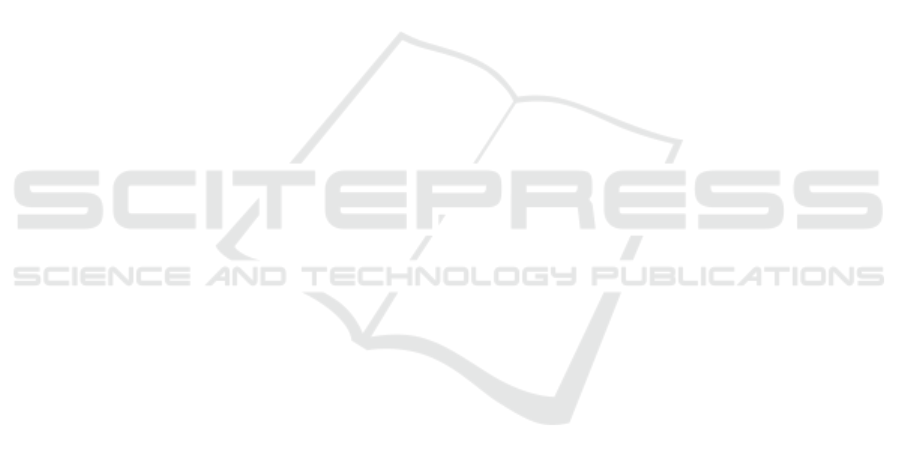
than the sky imagers. At the same time, the
prediction can be performed by using commercially
available ANN software. Software specifically
developed for solar radiation prediction is not
needed. A general use personal computer can be
used for the prediction since the required
computational efforts are limited. Because the
proposed methodologies do not require special
equipment, nor software, global solar radiation
prediction is possible for much less cost compared to
the existing methodologies.
The authors are currently improving the accuracy
of prediction by optimizing the network
configurations and their parameters. When an ANN
has relatively large number of inputs, its prediction
accuracy tends to be degraded. Having hundreds of
inputs, the prediction accuracy may not be improved
even if the number of axes is increased further.
4 CONCLUSIONS
Two different methodologies to predict global solar
radiation in 1 to 5 minutes in advance using sky
images are proposed. Image information extracted
from a total of maximum 40 points placed in one
image, combined with global solar radiation values
measured simultaneously with the sky images photo
taking, are used as the inputs for ANNs to predict
global solar radiation values. The global solar
radiation predicted by the proposed methodologies
captures the trends of the measured data well even
when there are sudden changes. When the number of
sampling points is increased the prediction accuracy
tends to be improved but the difference is rather
small. The proposed 2-step method (6 and 8-axis)
gives more accurate results than 1-step method but
the difference is not large. Cloud movement
direction data, which requires manual measurement,
is needed for 2-step method, while 1-step method
does not need it.
This methodology is focused on global solar
radiation prediction in 1 to 5 minutes in advance
because it is assumed that 1 to 5 minutes is enough
for an electrical grid to prepare for output changes
from a solar electricity generation system. The
number of sampling points in the sky images, the
sampling point interval, and the time interval to take
the sky images are optimized for the 1 to 5 minutes
prediction. It is expected that this methodology is
applicable for predictions longer than 5 minutes
when the data sampling parameters are optimized.
These proposed methodologies have an
advantage of requiring much less computational
resources and efforts compared to the existing sky
image-based prediction methodologies. These
methodologies do not need complete meteorological
data and suited to locations with no weather
measurement systems.
ACKNOWLEDGEMENTS
The authors would like to express their appreciation
to Takasago Thermal Engineering Co., Ltd. and
Universiti Teknologi Malaysia for supporting this
research through Takasago Research Grant Vot No.
4B211. The authors also wish to thank Wind
Engineering for (Urban, Artificial, Man-made)
Environment Lab (Dr. Sheikh Ahmad Zaki) in
Malaysia-Japan International Institute of
Technology for providing the global solar radiation
data.
REFERENCES
Alonso-Montesinos, J., Batlles, F.J., Portillo, C., 2015.
Solar irradiance forecasting at one-minute intervals for
different sky conditions using sky camera images.
Energy Convers. Manag. 105, 1166–1177.
Chow, C.W., Urquhart, B., Lave, M., Dominguez, A.,
Kleissl, J., Shields, J., Washom, B., 2011. Intra-hour
forecasting with a total sky imager at the UC San
Diego solar energy testbed. Sol. Energy 85, 2881–
2893.
Chu, Y., Li, M., Pedro, H.T.C., Coimbra, C.F.M., 2015.
Real-time prediction intervals for intra-hour DNI
forecasts. Renew. Energy 83, 234–244.
Davis, G.B., Griggs, D.J., Sullivan, G.D., 1991. Automatic
Estimation of Cloud Amount Using Computer Vision.
J. Atmos. Ocean. Technol.
Hasni, A., Sehli, A., Draoui, B., Bassou, A., Amieur, B.,
2012. Estimating global solar radiation using artificial
neural network and climate data in the south-western
region of Algeria. Energy Procedia 18, 531–537.
Marquez, R., Coimbra, C.F.M., 2013. Intra-hour DNI
forecasting based on cloud tracking image analysis.
Sol. Energy 91, 327–336.
Mellit, A., Eleuch, H., Benghanem, M., Elaoun, C., Pavan,
A.M., 2010. An adaptive model for predicting of
global, direct and diffuse hourly solar irradiance.
Energy Convers. Manag. 51, 771–782.
Pedro, H.T.C., Coimbra, C.F.M., 2015. Nearest-neighbor
methodology for prediction of intra-hour global
horizontal and direct normal irradiances. Renew.
Energy 80, 770–782.
Sabburg, J., Wong, J., 1999. Evaluation of a ground-based
sky camera system for use in surface irradiance
measurement. J. Atmos. Ocean. Technol. 16, 752–759.
Global Solar Radiation Prediction Methodology using Artificial Neural Networks for Photovoltaic Power Generation Systems
21