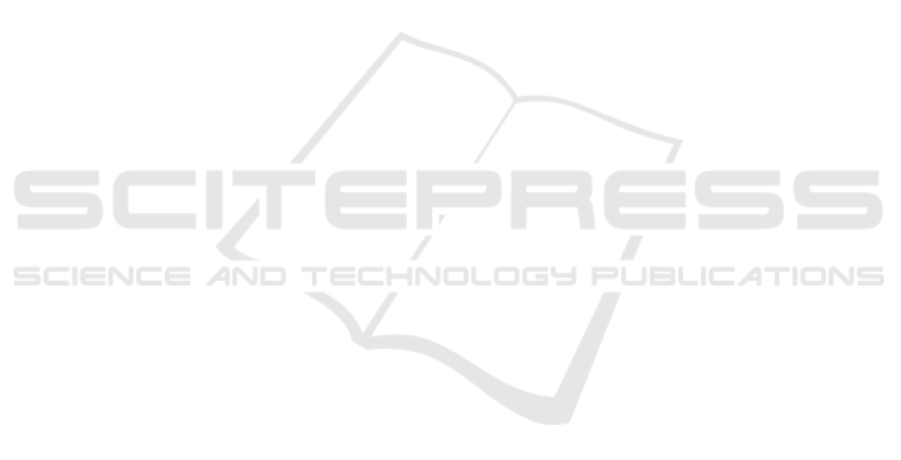
Lin, W.-C., Tsai, C.-F., Chen, Z.-Y., and Ke, S.-W. (2016).
Keypoint selection for efficient bag-of-words feature
generation and effective image classification. Infor-
mation Sciences, 329:33–51.
Liu, J., Ali, S., and Shah, M. (2008). Recognizing human
actions using multiple features. In Computer Vision
and Pattern Recognition, 2008. CVPR 2008. IEEE
Conference on, pages 1–8. IEEE.
Mairal, J., Ponce, J., Sapiro, G., Zisserman, A., and Bach,
F. R. (2009). Supervised dictionary learning. In
Advances in neural information processing systems,
pages 1033–1040.
Negin, F. and Bremond, F. (2016). Human action recogni-
tion in videos: A survey.
Nowak, E., Jurie, F., and Triggs, B. (2006). Sampling strate-
gies for bag-of-features image classification. In Euro-
pean conference on computer vision, pages 490–503.
Springer.
Olshausen, B. A. and Field, D. J. (1997). Sparse coding
with an overcomplete basis set: A strategy employed
by v1? Vision research, 37(23):3311–3325.
Poppe, R. (2010). A survey on vision-based human action
recognition. Image and vision computing, 28(6):976–
990.
Sapienza, M., Cuzzolin, F., and Torr, P. H. (2014). Learning
discriminative space–time action parts from weakly
labelled videos. International Journal of Computer
Vision, 110(1):30–47.
Schuldt, C., Laptev, I., and Caputo, B. (2004). Recogniz-
ing human actions: a local svm approach. In Pat-
tern Recognition, 2004. ICPR 2004. Proceedings of
the 17th International Conference on, volume 3, pages
32–36. IEEE.
Tran, D., Bourdev, L., Fergus, R., Torresani, L., and Paluri,
M. (2015). Learning spatiotemporal features with 3d
convolutional networks. In 2015 IEEE International
Conference on Computer Vision (ICCV), pages 4489–
4497. IEEE.
Verma, Y. and Jawahar, C. (2016). A robust distance with
correlated metric learning for multi-instance multi-
label data. In Proceedings of the 2016 ACM on Multi-
media Conference, pages 441–445. ACM.
Wang, H., Kl
¨
aser, A., Schmid, C., and Liu, C.-L. (2011).
Action recognition by dense trajectories. In Computer
Vision and Pattern Recognition (CVPR), 2011 IEEE
Conference on, pages 3169–3176. IEEE.
Wang, H., Ullah, M. M., Klaser, A., Laptev, I., and Schmid,
C. (2009). Evaluation of local spatio-temporal fea-
tures for action recognition. In BMVC 2009-British
Machine Vision Conference, pages 124–1. BMVA
Press.
Wang, J., Yang, J., Yu, K., Lv, F., Huang, T., and Gong,
Y. (2010). Locality-constrained linear coding for
image classification. In Computer Vision and Pat-
tern Recognition (CVPR), 2010 IEEE Conference on,
pages 3360–3367. IEEE.
Wang, J. and Zucker, J.-D. (2000). Solving multiple-
instance problem: A lazy learning approach.
Wang, X., Wang, B., Bai, X., Liu, W., and Tu, Z. (2013).
Max-margin multiple-instance dictionary learning. In
ICML (3), pages 846–854.
Wang, X., Wang, L., and Qiao, Y. (2012). A comparative
study of encoding, pooling and normalization meth-
ods for action recognition. In Asian Conference on
Computer Vision, pages 572–585. Springer.
Weinland, D., Ronfard, R., and Boyer, E. (2011). A sur-
vey of vision-based methods for action representation,
segmentation and recognition. Computer vision and
image understanding, 115(2):224–241.
Zhang, C., Platt, J. C., and Viola, P. A. (2005). Multiple
instance boosting for object detection. In Advances in
neural information processing systems, pages 1417–
1424.
Zhang, D., Wang, F., Si, L., and Li, T. (2009). M3ic: Max-
imum margin multiple instance clustering. In IJCAI,
volume 9, pages 1339–1344.
Zhou, Y., Ni, B., Hong, R., Wang, M., and Tian, Q. (2015).
Interaction part mining: A mid-level approach for
fine-grained action recognition. In Proceedings of
the IEEE Conference on Computer Vision and Pattern
Recognition, pages 3323–3331.
Zhou, Z.-H. (2004). Multi-instance learning: A survey. De-
partment of Computer Science & Technology, Nanjing
University, Tech. Rep.
Zhu, J., Wang, B., Yang, X., Zhang, W., and Tu, Z. (2013).
Action recognition with actons. In Proceedings of the
IEEE International Conference on Computer Vision,
pages 3559–3566.
ICPRAM 2017 - 6th International Conference on Pattern Recognition Applications and Methods
526