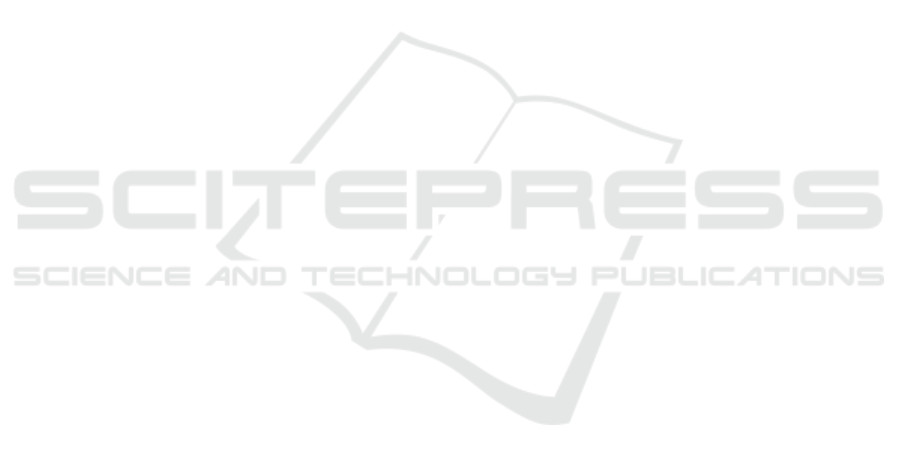
mechanisms. To this aim, specific experimental tasks
have been developped. The proposed experimental
platform is equipped with an eye-tracking system to
collect specific eye movement data. Despite the gap
between the real and the virtual world, VR is an inter-
esting compromise between highly controlled experi-
mental situations and studies undertaken in a natural
setting. Indeed, VR makes it possible to associate a
controlled study of cognitive processes with situations
closer to everyday life by enabling an interaction with
the environment. In future, we plan to add more sen-
sors to get additional data such as physiological data
(EMG, ECG, and GSR) for a more in-depth analysis
of users behavior and performance.
REFERENCES
Ahlstrom, C., Victor, T., Wege, C., and Steinmetz, E.
(2012). Processing of eye/head tracking data in large-
scale naturalistic driving data sets. IEEE Transactions
on Intelligent Transportation Systems, 13(2):553–564.
Aoki, H., Nakayasu, H., Kondo, N., and Miyoshi, T. (2010).
Cognitive study on drivers behavior by vehicle trajec-
tory and eye movement in virtual environment. In Pro-
ceedings of the 3
rd
International Conference on Ap-
plied Human Factors and Ergonomics, pages 6768–
6777.
Artz, B., Cathey, L., Grant, P., Houston, D., and Greenberg,
J. (2001). The design and construction of the visual
subsystem for virttex, the driving simulator at the ford
research laboratories. In Proceedings of Driving Sim-
ulation Conference, pages 69–79.
Auberlet, J., Pacaux, M., Anceaux, F., Plainchault, P., and
Rosey, F. (2010). The impact of perceptual treat-
ments on lateral control: A study using fixed-base and
motion-base driving simulators. Accident Analysis &
Prevention, 42(1):166–173.
Bian, D., Wade, J., Swanson, A., Warren, Z., and Sarkar,
N. (2015). Physiology-based affect recognition during
driving in virtual environment for autism intervention.
In Proceedings of the 2Nd International Conference
on Physiological Computing Systems, PhyCS 2015,
pages 137–145, Portugal. SCITEPRESS - Science and
Technology Publications, Lda.
Bian, D., Wade, J. W., Zhang, L., Bekele, E., Swanson,
A., Crittendon, J. A., Sarkar, M., Warren, Z., and
Sarkar, N. (2013). A novel virtual reality driving en-
vironment for autism intervention. In Proceedings
of the 7th International Conference on Universal Ac-
cess in Human-Computer Interaction: User and Con-
text Diversity - Volume 2, UAHCI’13, pages 474–483,
Berlin, Heidelberg. Springer-Verlag.
Blana, E. and Golias, J. (2002). Differences between vehi-
cle lateral displacement on the road and in a fixed-base
simulator. Human Factors, 44:303–313.
Bl
¨
attler, C., Ferrari, V., Didierjean, A., and Marm
`
eche, E.
(2012). Role of expertise and action in motion ex-
trapolation from real road scenes. Visual Cognition,
20(9):988 – 1011.
Breidenbach, C. and Tomaske, W. (2004). The use of
driving simulators in simulating the on-road drive of
wheeled vehicles. In Proceedings of Driving Simula-
tion Conference, pages 333–341.
Burnham, B. R., Sabia, M., and Langan, C. (2014). Com-
ponents of working memory and visual selective at-
tention. Journal of Experimental Psychology: Human
Perception and Performance, 40(1):391–403.
Crundall, D., Chapman, P., and Underwood, G. (2003). Eye
movements and hazard perception in police pursuit
and emergency response driving. Journal of Exper-
imental Psychology: Applied, 9:163–174.
Freyd, J. J. and Finke, R. A. (1984). Representational
momentum. Journal of Experimental Psychology:
Learning, Memory and Cognition, 10:126 – 132.
Galpin, A., Underwood, G., and Crundall, D. (2009).
Change blindness in driving scenes. Transportation
Research (Part F), 12:179–185.
Grabe, V., Pretto, P., Giordano, P. R., and Bultho, H. H.
(2010). Infuence of display type on drivers perfor-
mance in a motion based driving simulator. In Pro-
ceedings of Driving Simulation Conference, pages 81–
88.
Graydon, F. X., Young, R., Benton, M. D., Genik, R., Posse,
S., Hsieh, L., and Green, C. (2004). Visual event de-
tection during simulated driving: Identifying the neu-
ral correlates with functional neuroimaging. Trans-
portation Research (Part F), 7:271–286.
Greenberg, J., Curry, R., Blommer, M., Kozak, K., Artz, B.,
Cathey, L., and Kao, B. (2006). The validity of last-
second braking and steering judgments in advanced
driving simulators. In Proceedings of Driving Simula-
tion Conference, pages 143–153.
Haines, A. (2011). The carrs-q advanced driving simulator.
In Road Safety Forum 2011: Directions in Road Safety
Research.
Hale, R. G., Brown, J., and McDunn, B. A. (2016). Increas-
ing task demand by obstructing object recognition in-
creases boundary extension. Psychonomic Bulletin &
Review, 23:1497–1503.
Holmqvist, K., Nystrom, M., Andersson, R., Dewhurst, R.,
Jarodzka, H., and van de Weijer, J. (2011). Eye Track-
ing: A comprehensive guide to methods and measures.
Oxford University Press, Oxford, United-Kingdom.
Intraub, H. and Richardson, M. (1989). Wide-angle memo-
ries of close-up scenes. Journal of Experimental Psy-
chology: Learning, Memory and Cognition, 15:179–
196.
Jacob, R. (1991). The use of eye movements in human-
computer interaction techniques: what you look at is
what you get. ACM Transactions on Information Sys-
tems (TOIS), 9(2):152–169.
Lavie, N. (2010). Attention, distraction and cognitive con-
trol under load. Journal of Eye Movement Research,
19(3):143–148.
Lavie, N. and Fockert, J. D. (2005). The role of working
memory in attentional capture. Psychonomic Bulletin
& Review, 12:669674.
HUCAPP 2017 - International Conference on Human Computer Interaction Theory and Applications
144