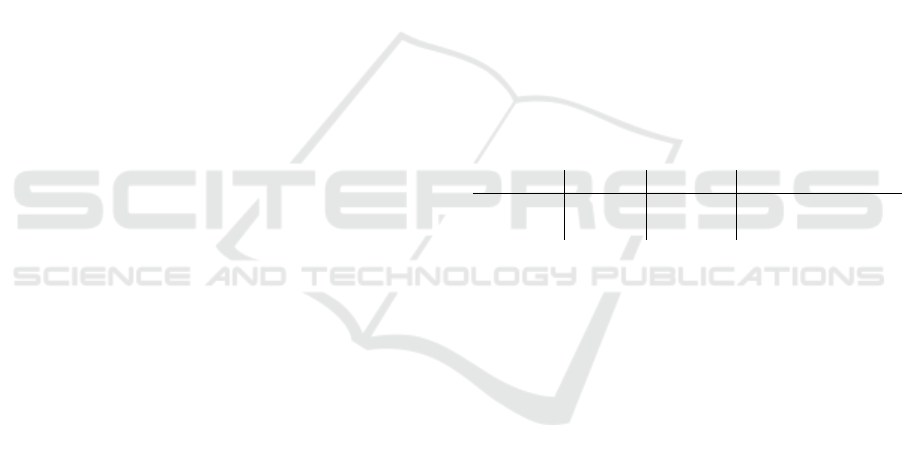
• nAreas: 10–20, step size 5,
• minRadius: 0.01–0.19, step size 0.02,
• toleranceFactor: 1.1–2.1, step size 0.2,
• distributionType: uniform and normal.
As a result, 360 different parameter sets are used
to evaluate the CHnMM authentication system. Ad-
ditionally, to test the influence of a different number
of training examples, the experiments have been con-
ducted with either five or ten training examples per
user. Consequently, for each corpus (MCYT, Doo-
dles, PseudoSignatures) and forgery data type (ran-
dom or skilled) 360 ∗ 2 FAR-FRR pairs are calcu-
lated. Plotting these results in a FAR-FRR point di-
agram helps to interpret the results. This diagram
must not be confused with the so-called Receiver Op-
erating Characteristic (ROC) curve although it is very
similar. The ROC curve is commonly used to visual-
ize the behaviour of a verification system but in this
work, there is currently no single threshold parameter
implemented.
To reduce the load of the immense amount of cal-
culations, the employed data sets were limited to 25
users for the parameter variation experiments. Only
for particular parameter sets, the complete data set
was utilised. All experiments were conducted on a
common modern laptop (Intel Core i5 - 5200U, 8GB
RAM).
4.3 Results
4.3.1 Result Overview
The outcome of the previously explained experiments
is visually summarized in Figure 3 with an FAR-FRR
point diagram for every corpus. The visual impres-
sion very much resembles a typical ROC curve espe-
cially if a Pareto frontier is imagined. The main dif-
ference is that there are also points behind the Pareto
frontier, which represent results of experiments where
an unsuitable parameter set was employed. Hence,
the general behaviour is as expected, because trying
to reduce the FRR produces higher FAR and vice
versa. Also as expected is the performance differ-
ence between random (circles) and skilled (crosses)
forgeries as most experiment outcomes for the ran-
dom approach are very close to a FAR of 0, especially
compared to the skilled forgery approach.
Comparing the different data sets, the best perfor-
mance was achieved with MCYT signatures, where
also the distance between random and skilled is rather
small compared to doodles and pseudo-signatures.
This is probably due to the fact that signatures writ-
ten with a pen are performed more consistently, since
they are a common and known movement for the
user. For the same reason, the pseudo-signature re-
sults are slightly better than for the doodles, but since
the pseudo-signatures are performed with a finger on
a touch-screen they are not as consistent as the signa-
tures.
Another unsurprising observation is that increas-
ing the number of training examples from five (yel-
low) to ten (blue) generally improves the perfor-
mances on all databases. However, this also indicates
that the developed system works as expected.
In Table 1 the achieved EER for each data set and
forgery type is displayed. Be aware that in this work
these EER values describe the best achievable bal-
anced (FAR equals FRR) result by using a good pa-
rameter set. The values do not recommend to use the
system in practice, especially due to the quite high
percentages for the random forgeries that seemingly
suggest that not even random input can be distin-
guished well, but the plots prove that the system has a
very low FAR until the parameter sets become too tol-
erant. Hence, in order to better understand the values
they have to be compared to other methods.
Table 1: Achieved EER for every database.
MCYT Doodles PseudoSignatures
Random 4% 12% 8%
Skilled 11% 29% 21%
The work by Martinez-Diaz et al.(Martinez-Diaz
et al., 2013) contains benchmark values for the Doo-
dles and Pseudo-signatures corpora that are based on
a DTW verification approach. Fortunately, they em-
ployed very basic DTW approaches that only use
the x,y-coordinates or their first or second derivative.
This allows for a fair comparison, because these fea-
tures are not application specific but very generic as
is our system that is not designed for specific trajec-
tories. Their results are based on experiments with
5 training examples, and with skilled forgeries they
achieved EER between 26.7%–36.4% for doodles and
between 19.8%–34.5% for Pseudo-signatures. For
random forgeries the EER are between 2.7%–7.6%
for doodles and between 1.6%–5.0% for Pseudo-
signatures.
In the work by Ortega-Garcia et al. (Ortega-
Garcia et al., 2003) an HMM verification approach
was applied to subsets of the MCYT database where
models were trained using 10 training examples. De-
pending on the chosen subset, EER between 1% and
3% were achieved for skilled forgeries. While this
value could not be achieved with our system, we still
think that the performance is very promising, espe-
cially considering that it is not specialized on signa-
Evaluating a New Conversive Hidden non-Markovian Model Approach for Online Movement Trajectory Verification
255