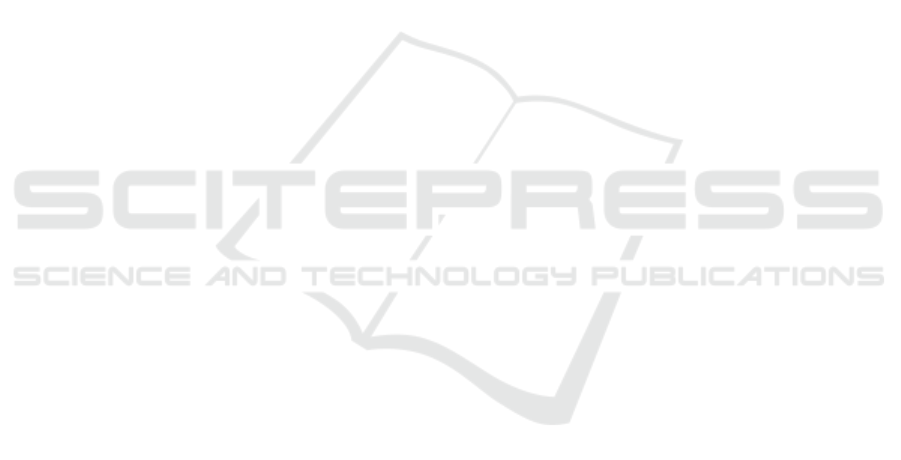
that these features achieve a high detection ratio
(Yang et al., 2011). Also the IP-address a tweet is
sent from, can be valuable information. This infor-
mation is not available to the public, but can be imple-
mented by Twitter itself. Furthermore, the approach
can be improved by checking if the URL is listed on
blacklists. N.S. Gawale and N.N. Patile already im-
plemented a system to successfully detect malicious
URLs on Twitter (Gawale and Patil, 2015). Twitter
also lends itself for network features, such as number
of followers, user distance and mutual links. How-
ever, Twitter does not offer a way to retrieve historical
data about changes in these network features, so such
an extension could only be developed and evaluated
by monitoring a large set of users ‘hoping’ that they
will get hacked. Finally, features based ontext anal-
ysis may have potential, because malicious tweets,
spam tweets in particular, use very striking and sus-
picious sentences.
REFERENCES
Ablon, L., Libicki, M. C., and Golay, A. A. (2014). Mar-
kets for Cybercrime Tools and Stolen Data: Hackers’
Bazaar. RAND Corporation, Santa Monica, CA.
Aggarwal, A. and Kumaraguru, P. (2015). What they do in
shadows: Twitter underground follower market.
Amleshwaram, A., Reddy, N., Yadav, S., Gu, G., and Yang,
C. (2013). Cats: Characterizing automation of twitter
spammers.
Benevenuto, F., Magno, G., Rodrigues, T., and Almeida, V.
(2010). Detecting spammers on twitter.
Beniwal, S. and Arora, J. (2012). Classification and feature
selection techniques in data mining. IJERT, 1(6).
Chen, C., Zhang, J., Chen, X., Xiang, Y., and Zhou, W.
(2015). 6 million spam tweets: A large ground truth
for timely twitter spam detection.
Demidova, N. (2014). Social network frauds.
https://securelist.com/analysis/publications/63855/ social-network-frauds/
.
Egele, M., Stringhini, G., Kruegel, C., and Vigna, G.
(2013). Compa: Detecting compromised accounts on
social networks.
Gawale, N. and Patil, N. (2015). Implementation of a sys-
tem to detect malicious urls for twitter users.
Google. Security notification settings, alerts for new sign-
ins.
https://support.google.com/accounts/answer/2733203
, Last vis-
ited: June 18th 2016.
Hall, M. and Holmes, G. (2003). Benchmarking attribute
selection techniques for discrete class data mining.
IEEE Transactions on Knowledge and Data Engineer-
ing, 15(6):1437–1447.
Hall, M. A. (1999). Correlation-based feature selection
for machine learning. PhD thesis, The University of
Waikato.
Iguyon, I. and Elisseeff, A. (2003). An introduction to vari-
able and feature selection. Journal of Machine Learn-
ing Research, 3:1157–1182.
McCord, M. and Chuah, M. (2011). Spam detection on
twitter using traditional classifiers.
Mei, Y., Zhang, Z., Zhao, W., Yang, J., and Nugroho, R.
(2015). A hybrid feature selection method for predict-
ing user influence on twitter.
Moore, H. and Roberts, D. (2013). Ap twit-
ter hack causes panic on wall street and sends
dow plunging.
http://www.theguardian.com/business/2013/apr/23/
ap-tweet-hack-wall-street-freefall
, 23th April 2013.
Platt, J. C. (1999). Fast training of support vector machines
using sequential minimal optimization. In Advances
in kernel methods, pages 185–208. MIT Press Cam-
bridge. ISBN 0-262-19416-3.
Sang, E. and Van Den Bosch, A. (2013). Dealing with big
data: The case of twitter. Computational Linguistics
in the Netherlands Journal, 3:121–134.
Thomas, K., Grier, C., Song, D., and Paxson, V. (2011).
Suspended accounts in retrospect: An analysis of twit-
ter spam. Proc. of IMC 2011, pages 243–258.
Thomas, K., Li, F., Grier, C., and Paxson, V. (2014). Con-
sequences of connectivity: Characterizing account hi-
jacking on twitter.
Twitter (2016a). Reporting spam on twitter.
https://support.twitter.com/articles/64986
, Last visited: May
9th 2016.
Twitter (2016b). Tweets field guide, developers documen-
tation overview.
https://dev.twitter.com/overview/api/tweets
, Last
visited: May 13th 2016.
Whittaker, Z. (2016). A hacker claims to be
selling millions of twitter accounts. ZD-
Net,
http://www.zdnet.com/article/twitter-32-million-
credentials-accounts-selling-online/
, June 9th 2016.
Yang, C., Harkreader, R., and Gu, G. (2011). Die free or
live hard? empirical evaluation and new design for
fighting evolving twitter spammers.
Yardi, S., Romero, D., Schoenebeck, G., and Boyd, D.
(2010). Detecting spam in a twitter network. First
Monday, 15(1).
Youn, S. and McLeod, D. (2007). A comparative study for
email classification. In Advances and Innovations in
Systems, Computing Sciences and Software Engineer-
ing, pages 387–391. Springer.
Zangerle, E. and Specht, G. (2014). ”sorry, i was hacked” a
classification of compromised twitter accounts. Proc.
of ACM SAC 2014, pages 587–593.
Detecting Hacked Twitter Accounts based on Behavioural Change
31