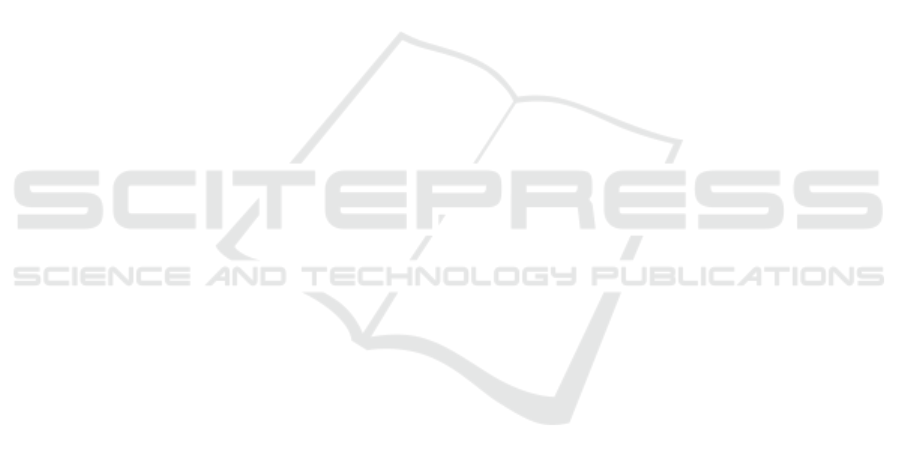
Hu, Z., Wang, Y., Tian, Y., and Huang, T. (2011). Selective
eigenbackgrounds method for background subtraction
in crowed scenes. In Image Processing (ICIP), 2011
18th IEEE International Conference on, pages 3277–
3280.
Kaewtrakulpong, P. and Bowden, R. (2002). An im-
proved adaptive background mixture model for real-
time tracking with shadow detection. In Video-based
surveillance systems, pages 135–144. Springer.
Marghes, C., Bouwmans, T., and Vasiu, R. (2012). Back-
ground modeling and foreground detection via a re-
constructive and discriminative subspace learning ap-
proach. In Image Processing, Computer Vision, and
Pattern Recognition (IPCV’12), The 2012 Interna-
tional Conference on, volume 02, pages 106–112.
Mignotte, M. (2010). A label field fusion bayesian model
and its penalized maximum rand estimator for image
segmentation. IEEE Transactions on Image Process-
ing, 19(6):1610–1624.
Nath, S. and Palaniappan, K. (2005). Adaptive robust
structure tensors for orientation estimation and im-
age segmentation. Lecture Notes in Computer Science
(ISVC), 3804:445–453.
Radolko, M., Farhadifard, F., Gutzeit, E., and von Lukas,
U. F. (2015). Real time video segmentation optimiza-
tion with a modified normalized cut. In Image and
Signal Processing and Analysis (ISPA), 2015 9th In-
ternational Symposium on, pages 31–36.
Radolko, M., Farhadifard, F., Gutzeit, E., and von Lukas,
U. F. (2016). Dataset on underwater change detection.
In OCEANS 2016 - MONTEREY, pages 1–8.
Radolko, M. and Gutzeit, E. (2015). Video segmentation via
a gaussian switch background-model and higher or-
der markov random fields. In Proceedings of the 10th
International Conference on Computer Vision Theory
and Applications Volume 1, pages 537–544.
Ridder, C., Munkelt, O., and Kirchner, H. (1995). Adap-
tive background estimation and foreground detection
using kalman-filtering. In Proceedings of Interna-
tional Conference on recent Advances in Mechatron-
ics, pages 193–199.
Schindler, K. and Wang, H. (2006). Smooth foreground-
background segmentation for video processing. In
Proceedings of the 7th Asian Conference on Computer
Vision - Volume Part II, ACCV’06, pages 581–590,
Berlin, Heidelberg. Springer-Verlag.
Shelley, A. J. and Seed, N. L. (1993). Approaches to static
background identification and removal. In Image Pro-
cessing for Transport Applications, IEE Colloquium
on, pages 6/1–6/4.
St-Charles, P. L., Bilodeau, G. A., and Bergevin, R. (2015).
Subsense: A universal change detection method with
local adaptive sensitivity. IEEE Transactions on Im-
age Processing, 24(1):359–373.
Stauffer, C. and Grimson, W. (1999). Adaptive background
mixture models for real-time tracking. In Proceedings
1999 IEEE Computer Society Conference on Com-
puter Vision and Pattern Recognition Vol. Two, pages
246–252. IEEE Computer Society Press.
Toyama, K., Krumm, J., Brumitt, B., and Meyers, B.
(1999). Wallflower: Principles and practice of back-
ground maintenance. In Seventh International Confer-
ence on Computer Vision, pages 255–261. IEEE Com-
puter Society Press.
Wang, R., Bunyak, F., Seetharaman, G., and Palaniappan,
K. (2014). Static and moving object detection using
flux tensor with split gaussian models. In 2014 IEEE
Conference on Computer Vision and Pattern Recogni-
tion Workshops, pages 420–424.
Warfield, S. K., Zou, K. H., and Wells, W. M. (2004).
Simultaneous truth and performance level estimation
(staple): An algorithm for the validation of image seg-
mentation. Ieee Transactions on Medical Imaging,
23:903–921.
Wren, C., Azarbayejani, A., Darrell, T., and Pentland, A.
(1997). Pfinder: Real-time tracking of the human
body. IEEE Transactions on Pattern Analysis and Ma-
chine Intelligence, 19:780–785.
Zivkovic, Z. (2004). Improved adaptive gaussian mixture
model for background subtraction. In Proceedings
of the Pattern Recognition, 17th International Confer-
ence on (ICPR’04) Volume 2 - Volume 02, ICPR ’04,
pages 28–31, Washington, DC, USA. IEEE Computer
Society.
Zivkovic, Z. and Heijden, F. (2006). Efficient adaptive den-
sity estimation per image pixel for the task of back-
ground subtraction. Pattern Recogn. Lett., 27(7):773–
780.
Change Detection in Crowded Underwater Scenes - Via an Extended Gaussian Switch Model Combined with a Flux Tensor
Pre-segmentation
415