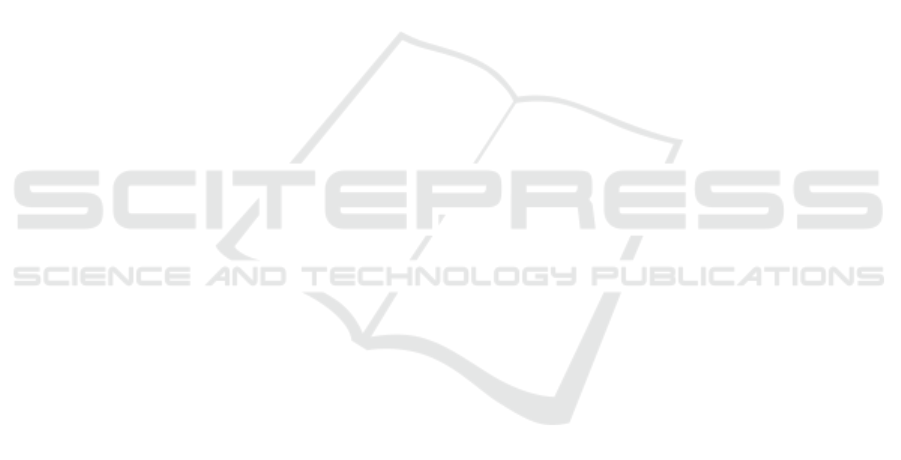
days. One more collection was performed later that
lasted for nearly 1 month and occupies 5 TB of disk
space for the further research. We took a look at
overall state of the gathered social graph by calcula-
ting weighted mean, standard weighted deviation and
found suspicious outlying users. Than, for the filte-
red ∼11.000 users that started their activity from 1
st
snapshot to 2
nd
we calculated social graph parameters
(degree, reciprocated ties and clustering) for the ego
networks that they form with their friends and friends
of friends. The analysis of suspicious users allowed
us to reveal fake profiles and even friend farms, that
we are going to study in more details in future rese-
arch. Hence, we accept the stated hypothesis that
fake profiles are more likely to be found among those
users that show abnormal behaviour in growth of so-
cial graph metrics. The contribution of this paper is
in the descriptive analysis of vk.com users’ longitudi-
nal data collection, accepting the stated earlier hypot-
hesis and revealing “friend farms”.
The further research is aimed on consecutive im-
mersion in friend farm groups. We will focus on users
who are active in this groups and analyze their actions
through time to understand their behavioural strategy
of gaining new friends. Then we would be able to
answer the question whether the friend farms are an
efficient instrument or not to make a profile look less
suspicious for subsequent implementation of advan-
ced persistent threats.
We have also identified number of websites which
sell fake social media accounts (including vk.com and
other sites). One of the further research directions is
to purchase several accounts as a ground truth about
fake profiles and analyze their behaviour (highly li-
kely, they would be in our dataset already), compare
their characteristics with legitimate accounts.
REFERENCES
Adikari, S. and Dutta, K. (2014). IDENTIFYING FAKE
PROFILES IN LINKEDIN. PACIS 2014 Proceedings.
Beutel, A., Xu, W., Guruswami, V., Palow, C., and Falout-
sos, C. (2013). CopyCatch: Stopping Group Attacks
by Spotting Lockstep Behavior in Social Networks. In
Proceedings of the 22Nd International Conference on
World Wide Web, WWW ’13, pages 119–130, New
York, NY, USA. ACM.
Boshmaf, Y., Logothetis, D., Siganos, G., Ler
´
ıa, J., Lo-
renzo, J., Ripeanu, M., Beznosov, K., and Halawa, H.
(2016).
´
Integro: Leveraging victim prediction for ro-
bust fake account detection in large scale OSNs. Com-
puters & Security, 61:142–168.
Cheng, Y. (1995). Mean Shift, Mode Seeking, and Clus-
tering. IEEE Trans. Pattern Anal. Mach. Intell.,
17(8):790–799.
Chu, Z., Gianvecchio, S., Wang, H., and Jajodia, S. (2010).
Who is Tweeting on Twitter: Human, Bot, or Cyborg?
In Proceedings of the 26th Annual Computer Security
Applications Conference, ACSAC ’10, pages 21–30,
New York, NY, USA. ACM.
Conti, M., Poovendran, R., and Secchiero, M. (2012). Fa-
keBook: Detecting Fake Profiles in On-Line Social
Networks. In ResearchGate, pages 1071–1078.
Dean, J. and Ghemawat, S. (2008). MapReduce: Simplified
Data Processing on Large Clusters. Commun. ACM,
51(1):107–113.
Facebook, i. (2014). Facebook annual report, FB-
12.31.2014-10k.
Freeman, L. C. (1982). Centered graphs and the struc-
ture of ego networks. Mathematical Social Sciences,
3(3):291–304.
Gani, A. (2015). Amazon sues 1,000 ’fake reviewers’. The
Guardian.
Hooi, B., Song, H. A., Beutel, A., Shah, N., Shin, K., and
Faloutsos, C. (2016). FRAUDAR: Bounding Graph
Fraud in the Face of Camouflage. In Proceedings of
the 22Nd ACM SIGKDD International Conference on
Knowledge Discovery and Data Mining, KDD ’16,
pages 895–904, New York, NY, USA. ACM.
Ikram, M., Onwuzurike, L., Farooqi, S., De Cristofaro, E.,
Friedman, A., Jourjon, G., Kaafar, M. A., and Shafiq,
M. Z. (2015). Combating Fraud in Online Social
Networks: Detecting Stealthy Facebook Like Farms.
arXiv:1506.00506 [cs]. arXiv: 1506.00506.
Jiang, M., Cui, P., Beutel, A., Faloutsos, C., and Yang, S.
(2016). Catching Synchronized Behaviors in Large
Networks: A Graph Mining Approach. ACM Trans.
Knowl. Discov. Data, 10(4):35:1–35:27.
Stringhini, G., Kruegel, C., and Vigna, G. (2010). Detecting
Spammers on Social Networks. In Proceedings of the
26th Annual Computer Security Applications Confe-
rence, ACSAC ’10, pages 1–9, New York, NY, USA.
ACM.
Ugander, J., Karrer, B., Backstrom, L., and Marlow, C.
(2011). The Anatomy of the Facebook Social Graph.
arXiv:1111.4503 [physics]. arXiv: 1111.4503.
WEBIST 2017 - 13th International Conference on Web Information Systems and Technologies
58