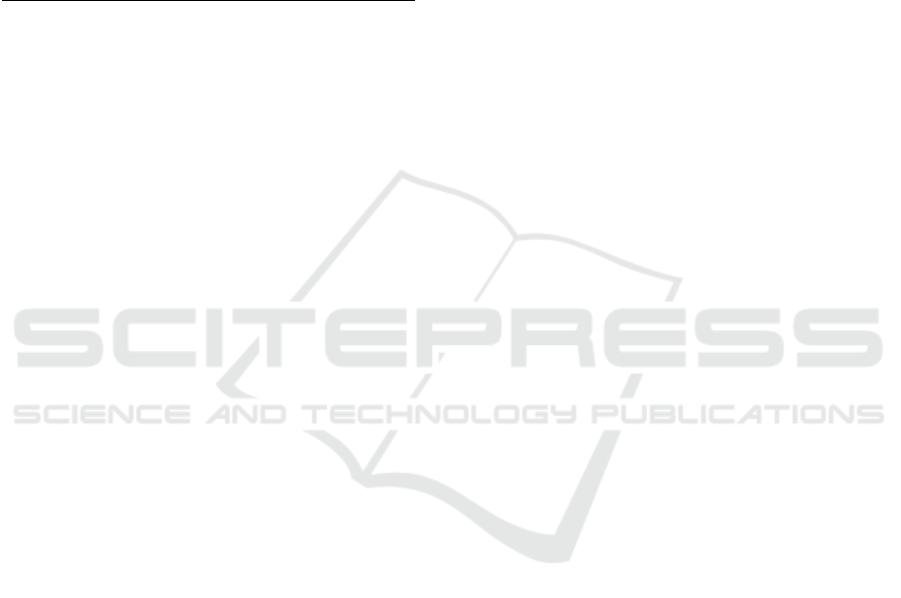
in Section III will be
max z =
M
∑
s=1
"
ω
s
K
∑
k=1
c
s
k
x
s
k
− f (d
s
) − g(ω
s
)
#
, (16)
where f (d
s
) is the cost of delay at sensor node s and
g(ω
s
) is the cost of power consumption at node s.
We will also have Eq(9) and Eq(10) as additional
constraints for the optimization problem, in addition
to stability sets of equations.
SUGGESTED IDEA FOR COLLABORATION:
This will be a new and a bit more complex class of
problems. If we decide to include the placement
problem with this then the whole model becomes
very challenging. The question of how to manage
the problem should be of interest to OR analysts
who traditionally have the expertise to handle
them.
We suggest more close collaborations between OR
analysts and Communication network researchers.
5 CONCLUSIONS & THE
POSITION
We start by discussing the first example of cognitive
radio networks. Given the information about channel
capacity, which is usually stochastic, for SUs there are
several research results for allocating that resource to
the SUs using optimization tools. However, the allo-
cations provide the service capacity to the users and
hence a very good queueing model is needed to ob-
tain the performance analysis, which itself will now
feed back into the optimization tools. Hence what we
need is a combined queueing and optimization model
in order to efficiently model these systems.
Next we consider the wireless sensor networks,
we see that the optimal placement of the sensor nodes
determine network life, its reliability and routing
which affects latency. The placements, routing and
sleep/awake mode determines the queueing delays at
the nodes which need to be included in the optimiza-
tion component of the placements, etc. Hence for the
WSN, combined optimization and queueing models
are essential in order to have a well design WSN.
Finally, keeping in mind that an effective opera-
tion of IoT depends on accurately gathering informa-
tion and passing it to the right destination within a
very short time it is imperative that a combination of
optimization and queueing models are needed for the
planning.
In summary OR analysts and communication
modelling researchers need to try and work very
closely together in order to come up with efficient
tools for analyzing modern day communication sys-
tems. That is the position that we are taking in this
paper.
ACKNOWLEDGEMENT
The authors would like to thank the Advanced Sensor
Networks (ASN) South African Research Chairs Ini-
tiative (SARChI) for their financial support in making
this work possible. Thanks to Babatunde Awoyemi
for assisting with drawing the diagrams and to Dr.
Haitham AbuGhazaleh for assisting in recovering the
Latex file.
REFERENCES
Mitola, J. and Maguire, Jr., G. Q.(1999), Cognitive radio:
Making software radio more personal, IEEE Pers.
Communications, vol. 6, No. 4, Aug. 1999, 13-18.
Haykin, S. (2005), Cognitive radio: Brain-empowered
wireless communications, IEEE Journal of Selected
Areas of Communications, vol. 23, No. 2, Feb. 2005.
Jiao, L. Li, F.Y. and Pla, V. (2012), Modelling and per-
formance analysis of channel assembling in multi-
channel cognitive radio networks with spectrum adap-
tation, IEEE Trans. Vehicular Technology, 61 (6),
2686-2697.
Jiao, L. Li, F.Y. and Pla, V. (2011), Dynamic channel ag-
gregation strategies in cognitive radio networks with
spectrum adaptation, IEEE Globecom 2011, 1-6.
Alfa, A. S., Maharaj, B. T., Lall, S., and Pal, S. (2016),
Mixed-Integer programming based techniques for re-
source allocation in underlay cognitive radio net-
works: A survey, Journal of Communications and
Networks, vol. 18, No. 5, October 2016, 744-761.
Peter Day’s World of Business, BBC World Service, OC-
TOBER 4, 2016.
Beynon, V. and Alfa, A. S. (2015), An improved algo-
rithm for finding the maximum number of set covers
for wireless sensor networks, Africon, 2015.
Cardei, M., Wu, J., Lu, M. and Pervaiz, M. O. (2005), Max-
imum network lifetime in wireless sensor networks
with adjustable sensing ranges, IEEE International
Conference on Wireless and Mobile Computing, Net-
working and Communications, WiMob 2005 vol. 3,
pp. 438-445, 2005.
Kabiri, C., Zepernick, H. and Tran, H. (2014), On
Power Consumption of Wireless Sensor Nodes with
Min(N,T) Policy in Spectrum Sharing Systems, Pro-
ceedings IEEE Vehicular Technology Conference,
Spring 2014, 1-5.
ICORES 2017 - 6th International Conference on Operations Research and Enterprise Systems
438