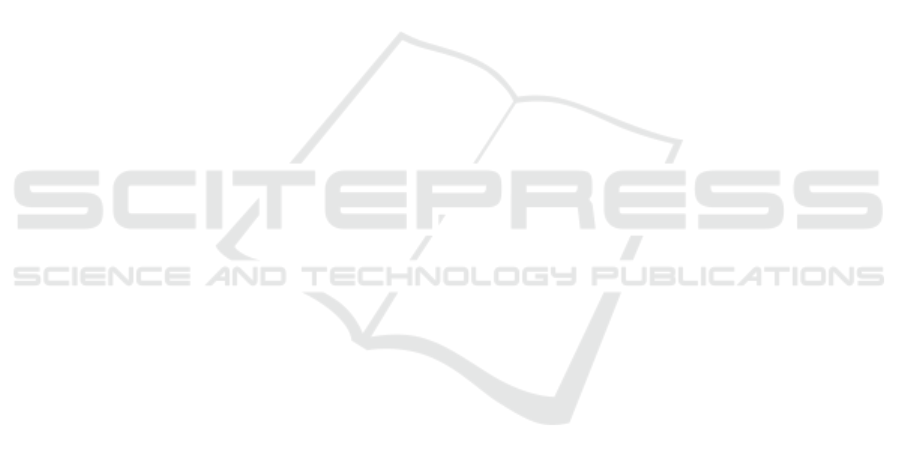
set of fourteen features seven were selected. A se-
quential forward search (SFS) wrapper-based selec-
tion algorithm was also used and its results validated
the soundness of the previously selected features.
The Controlled On/Off Loads Library (COOLL)
was used for the classification. A comparison be-
tween the appliance identification and clustering re-
sults using the turn-on transient features was con-
ducted. The results indicate that the amplitude-based
features A
i
, i = 1, . . . , 5 are the most relevant for appli-
ance identification whereas the envelope-based fea-
tures A
0
and b
3
are the most relevant for appliance
clustering.
Future work may investigate further the robust-
ness of the obtained results by testing the classifica-
tion on other datasets with bigger sizes than COOLL
and containing other families of appliance types (TV,
washing machines, refrigerator, etc.). Other problems
like model selection (parameters d and n) for the turn-
on transient current model may also be addressed.
ACKNOWLEDGEMENTS
This study was supported by the R
´
egion Centre-Val
de Loire (France) as part of the project MDE–MAC3
(Contract n
◦
2012 00073640).
REFERENCES
Barsim, K. S. and Yang, B. (2015). Toward a semi-
supervised non-intrusive load monitoring system for
event-based energy disaggregation. In 2015 IEEE
Global Conference on Signal and Information Pro-
cessing (GlobalSIP), pages 58–62.
Bonfigli, R., Squartini, S., Fagiani, M., and Piazza, F.
(2015). Unsupervised algorithms for non-intrusive
load monitoring: An up-to-date overview. In Environ-
ment and Electrical Engineering (EEEIC), 2015 IEEE
15th International Conference on, pages 1175–1180.
Coleman, T. F. and Li, Y. (1994). On the convergence of
interior-reflective newton methods for nonlinear min-
imization subject to bounds. Mathematical Program-
ming, 67(1):189–224.
Darby, S. (2010). Smart metering: what potential for house-
holder engagement? Building Research & Informa-
tion, 38(5):442–457.
Fischer, C. (2008). Feedback on household electricity con-
sumption: a tool for saving energy? Energy efficiency,
1(1):79–104.
Gillis, J. M. and Morsi, W. G. (2016). Non-intrusive load
monitoring using semi-supervised machine learning
and wavelet design. IEEE Transactions on Smart
Grid, PP(99):1–8.
Goncalves, H., Ocneanu, A., Berges, M., and Fan, R.
(2011). Unsupervised disaggregation of appliances
using aggregated consumption data. In The 1st KDD
Workshop on Data Mining Applications in Sustain-
ability (SustKDD).
Hacine-Gharbi, A., Petit, M., Ravier, P., and N
´
emo, F.
(2015). Prosody based automatic classification of the
uses of french ouias convinced or unconvinced uses.
In International Conference on Pattern Recognition
Applications and Methods (ICPRAM), number ISBN
978-989-758-077-2, pages 349–354.
Hancke, G. P., Hancke Jr, G. P., et al. (2012). The role of
advanced sensing in smart cities. Sensors, 13(1):393–
425.
Hart, G. W. (1985). Prototype nonintrusive appliance load
monitor. In MIT Energy Laboratory Technical Report,
and Electric Power Research Institute Technical Re-
port.
Hart, G. W. (1989). Residential energy monitoring and
computerized surveillance via utility power flows.
Technology and Society Magazine, IEEE, 8(2):12–16.
Hart, G. W. (1992). Nonintrusive appliance load monitor-
ing. Proceedings of the IEEE, 80(12):1870–1891.
Kohavi, R. and John, G. H. (1997). Wrappers for feature
subset selection. Artificial intelligence, 97(1):273–
324.
Nait Meziane, M., Picon, T., Ravier, P., Lamarque, G.,
Le Bunetel, J.-C., and Raingeaud, Y. (2016). A
measurement system for creating datasets of on/off-
controlled electrical loads. In Conference on Envi-
ronment and Electrical Engineering (EEEIC), Pro-
ceedings of the 16th IEEE International, pages 2579–
2583.
Nait Meziane, M., Ravier, P., Lamarque, G., Abed-Meraim,
K., Le Bunetel, J.-C., and Raingeaud, Y. (2015). Mod-
eling and estimation of transient current signals. In
Signal Processing Conference (EUSIPCO), 2015 Pro-
ceedings of the 23rd European, pages 2005–2009.
Parson, O. (2016). Overview of the nilm field.
http://blog.oliverparson.co.uk/2015/03/overview-
of-nilm-field.html.
Picon, T., Nait Meziane, M., Ravier, P., Lamarque, G., Nov-
ello, C., Le Bunetel, J.-C., and Raingeaud, Y. (2016).
COOLL: Controlled on/off loads library, a public
dataset of high-sampled electrical signals for appli-
ance identification. arXiv preprint arXiv:1611.05803.
Zeifman, M. and Roth, K. (2011). Nonintrusive appli-
ance load monitoring: Review and outlook. Consumer
Electronics, IEEE Transactions on, 57(1):76–84.
Zoha, A., Gluhak, A., Imran, M. A., and Rajasegarar, S.
(2012). Non-intrusive load monitoring approaches
for disaggregated energy sensing: a survey. Sensors,
12(12):16838–16866.
ICPRAM 2017 - 6th International Conference on Pattern Recognition Applications and Methods
654