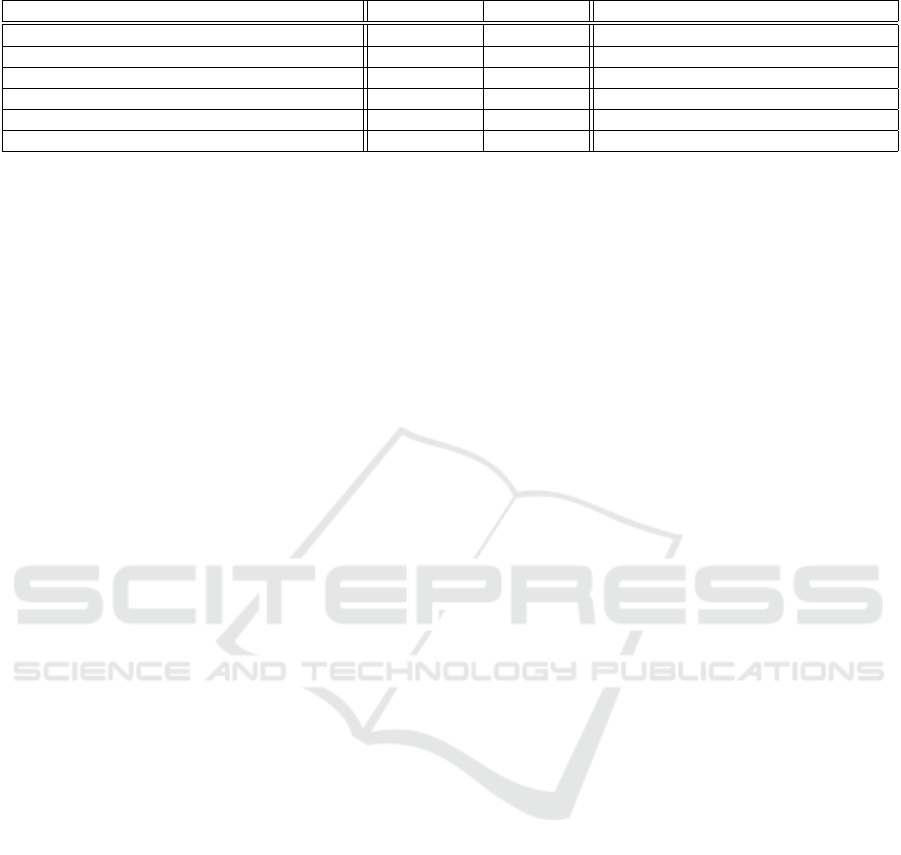
Table 2: Average transaction-level performance of the proposed and state-of-the-art FR systems on videos of the COX-S2V.
FR Systems pAUC(20%) AUPR Complexity (number of dot products)
VSkNN (Pagano et al., 2014) 56.80±4.02 26.68±3.58 671,744
SVDL (Yang et al., 2013) 69.93±5.67 44.09±6.29 810,000
Ensemble of TMs (Bashbaghi et al., 2014) 84.00±0.86 73.36±9.82 1,387,200
ESRC-DA (Nourbakhsh et al., 2016) 99.00±0.13 63.21±4.56 432,224,100
Ensemble of e-SVMs (Bashbaghi et al., 2016) 99.02±0.15 88.03±0.85 2,327,552
Proposed system w. DS 99.02±0.23 88.40±0.96 504,720
the competence criteria. Simulation results obtained
using videos of the COX-S2V dataset confirm that the
proposed system is computationally efficient and out-
performs the state-of-the-art systems even when the
data is limited and imbalanced.
ACKNOWLEDGMENT
This work was supported by the Fonds de Recherche
du Québec - Nature et Technologies.
REFERENCES
Ahonen, T., Rahtu, E., Ojansivu, V., and Heikkila, J. (2008).
Recognition of blurred faces using local phase quanti-
zation. In ICPR, pages 1–4.
Barr, J. R., Bowyer, K. W., Flynn, P. J., and Biswas, S.
(2012). Face recognition from video: A review. IJ-
PRAI, 26(05).
Bashbaghi, S., Granger, E., Sabourin, R., and Bilodeau, G.-
A. (2014). Watch-list screening using ensembles ba-
sed on multiple face representations. In ICPR, pages
4489–4494.
Bashbaghi, S., Granger, E., Sabourin, R., and Bilodeau, G.-
A. (2015). Ensembles of exemplar-svms for video
face recognition from a single sample per person. In
AVSS, pages 1–6.
Bashbaghi, S., Granger, E., Sabourin, R., and Bilodeau, G.-
A. (2016). Robust watch-list screening using dynamic
ensembles of svms based on multiple face representa-
tions. Machine Vision and Applications.
Britto, A. S., Sabourin, R., and Oliveira, L. E. (2014). Dyn-
amic selection of classifiers - a comprehensive review.
Pattern Recognition, 47(11):3665 – 3680.
Chang, C.-C. and Lin, C.-J. (2011). Libsvm: A library for
support vector machines. ACM TIST, 2(3):1–27.
Chen, C., Dantcheva, A., and Ross, A. (2015). An ensem-
ble of patch-based subspaces for makeup-robust face
recognition. Information Fusion, pages 1–13.
De la Torre Gomerra, M., Granger, E., Radtke, P. V., Sa-
bourin, R., and Gorodnichy, D. O. (2015). Partially-
supervised learning from facial trajectories for face re-
cognition in video surveillance. Information Fusion,
24:31–53.
De-la Torre Gomerra, M., Granger, E., Sabourin, R., and
Gorodnichy, D. O. (2015). Adaptive skew-sensitive
ensembles for face recognition in video surveillance.
Pattern Recognition, 48(11):3385 – 3406.
Deniz, O., Bueno, G., Salido, J., and la Torre, F. D. (2011).
Face recognition using histograms of oriented gra-
dients. Pattern Recognition Letters, 32(12):1598 –
1603.
Dewan, M. A. A., Granger, E., Marcialis, G.-L., Sabourin,
R., and Roli, F. (2016). Adaptive appearance model
tracking for still-to-video face recognition. Pattern
Recognition, 49:129 – 151.
Huang, Z., Shan, S., Wang, R., Zhang, H., Lao, S., Kuerban,
A., and Chen, X. (2015). A benchmark and compara-
tive study of video-based face recognition on cox face
database. IP, IEEE Trans on, 24(12):5967–5981.
Kamgar-Parsi, B., Lawson, W., and Kamgar-Parsi, B.
(2011). Toward development of a face recognition sy-
stem for watchlist surveillance. IEEE Trans on PAMI,
33(10):1925–1937.
Malisiewicz, T., Gupta, A., and Efros, A. (2011). Ensemble
of exemplar-svms for object detection and beyond. In
ICCV, pages 89–96.
Mokhayeri, F., Granger, E., and Bilodeau, G.-A. (2015).
Synthetic face generation under various operational
conditions in video surveillance. In ICIP, pages 4052–
4056.
Nourbakhsh, F., Granger, E., and Fumera, G. (2016). An
extended sparse classification framework for domain
adaptation in video surveillance. In ACCV, Workshop
on Human Identification for Surveillance.
Pagano, C., Granger, E., Sabourin, R., Marcialis, G., and
Roli, F. (2014). Adaptive ensembles for face recogni-
tion in changing video surveillance environments. In-
formation Sciences, 286:75–101.
Pan, S. J. and Yang, Q. (2010). A survey on transfer lear-
ning. KDE, IEEE Trans on, 22(10):1345–1359.
Patel, V., Gopalan, R., Li, R., and Chellappa, R. (2015). Vi-
sual domain adaptation: A survey of recent advances.
IEEE Signal Processing Magazine, 32(3):53–69.
Qiu, Q., Ni, J., and Chellappa, R. (2014). Dictionary-based
domain adaptation for the re-identification of faces. In
Person Re-Identification, Advances in Computer Vi-
sion and Pattern Recognition, pages 269–285.
Shekhar, S., Patel, V., Nguyen, H., and Chellappa, R.
(2013). Generalized domain-adaptive dictionaries. In
CVPR, pages 361–368.
Yang, M., Van Gool, L., and Zhang, L. (2013). Sparse va-
riation dictionary learning for face recognition with
a single training sample per person. In ICCV, pages
689–696.
Dynamic Selection of Exemplar-SVMs for Watch-list Screening through Domain Adaptation
745