonvara, B.,
Rudnicka, A. R., Owen, C. G., and Barman, S. A.
(2012). Blood vessel segmentation methodologies in
retinal images–a survey. In Computer methods and
programs in biomedicine, Vol. 108(1), pp. 407-433.
Elsevier.
Hafner, M., Gangl, A., Wrba, F., Thonhauser, K., Schmidt,
H.-P., Kastinger, C., Uhl, A., and Vecsei, A. (2007).
Comparison of k-nn, svm, and nn in pit pattern clas-
sification of zoom-endoscopic colon images using co-
occurrence histograms. In Image and Signal Process-
ing and Analysis, 2007. ISPA 2007. 5th International
Symposium on, pp. 516-521. IEEE.
Ikeda, N., Usami, H., Iwahori, Y., Bhuyan, M., and Ka-
sugai, K. (2016). Generating lambertian image by re-
moving specular reflection component and difference
of reflectance factor using hsv. In Proc. of ITC-CSCC
2016, T2-5, Computer Vision (2), pp.547-550.
Kumar, V., Abbas, A. K., Fausto, N., and Aster, J. C. (2014).
Robbins and Cotran pathologic basis of disease. El-
sevier Health Sciences, 9th edition.
Lin, B., Sun, Y., Sanchez, J. E., and Qian, X. (2015). Ef-
ficient vessel feature detection for endoscopic image
analysis. IEEE Transactions on Biomedical Engineer-
ing, 62(4):1141–1150.
Mohanty, A. A., Vaibhav, B., and Sethi, A. (2013). A frame-
based decision pooling method for video classifica-
tion. In 2013 Annual IEEE India Conference (INDI-
CON), pages 1–5. IEEE.
Zhang, D., Wong, A., Indrawan, M., and Lu, G. (2000).
Content-based image retrieval using gabor texture fea-
tures. In IEEE Pacific-Rim Conference on Multimedia,
University of Sydney, Australia, pages 392–395.
ICPRAM 2017 - 6th International Conference on Pattern Recognition Applications and Methods
156