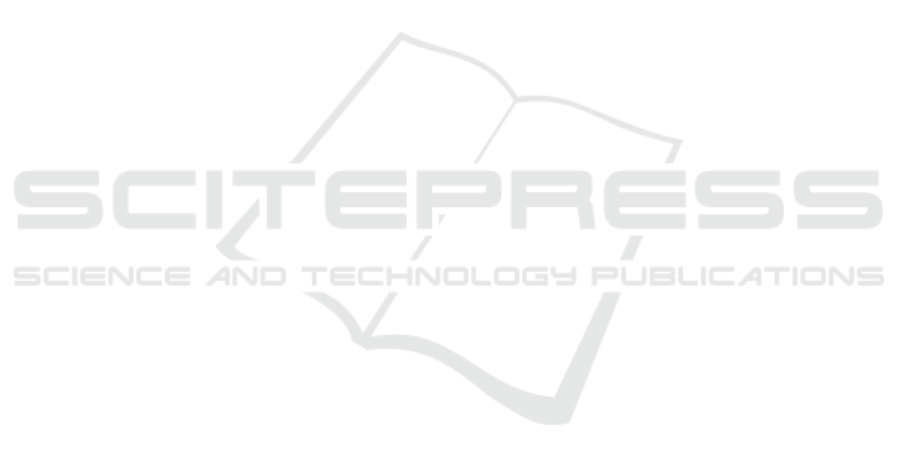
duction of the number of features selected are mixed,
when using Inconsistent Examples the number of fea-
tures seems to grow using started methods while when
using the Wrapper and Mutual Information measures,
the largest reduction of selected features is often car-
ried out by some started search methods.
As future work, we hope that this contribution will
open new opportunities for researching improvements
on many feature selection methods and that being on
a systematized way that may lead to many different
proposals but in a well organized development frame.
ACKNOWLEDGMENTS
This research is partially supported by projects:
TIN2013-47210-P of the Ministerio de Econom
´
ıa y
Competitividad (Spain), P12-TIC-2958 and TIC1582
of the Consejeria de Economia, Innovacion, Ciencia
y Empleo from Junta de Andalucia (Spain).
REFERENCES
Amjady, N. and Daraeepour, A. (2009). Mixed price and
load forecasting of electricity markets by a new iter-
ative prediction method. Electric power systems re-
search, 79(9):1329–1336.
Arauzo-Azofra, A., Aznarte, J. L., and Ben
´
ıtez, J. M.
(2011). Empirical study of feature selection meth-
ods based on individual feature evaluation for classi-
fication problems. Expert Systems with Applications,
38(7):8170 – 8177.
Arauzo-Azofra, A., Benitez, J. M., and Castro, J. L. (2008).
Consistency measures for feature selection. Journal
of Intelligent Information Systems, 30(3):273–292.
Blum, A. L. and Langley, P. (1997). Selection of relevant
features and examples in machine learning. Artificial
Intelligence, 97(1-2):245–271.
Dem
ˇ
sar, J., Curk, T., Erjavec, A.,
ˇ
Crt Gorup, Ho
ˇ
cevar, T.,
Milutinovi
ˇ
c, M., Mo
ˇ
zina, M., Polajnar, M., Toplak,
M., Stari
ˇ
c, A.,
ˇ
Stajdohar, M., Umek, L.,
ˇ
Zagar, L.,
ˇ
Zbontar, J.,
ˇ
Zitnik, M., and Zupan, B. (2013). Orange:
Data mining toolbox in python. Journal of Machine
Learning Research, 14:2349–2353.
Duda, R. O., Hart, P. E., and Stork, D. G. (2000). Pattern
Classification (2Nd Edition). Wiley-Interscience.
Kohavi, R. (1994). Feature Subset Selection as Search with
Probabilistic Estimates.
Kohavi, R. and John, G. H. (1997). Wrappers for feature
subset selection. Artificial Intelligence, 97:273–324.
Kononenko, I. (1994). Estimating attributes: Analysis and
extensions of relief. In Proceedings of the European
Conference on Machine Learning on Machine Learn-
ing, ECML-94, pages 171–182, Secaucus, NJ, USA.
Springer-Verlag New York, Inc.
Liu, H. and Yu, L. (2005). Toward integrating feature selec-
tion algorithms for classification and clustering. IEEE
Trans. on Knowl. and Data Eng., 17(4):491–502.
Newman, C. B. D. and Merz, C. (1998). UCI repository of
machine learning databases.
Polat, K. and G
¨
unes, S. (2009). A new feature selection
method on classification of medical datasets: Ker-
nel f-score feature selection. Expert Syst. Appl.,
36(7):10367–10373.
Schiffner, J., Bischl, B., Lang, M., Richter, J., Jones, Z. M.,
Probst, P., Pfisterer, F., Gallo, M., Kirchhoff, D.,
K
¨
uhn, T., Thomas, J., and Kotthoff, L. (2016). mlr
Tutorial. ArXiv e-prints.
Tang, J., Alelyani, S., and Liu, H. (2014). Feature Selec-
tion for Classification: A Review. In Data Classifica-
tion, Chapman & Hall/CRC Data Mining and Knowl-
edge Discovery Series, pages 37–64. Chapman and
Hall/CRC.
Thangavel, K. and Pethalakshmi, A. (2009). Dimension-
ality reduction based on rough set theory: A review.
Applied Soft Computing, 9(1):1 – 12.
Vergara, J. R. and Est
´
evez, P. A. (2015). A review of fea-
ture selection methods based on mutual information.
CoRR, abs/1509.07577.
ICAART 2017 - 9th International Conference on Agents and Artificial Intelligence
614