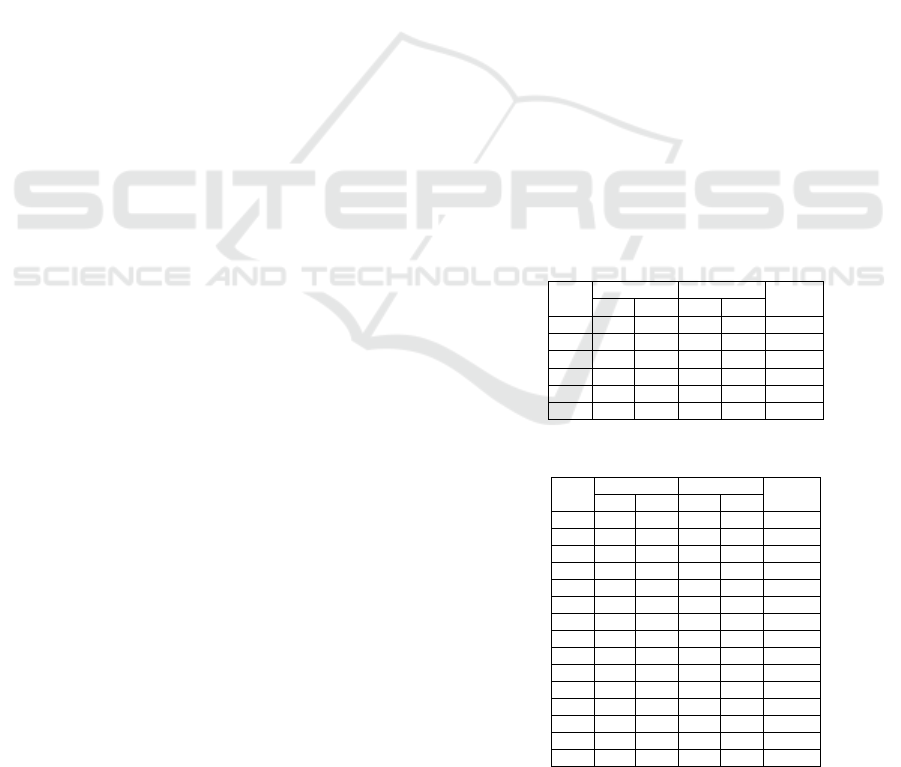
Cao, F., Ester, M., Qian, W. and Zhou, A., 2006. Density-
based clustering over an evolving data stream with
noise. Proceedings of the Sixth SIAM International
Conference on Data Mining, 2006, pp.328–339.
Chakraborty, S. and Nagwani, N.K., 2011. Analysis and
Study of Incremental K-Means. Springer-Verlag
Berlin Heidelberg, pp.338–341.
Dempster, A.P., Laird, N.M. and Rubin, D.B., 1977.
Maximum Likelihood from Incomplete Data via the
EM Algorithm. Journal of the Royal Statistical
Society. Series B (Methodological), 39(1), pp.1–38.
Ester, M., Kriegel, H.-P., Sander, J. and Xu, X., 1996. A
Density-Based Algorithm for Discovering Clusters in
Large Spatial Databases with Noise. the 2nd
International Conference on Know- ledge Discovery
and Data Mining, 2, pp.226–231.
Guha, S., Mishra, N., Motwani, R. and O’Callaghan, L.,
2000. Clustering Data Streams. IEEE FOCS
Conference, pp.359–366.
Islam, M.Z., 2013. A Cloud Based Platform for Big Data
Science. Department of Computer and Information
Science, Linköping University, pp.1–57.
Karypis, Y.Z. and George, 2001. Technical Report
Criterion Functions for Document Clustering:
Experiments and Analysis. , pp.1–30.
Kodinariya, T.M. and Makwana, P.R., 2013. Review on
determining number of Cluster in K-Means Clustering.
International Journal of Advance Research in
Computer Science and Management Studies, 1(6),
pp.2321–7782.
Kremer, H., Kranen, P., Jansen, T., Seidl, T., Bifet, A.,
Holmes, G. and Pfahringer, B., 2011. An effective
evaluation measure for clustering on evolving data
streams. Proceedings of the 17th ACM SIGKDD
International Conference on Knowledge Discovery
and Data Mining - KDD ’11, pp.868–876. Available
at:
http://eprints.pascal-network.org/archive/00008693/.
Liu, C., Ranjan, R., Zhang, X., Yang, C., Georgakopoulos,
D. and Chen, J., 2013. Public Auditing for Big Data
Storage in Cloud Computing - A Survey. 2013 IEEE
16th International Conference on Computational
Science and Engineering, pp.1128–1135. Available at:
http://ieeexplore.ieee.org/lpdocs/epic03/wrapper.htm?
arnumber=6755345.
MacQueen, J., 1967. Some Methods for classification and
Analysis of Multivariate Observations. 5th Berkeley
Symposium on Mathematical Statistics and Probability
1967, 1(233), pp.281–297. Available at:
http://projecteuclid.org/euclid.bsmsp/1200512992.
Nguyen, H.L., Woon, Y.K. and Ng, W.K., 2015. A survey
on data stream clustering and classification.
Knowledge and Information Systems, pp.535–569.
Available at: http://dx.doi.org/10.1007/s10115-014-
0808-1.
Ntoutsi, I., Spiliopoulou, M. and Theodoridis, Y., 2009.
Tracing cluster transitions for different cluster types.
Control and Cybernetics, 38(1), pp.239–259.
Oliveira, M. and Gama, J., 2012. A Framework to Monitor
Clusters’ Evolution Applied to Economy and Finance
Problems. Intell. Data Anal. 16, 1, 93-111.
Olshannikova, E., Ometov, A. and Koucheryavy, Y.,
2014. Towards Big Data Visualization for Augmented
Reality. 2014 IEEE 16th Conference on Business
Informatics, pp.33–37. Available at:
http://ieeexplore.ieee.org/lpdocs/epic03/wrapper.htm?
arnumber=6904299.
Pio, G., Lanotte, P. F., Ceci, M. and Malerba, D., 2014.
Mining Temporal Evolution of Entities in a Stream of
Textual Documents. Springer International Publishing
Switzerland 2014, pp.50–60.
Silva, J., Faria, E., Barros, R., Hruschka, E. and Carvalho,
A., 2013. Data Stream Clustering : A Survey. ACM
Computing Surveys (CSUR), pp.1–37.
Yogita and Toshniwal, D., 2012. Clustering Techniques
for Streaming Data – A Survey. , pp.951–956.
Zhang, T., Ramakrishnan, R. and Livny, M., 1996.
BIRCH: An Efficient Data Clustering Databases
Method for Very Large Databases. ACM SIGMOD
International Conference on Management of Data, 1,
pp.103–114.
APPENDIX 1
The following tables show the details and specify
the distribution of each of the three synthesized
datasets.
Table 1: Parameters details of DS1.
Table 2: Parameters details of DS2.
ICPRAM 2017 - 6th International Conference on Pattern Recognition Applications and Methods
182