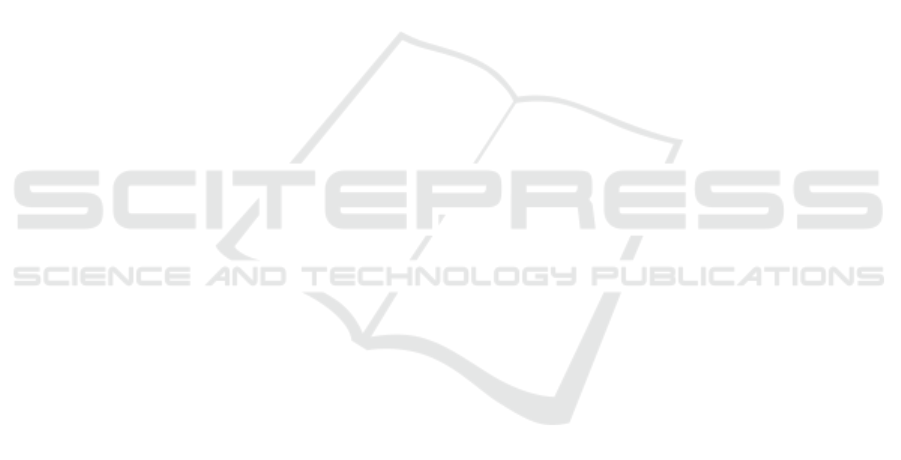
print class recognition rate in comparaison with 80%
for the ISO template. We have improved the recogni-
tion rate around 10%. In our case of study, an EMV
transaction, we have two approach and we have to
choose between more computation time, ressources
but 89% of recognition rate and few ressources and
quicker approach based on ISO template but only with
80% of fingerprint class recognition. We have shown
when one adds fingerprint recognition module, both
on smartcard and Point of sales we help to secure
EMV transaction when biometric is taking into ac-
count.
As perspectives, we plan to improve these recog-
nition rates of the fingerprint class by using other fea-
tures. And also to test in real condition on SE these
approach.
REFERENCES
Alok, A. K., Saha, S., and Ekbal, A. (2015). Multi-objective
semi-supervised clustering for automatic pixel classi-
fication from remote sensing imagery. Soft Comput-
ing, pages 1–19.
Aurenhammer, F. (1991). Voronoi diagramsa survey of a
fundamental geometric data structure. ACM Comput-
ing Surveys (CSUR), 23(3):345–405.
Chang, C.-C. and Lin, C.-J. (2011). Libsvm: A library for
support vector machines. ACM Transactions on Intel-
ligent Systems and Technology (TIST), 2(3):27.
Charrier, C., L
´
ezoray, O., and Lebrun, G. (2012). A
machine learning regression scheme to design a fr-
image quality assessment algorithm. In Conference
on Colour in Graphics, Imaging, and Vision, volume
2012, pages 35–42. Society for Imaging Science and
Technology.
Elguebaly, T. and Bouguila, N. (2015). A hierarchical
nonparametric bayesian approach for medical images
and gene expressions classification. Soft Computing,
19(1):189–204.
EMVCo (2008). EMV integrated circuit card specifications
for payment systems. Technical report, EMVCo.
Fi
´
errez-Aguilar, J., Nanni, L., Ortega-Garcia, J., Cappelli,
R., and Maltoni, D. (2005). Combining multiple
matchers for fingerprint verification: a case study in
fvc2004. In International Conference on Image Anal-
ysis and Processing, pages 1035–1042. Springer.
Fiore, U., Palmieri, F., Castiglione, A., and De Santis, A.
(2013). Network anomaly detection with the restricted
boltzmann machine. Neurocomputing, 122:13–23.
Gopi, M., Krishnan, S., and Silva, C. T. (2000). Surface
reconstruction based on lower dimensional localized
delaunay triangulation. In Computer Graphics Forum,
volume 19, pages 467–478.
Hsu, C.-W. and Lin, C.-J. (2002). A comparison of meth-
ods for multiclass support vector machines. Neural
Networks, IEEE Transactions on, 13(3):415–425.
ISO (2011). ISO/IEC 19794-2. information technology -
biometric data interchange format format - part 2 :
Finger minutiae data, 2011.
Jain, A. and Pankanti, S. (2000). Fingerprint classification
and matching. Handbook for Image and Video Pro-
cessing.
Jain, A. K., Prabhakar, S., , and Hong, L. (1999a). A multi-
channel approach to fingerprint classification. In Pat-
tern Analysis and Machine Intelligence, IEEE Trans-
actions on, volume 24, pages 248–359.
Jain, A. K., Prabhakar, S., and Hong, L. (1999b). A mul-
tichannel approach to fingerprint classification. Pat-
tern Analysis and Machine Intelligence, IEEE Trans-
actions on, 21(4):348–359.
Jayaraman, U., Gupta, A. K., and Gupta, P. (2014). An effi-
cient minutiae based geometric hashing for fingerprint
database. Neurocomputing, 137:115–126.
Kudo, M. and Sklansky, J. (2000). Comparison of algo-
rithms that select features for pattern classifiers. Pat-
tern Recognition, 33(1):25–41.
Kumar, M. et al. (2014). A novel fingerprint minutiae
matching using lbp. In Reliability, Infocom Technolo-
gies and Optimization (ICRITO)(Trends and Future
Directions), 2014 3rd International Conference on,
pages 1–4. IEEE.
Kumar, S. U. and Inbarani, H. H. (2016). Neighborhood
rough set based ecg signal classification for diagnosis
of cardiac diseases. Soft Computing, pages 1–13.
Labatut, P., Pons, J.-P., and Keriven, R. (2007). Efficient
multi-view reconstruction of large-scale scenes using
interest points, delaunay triangulation and graph cuts.
In Computer Vision, 2007. ICCV 2007. IEEE 11th In-
ternational Conference on, pages 1–8. IEEE.
Li, J., Du, Q., and Li, Y. (2015). An efficient radial ba-
sis function neural network for hyperspectral remote
sensing image classification. Soft Computing, pages
1–7.
Maio, D., Maltoni, D., Cappelli, R., Wayman, J. L., and
Jain, A. K. (2004). Fvc2004: Third fingerprint ver-
ification competition. In Biometric Authentication,
pages 1–7. Springer.
Oehlmann, L., Huckemann, S., and Gottschlich, C. (2015).
Performance evaluation of fingerprint orientation field
reconstruction methods. In Biometrics and Forensics
(IWBF), 2015 International Workshop on, pages 1–6.
IEEE.
Palmieri, F., Fiore, U., and Castiglione, A. (2014). A dis-
tributed approach to network anomaly detection based
on independent component analysis. Concurrency and
Computation: Practice and Experience, 26(5):1113–
1129.
Palmieri, F., Fiore, U., Castiglione, A., and De Santis,
A. (2013). On the detection of card-sharing traf-
fic through wavelet analysis and support vector ma-
chines. Applied Soft Computing, 13(1):615–627.
Park, J., Bhuiyan, M. Z. A., Kang, M., Son, J., and Kang, K.
(2016). Nearest neighbor search with locally weighted
linear regression for heartbeat classification. Soft
Computing, pages 1–12.
Roy, B. R. and Trivedi, A. K. (2014). Construction of fin-
gerprint orientation field from minutia points. In Ad-
Fingerprint Class Recognition for Securing EMV Transaction
409