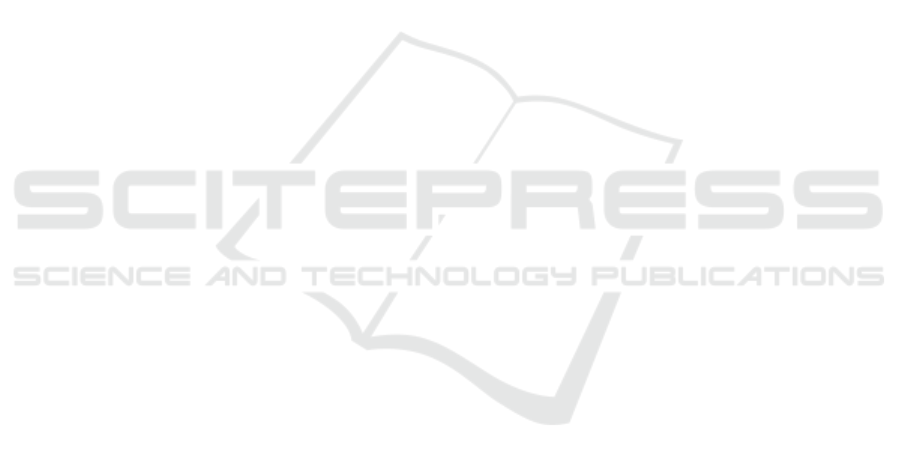
using features of RQA. This indicates some classi-
fiers are more appropriate to some type of features
than others. The authors did not find works with si-
milar objectives, but in the task of identifying types
of corrosion, as in (Jian et al., 2013) and (Hou et al.,
2016), that also use ECN signal, the accuracy is in the
range of 90% to 100%.
5 CONCLUSIONS
This paper presented an approach to identify some ty-
pes of reagents on metal surface through electroche-
mical noise signals using wavelet transform and re-
currence quantification analysis. Comparing the two
techniques, we noticed that both had a similar perfor-
mance, but wavelet transform was able to provide a
slightly higher average accuracy. For the classifiers
evaluated, we noted that MLP achieved an average
accuracy above 95% to perform the task. In relation
to the classification stage, the feature vector obtained
from the RQA is smaller, and requires less processing
capacity.
Therefore, the results of this study highlight the
importance of using wavelet transform and RQA for
electrochemical noise analysis. In future work, we in-
tend to analyze the combination of these methods, ot-
her algorithms and other features in order to improve
the results.
ACKNOWLEDGEMENTS
Lorraine Marques Alves would like to thank CAPES
for the scholarship granted.
REFERENCES
Aballe, A., Bethencourt, M., Botana, F., and Marcos, M.
(1999). Using wavelets transform in the analysis
of electrochemical noise data. Electrochimica Acta,
44(26):4805–4816.
Al-Mazeedi, H. and Cottis, R. (2004). A practical evalu-
ation of electrochemical noise parameters as indica-
tors of corrosion type. Electrochimica Acta, 49(17–
18):2787–2793.
Allegri, T. (2012). Handling and Management of Hazar-
dous Materials and Waste. Springer Science & Busi-
ness Media.
Bergelson, V. (2000). The multifarious poincaré recurrence
theorem. Descriptive set theory and dynamical sys-
tems, 1:31–57.
Bertocci, U., Gabrielli, C., Huet, F., and Keddan, M. (1997).
Noise resistance applied to corrosion measurements.
Electrochemical Society Journal, 144:31–37.
Cottis, R. A., Homborg, A., and Mol, J. (2015). The rela-
tionship between spectral and wavelet techniques for
noise analysis. Electrochimica Acta, pages 277–287.
Duda, R., Hart, D. G., and Stork, P. (2000). Pattern Recog-
nition. Wiley Interscience, New York, 1nd edition.
Dutra, L. (1999). Feature extraction and selection for ers-
1/2 insar classification. International Journal of Re-
mote Sensing, pages 993–1016.
Fofano, S. and Jambo, H. (2007). Corrosion: fundamentals,
monitoration and control. Ciencia Moderna, Brazil,
1nd edition.
Garcia-Ochoa, E. and Corvo, F. (2015). Using recurrence
plot to study the dynamics of reinforcement steel cor-
rosion. Protection of Metals and Physical Chemistry
of Surfaces, 51(4):716–724.
Hou, Y., Aldrich, C., Lepkova, K., Suarez, L., and Kinsella,
B. (2016). Monitoring of carbon steel corrosion by use
of electrochemical noise and recurrence quantification
analysis. Corrosion Science, pages 63–72.
Javaherdashti, R., Nwaoha, C., and Tan, H. (2013). Cor-
rosion and Materials in the Oil and Gas Industries.
CRC Press.
Jian, L., Weikang, K., Jiangbo, S., Ke, W., Weikui, W.,
Z.Weipu, and Zhoumo, Z. (2013). Determination of
corrosion types from electrochemical noise by artifi-
cial neural networks. international Journal of Elec-
trochemical Science, 8:2365–2377.
Koch, G., Varney, J., Thompson, N., Moghissi, O., Gould,
M., and Payer, J. (2016). International measures of
prevention, application and economics of corrosion
technologies study (impact). Technical report.
Marquardt, D. W. (1963). An algorithm for least-squares
estimation of nonlinear parameters. Journal of
the Society for Industrial and Applied Mathematics,
11(2):431–441.
Marwan, N., Romano, M., Thiel, M., and Kurths, J. (2007).
Recurrence plots for the analysis of complex systems.
Physics reports, 438:237–329.
Masters, T. (1995). Advanced algorithms for Neural Net-
works: A C++ Sourcebook. John Wiley and Sonsl,
New York, 1nd edition.
Montalban, L., Henttu, P., and Piche, R. (2007). Recur-
rence quantification analysis of electrochemical noise
data during pit development. International Journal of
Bifurcation and Chaos, 17:3725–3728.
Moshrefi, R., Mahjani, M., and Jafarian, M. (2014). Appli-
cation of wavelet entropy in analysis of electrochemi-
cal noise for corrosion type identification. Electroche-
mistry Communications, 48:49–51.
Quinlan, J. (1988). Decision trees and multivalued attribu-
tes. Oxford University Press, New York, 11nd edition.
Theodoridis, S. and Koutroumbas, K. (2008). Pattern Re-
cognition. Elsevier, San Diego, 4nd edition.
Identification of Corrosive Substances through Electrochemical Noise using Wavelet and Recurrence Quantification Analysis
723