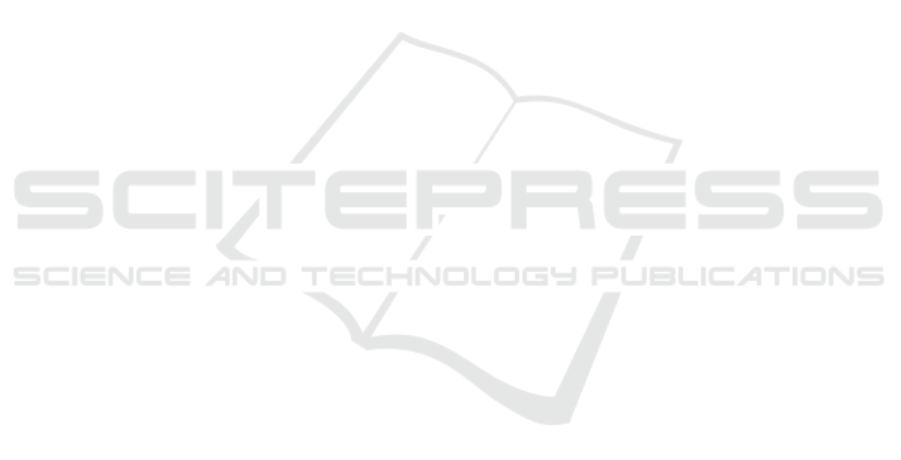
uation results of our algorithm and state-of-the-art al-
gorithms using different serendipity metrics.
SOG is based on the topic diversification (TD) al-
gorithm (Ziegler et al., 2005) and improves its accu-
racy and serendipity for the insignificant price of di-
versity.
Our serendipity-oriented algorithm outperforms
the state-of-the-art serendipity-oriented algorithms in
terms of serendipity and diversity, and underperforms
them in terms of accuracy.
Unlike the traditional serendipity metric, the
serendipity metric we employed in this study captures
each component of serendipity. The choice of this
metric is supported by qualitative analysis.
In our future research, we intend to further inves-
tigate serendipity-oriented algorithms. We will also
involve real users to validate our results.
ACKNOWLEDGEMENTS
The research at the University of Jyv
¨
askyl
¨
a was per-
formed in the MineSocMed project, partially sup-
ported by the Academy of Finland, grant #268078.
REFERENCES
Adamopoulos, P. and Tuzhilin, A. (2014). On unexpected-
ness in recommender systems: Or how to better ex-
pect the unexpected. ACM Transactions on Intelligent
Systems and Technology, 5(4):1–32.
Amatriain, X. and Basilico, J. (2015). Recommender sys-
tems in industry: A netflix case study. In Recom-
mender Systems Handbook, pages 385–419. Springer.
Castells, P., Hurley, N. J., and Vargas, S. (2015). Novelty
and diversity in recommender systems. In Recom-
mender Systems Handbook, pages 881–918. Springer.
Celma Herrada,
`
O. (2009). Music recommendation and dis-
covery in the long tail. PhD thesis, Universitat Pom-
peu Fabra.
Ekstrand, M. D., Ludwig, M., Konstan, J. A., and Riedl,
J. T. (2011). Rethinking the recommender research
ecosystem: Reproducibility, openness, and lenskit.
In Proceedings of the 5th ACM Conference on Rec-
ommender Systems, pages 133–140, New York, NY,
USA. ACM.
Harper, F. M. and Konstan, J. A. (2015). The movielens
datasets: History and context. ACM Transactions on
Interactive Intelligent Systems, 5(4):19:1–19:19.
Iaquinta, L., Semeraro, G., de Gemmis, M., Lops, P., and
Molino, P. (2010). Can a recommender system induce
serendipitous encounters? InTech.
J
¨
arvelin, K. and Kek
¨
al
¨
ainen, J. (2000). Ir evaluation meth-
ods for retrieving highly relevant documents. In Pro-
ceedings of the 23rd Annual International ACM SIGIR
Conference on Research and Development in Infor-
mation Retrieval, pages 41–48, New York, NY, USA.
ACM.
Kaminskas, M. and Bridge, D. (2014). Measuring surprise
in recommender systems. In Proceedings of the Work-
shop on Recommender Systems Evaluation: Dimen-
sions and Design (Workshop Programme of the 8th
ACM Conference on Recommender Systems).
Kotkov, D., Veijalainen, J., and Wang, S. (2016a). Chal-
lenges of serendipity in recommender systems. In
Proceedings of the 12th International conference on
web information systems and technologies., volume 2,
pages 251–256. SCITEPRESS.
Kotkov, D., Wang, S., and Veijalainen, J. (2016b). A survey
of serendipity in recommender systems. Knowledge-
Based Systems, 111:180–192.
Lu, Q., Chen, T., Zhang, W., Yang, D., and Yu, Y. Serendip-
itous personalized ranking for top-n recommendation.
In Proceedings of the The IEEE/WIC/ACM Interna-
tional Joint Conferences on Web Intelligence and In-
telligent Agent Technology, volume 1.
Maksai, A., Garcin, F., and Faltings, B. (2015). Predict-
ing online performance of news recommender sys-
tems through richer evaluation metrics. In Proceed-
ings of the 9th ACM Conference on Recommender
Systems, pages 179–186, New York, NY, USA. ACM.
Ricci, F., Rokach, L., and Shapira, B. (2011). Recommender
Systems Handbook, chapter Introduction to Recom-
mender Systems Handbook, pages 1–35. Springer US.
Tacchini, E. (2012). Serendipitous mentorship in music rec-
ommender systems. PhD thesis.
Zhang, Y. C., S
´
eaghdha, D. O., Quercia, D., and Jambor, T.
(2012). Auralist: Introducing serendipity into music
recommendation. In Proceedings of the 5th ACM In-
ternational Conference on Web Search and Data Min-
ing, pages 13–22, New York, NY, USA. ACM.
Zheng, Q., Chan, C.-K., and Ip, H. H. (2015). An
unexpectedness-augmented utility model for making
serendipitous recommendation. In Advances in Data
Mining: Applications and Theoretical Aspects, vol-
ume 9165, pages 216–230. Springer International
Publishing.
Ziegler, C.-N., McNee, S. M., Konstan, J. A., and Lausen,
G. (2005). Improving recommendation lists through
topic diversification. In Proceedings of the 14th Inter-
national Conference on World Wide Web, pages 22–
32, New York, NY, USA. ACM.
WEBIST 2017 - 13th International Conference on Web Information Systems and Technologies
40