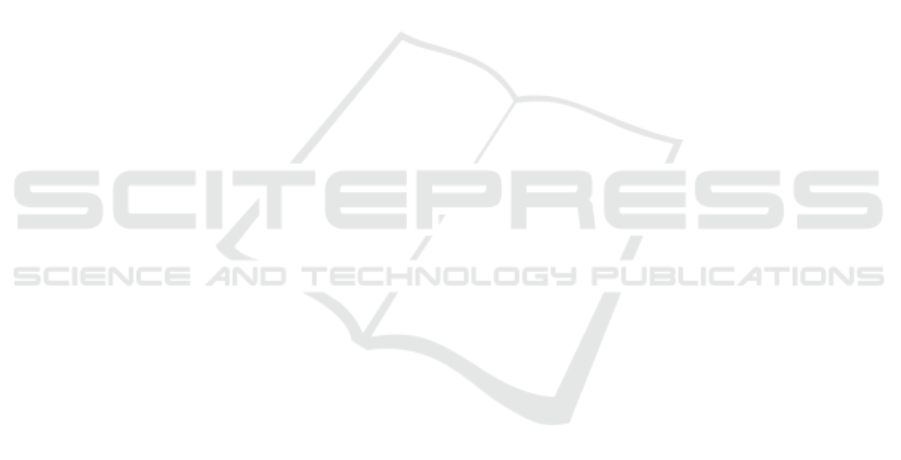
agreement combined. We think that GBVS scores
that good, because of its graph based approach which
may correlate to human eye movements. Therefore
the question how binarization can positively affect
saliency detection methods poses to be interesting and
needs to be investigated.
Unfortunately a combination of two saliency methods
didn’t yield much improvements. Nevertheless the
combinations containing GBVS(M4) often resulted in
the best scores.
These results are very interesting and can be used for
many different applications, such as developing a bet-
ter sentiment classifier for ANPs using salient regions
for feature extractions.
REFERENCES
Al-Naser, M., Chanijani, S. S. M., Bukhari, S. S., Borth,
D., and Dengel, A. (2015). What makes a beautiful
landscape beautiful: Adjective noun pairs attention by
eye-tracking and gaze analysis. In Proceedings of the
1st International Workshop on Affect & Sentiment in
Multimedia, pages 51–56. ACM.
Borji, A., Sihite, D. N., and Itti, L. (2013). Quantitative
analysis of human-model agreement in visual saliency
modeling: a comparative study. IEEE Transactions on
Image Processing, 22(1):55–69.
Borth, D., Ji, R., Chen, T., Breuel, T., and Chang, S.-F.
(2013). Large-scale visual sentiment ontology and de-
tectors using adjective noun pairs. In Proceedings of
the 21st ACM international conference on Multime-
dia, pages 223–232. ACM.
Fang, Y., Chen, Z., Lin, W., and Lin, C.-W. (2011).
Saliency-based image retargeting in the compressed
domain. In Proceedings of the 19th ACM international
conference on Multimedia, pages 1049–1052. ACM.
Goferman, S., Zelnik-manor, L., and Tal, A. (2010).
Context-aware saliency detection. In in [IEEE Conf.
on Computer Vision and Pattern Recognition.
Harel, J., Koch, C., and Perona, P. (2006). Graph-based vi-
sual saliency. In Advances in neural information pro-
cessing systems, pages 545–552.
Itti, L. and Koch, C. (2000). A saliency-based search mech-
anism for overt and covert shifts of visual attention.
Vision research, 40(10):1489–1506.
Judd, T., Durand, F., and Torralba, A. (2012). A benchmark
of computational models of saliency to predict human
fixations.
Mancas, M. (2009). Relative influence of bottom-up and
top-down attention, attention in cognitive systems: 5th
international workshop on attention in cognitive sys-
tems, wapcv 2008 fira, santorini, greece, may 12, 2008
revised selected papers.
Mancas, M., Couvreur, L., Gosselin, B., Macq, B., et al.
(2007). Computational attention for event detection.
In Proc. Fifth Intl Conf. Computer Vision Systems.
Mancas, M., Mancas-Thillou, C., Gosselin, B., and Macq,
B. (2006). A rarity-based visual attention map - ap-
plication to texture description. In 2006 International
Conference on Image Processing, pages 445–448.
Otsu, N. (1975). A threshold selection method from gray-
level histograms. Automatica, 11(285-296):23–27.
Riche, N., Mancas, M., Duvinage, M., Mibulumukini, M.,
Gosselin, B., and Dutoit, T. (2013). Rare2012: A
multi-scale rarity-based saliency detection with its
comparative statistical analysis. Signal Processing:
Image Communication, 28(6):642–658.
Vikram, T. N., Tscherepanow, M., and Wrede, B. (2011).
A random center surround bottom up visual attention
model useful for salient region detection. In Applica-
tions of Computer Vision (WACV), 2011 IEEE Work-
shop on, pages 166–173. IEEE.
Zhang, L., Gu, Z., and Li, H. (2013). Sdsp: A novel saliency
detection method by combining simple priors. In 2013
IEEE International Conference on Image Processing,
pages 171–175. IEEE.
Which Saliency Detection Method is the Best to Estimate the Human Attention for Adjective Noun Concepts?
189